User login
Automating venous thromboembolism risk calculation using electronic health record data upon hospital admission: The automated Padua Prediction Score
Hospital-acquired venous thromboembolism (VTE) continues to be a critical quality challenge for U.S. hospitals,1 and high-risk patients are often not adequately prophylaxed. Use of VTE prophylaxis (VTEP) varies as widely as 26% to 85% of patients in various studies, as does patient outcomes and care expenditures.2-6 The 9th edition of the American College of Chest Physicians (CHEST) guidelines7 recommend the Padua Prediction Score (PPS) to select individual patients who may be at high risk for venous thromboembolism (VTE) and could benefit from thromboprophylaxis. Use of the manually calculated PPS to select patients for thromboprophylaxis has been shown to help decrease 30-day and 90-day mortality associated with VTE events after hospitalization to medical services.8 However, the PPS requires time-consuming manual calculation by a provider, who may be focused on more immediate aspects of patient care and several other risk scores competing for his attention, potentially decreasing its use.
Other risk scores that use only discrete scalar data, such as vital signs and lab results to predict early recognition of sepsis, have been successfully automated and implemented within electronic health records (EHRs).9-11 Successful automation of scores requiring input of diagnoses, recent medical events, and current clinical status such as the PPS remains difficult.12 Data representing these characteristics are more prone to error, and harder to translate clearly into a single data field than discrete elements like heart rate, potentially impacting validity of the calculated result.13 To improve usage of guideline based VTE risk assessment and decrease physician burden, we developed an algorithm called Automated Padua Prediction Score (APPS) that automatically calculates the PPS using only EHR data available within prior encounters and the first 4 hours of admission, a similar timeframe to when admitting providers would be entering orders. Our goal was to assess if an automatically calculated version of the PPS, a score that depends on criteria more complex than vital signs and labs, would accurately assess risk for hospital-acquired VTE when compared to traditional manual calculation of the Padua Prediction Score by a provider.
METHODS
Site Description and Ethics
The study was conducted at University of California, San Francisco Medical Center, a 790-bed academic hospital; its Institutional Review Board approved the study and collection of data via chart review. Handling of patient information complied with the Health Insurance Portability and Accountability Act of 1996.
Patient Inclusion
Adult patients admitted to a medical or surgical service between July 1, 2012 and April 1, 2014 were included in the study if they were candidates for VTEP, defined as: length of stay (LOS) greater than 2 days, not on hospice care, not pregnant at admission, no present on admission VTE diagnosis, no known contraindications to prophylaxis (eg, gastrointestinal bleed), and were not receiving therapeutic doses of warfarin, low molecular weight heparins, heparin, or novel anticoagulants prior to admission.
Data Sources
Clinical variables were extracted from the EHR’s enterprise data warehouse (EDW) by SQL Server query (Microsoft, Redmond, Washington) and deposited in a secure database. Chart review was conducted by a trained researcher (Mr. Jacolbia) using the EHR and a standardized protocol. Findings were recorded using REDCap (REDCap Consortium, Vanderbilt University, Nashville, Tennessee). The specific ICD-9, procedure, and lab codes used to determine each criterion of APPS are available in the Appendix.
Creation of the Automated Padua Prediction Score (APPS)
We developed APPS from the original 11 criteria that comprise the Padua Prediction Score: active cancer, previous VTE (excluding superficial vein thrombosis), reduced mobility, known thrombophilic condition, recent (1 month or less) trauma and/or surgery, age 70 years or older, heart and/or respiratory failure, acute myocardial infarction and/or ischemic stroke, acute infection and/or rheumatologic disorder, body mass index (BMI) 30 or higher, and ongoing hormonal treatment.13 APPS has the same scoring methodology as PPS: criteria are weighted from 1 to 3 points and summed with a maximum score of 20, representing highest risk of VTE. To automate the score calculation from data routinely available in the EHR, APPS checks pre-selected structured data fields for specific values within laboratory results, orders, nursing flowsheets and claims. Claims data included all ICD-9 and procedure codes used for billing purposes. If any of the predetermined data elements are found, then the specific criterion is considered positive; otherwise, it is scored as negative. The creators of the PPS were consulted in the generation of these data queries to replicate the original standards for deeming a criterion positive. The automated calculation required no use of natural language processing.
Characterization of Study Population
We recorded patient demographics (age, race, gender, BMI), LOS, and rate of hospital-acquired VTE. These patients were separated into 2 cohorts determined by the VTE prophylaxis they received. The risk profile of patients who received pharmacologic prophylaxis was hypothesized to be inherently different from those who had not. To evaluate APPS within this heterogeneous cohort, patients were divided into 2 major categories: pharmacologic vs. no pharmacologic prophylaxis. If they had a completed order or medication administration record on the institution’s approved formulary for pharmacologic VTEP, they were considered to have received pharmacologic prophylaxis. If they had only a completed order for usage of mechanical prophylaxis (sequential compression devices) or no evidence of any form of VTEP, they were considered to have received no pharmacologic prophylaxis. Patients with evidence of both pharmacologic and mechanical were placed in the pharmacologic prophylaxis group. To ensure that automated designation of prophylaxis group was accurate, we reviewed 40 randomly chosen charts because prior researchers were able to achieve sensitivity and specificity greater than 90% with that sample size.14
The primary outcome of hospital-acquired VTE was defined as an ICD-9 code for VTE (specific codes are found in the Appendix) paired with a “present on admission = no” flag on that encounter’s hospital billing data, abstracted from the EDW. A previous study at this institution used the same methodology and found 212/226 (94%) of patients with a VTE ICD-9 code on claim had evidence of a hospital-acquired VTE event upon chart review.14 Chart review was also completed to ensure that the primary outcome of newly discovered hospital-acquired VTE was differentiated from chronic VTE or history of VTE. Theoretically, ICD-9 codes and other data elements treat chronic VTE, history of VTE, and hospital-acquired VTE as distinct diagnoses, but it was unclear if this was true in our dataset. For 75 randomly selected cases of presumed hospital-acquired VTE, charts were reviewed for evidence that confirmed newly found VTE during that encounter.
Validation of APPS through Comparison to Manual Calculation of the Original PPS
To compare our automated calculation to standard clinical practice, we manually calculated the PPS through chart review within the first 2 days of admission on 300 random patients, a subsample of the entire study cohort. The largest study we could find had manually calculated the PPS of 1,080 hospitalized patients with a mean PPS of 4.86 (standard deviation [SD], 2.26).15 One researcher (Mr. Jacolbia) accessed the EHR with all patient information available to physicians, including admission notes, orders, labs, flowsheets, past medical history, and all prior encounters to calculate and record the PPS. To limit potential score bias, 2 authors (Drs. Elias and Davies) assessed 30 randomly selected charts from the cohort of 300. The standardized chart review protocol mimicked a physician’s approach to determine if a patient met a criterion, such as concluding if he/she had active cancer by examining medication lists for chemotherapy, procedure notes for radiation, and recent diagnoses on problem lists. After the original PPS was manually calculated, APPS was automatically calculated for the same 300 patients. We intended to characterize similarities and differences between APPS and manual calculation prior to investigating APPS’ predictive capacity for the entire study population, because it would not be feasible to manually calculate the PPS for all 30,726 patients.
Statistical Analysis
For the 75 randomly selected cases of presumed hospital-acquired VTE, the number of cases was chosen by powering our analysis to find a difference in proportion of 20% with 90% power, α = 0.05 (two-sided). We conducted χ2 tests on the entire study cohort to determine if there were significant differences in demographics, LOS, and incidence of hospital-acquired VTE by prophylaxis received. For both the pharmacologic and the no pharmacologic prophylaxis groups, we conducted 2-sample Student t tests to determine significant differences in demographics and LOS between patients who experienced a hospital-acquired VTE and those who did not.
For the comparison of our automated calculation to standard clinical practice, we manually calculated the PPS through chart review within the first 2 days of admission on a subsample of 300 random patients. We powered our analysis to detect a difference in mean PPS from 4.86 to 4.36, enough to alter the point value, with 90% power and α = 0.05 (two-sided) and found 300 patients to be comfortably above the required sample size. We compared APPS and manual calculation in the 300-patient cohort using: 2-sample Student t tests to compare mean scores, χ2 tests to compare the frequency with which criteria were positive, and receiver operating characteristic (ROC) curves to determine capacity to predict a hospital-acquired VTE event. Pearson’s correlation was also completed to assess score agreement between APPS and manual calculation on a per-patient basis. After comparing automated calculation of APPS to manual chart review on the same 300 patients, we used APPS to calculate scores for the entire study cohort (n = 30,726). We calculated the mean of APPS by prophylaxis group and whether hospital-acquired VTE had occurred. We analyzed APPS’ ROC curve statistics by prophylaxis group to determine its overall predictive capacity in our study population. Lastly, we computed the time required to calculate APPS per patient. Statistical analyses were conducted using SPSS Statistics (IBM, Armonk, New York) and Python 2.7 (Python Software Foundation, Beaverton, Oregon); 95% confidence intervals (CI) and (SD) were reported when appropriate.
RESULTS
Among the 30,726 unique patients in our entire cohort (all patients admitted during the time period who met the study criteria), we found 6574 (21.4%) on pharmacologic (with or without mechanical) prophylaxis, 13,511 (44.0%) on mechanical only, and 10,641 (34.6%) on no prophylaxis. χ2 tests found no significant differences in demographics, LOS, or incidence of hospital-acquired VTE between the patients who received mechanical prophylaxis only and those who received no prophylaxis (Table 1). Similarly, there were no differences in these characteristics in patients receiving pharmacologic prophylaxis with or without the addition of mechanical prophylaxis. Designation of prophylaxis group by manual chart review vs. our automated process was found to agree in categorization for 39/40 (97.5%) sampled encounters. When comparing the cohort that received pharmacologic prophylaxis against the cohort that did not, there were significant differences in racial distribution, sex, BMI, and average LOS as shown in Table 1. Those who received pharmacologic prophylaxis were found to be significantly older than those who did not (62.7 years versus 53.2 years, P < 0.001), more likely to be male (50.6% vs, 42.4%, P < 0.001), more likely to have hospital-acquired VTE (2.2% vs. 0.5%, P < 0.001), and to have a shorter LOS (7.1 days vs. 9.8, P < 0.001).
Within the cohort group receiving pharmacologic prophylaxis (n = 6574), hospital-acquired VTE occurred in patients who were significantly younger (58.2 years vs. 62.8 years, P = 0.003) with a greater LOS (23.8 days vs. 6.7, P < 0.001) than those without. Within the group receiving no pharmacologic prophylaxis (n = 24,152), hospital-acquired VTE occurred in patients who were significantly older (57.1 years vs. 53.2 years, P = 0.014) with more than twice the LOS (20.2 days vs. 9.7 days, P < 0.001) compared to those without. Sixty-six of 75 (88%) randomly selected patients in which new VTE was identified by the automated electronic query had this diagnosis confirmed during manual chart review.
As shown in Table 2, automated calculation on a subsample of 300 randomly selected patients using APPS had a mean of 5.5 (SD, 2.9) while manual calculation of the original PPS on the same patients had a mean of 5.1 (SD, 2.6). There was no significant difference in mean between manual calculation and APPS (P = 0.073). There were, however, significant differences in how often individual criteria were considered present. The largest contributors to the difference in scores between APPS and manual calculation were “prior VTE” (positive, 16% vs. 8.3%, respectively) and “reduced mobility” (positive, 74.3% vs. 66%, respectively) as shown in Table 2. In the subsample, there were a total of 6 (2.0%) hospital-acquired VTE events. APPS’ automated calculation had an AUC = 0.79 (CI, 0.63-0.95) that was significant (P = 0.016) with a cutoff value of 5. Chart review’s manual calculation of the PPS had an AUC = 0.76 (CI 0.61-0.91) that was also significant (P = 0.029).
Distribution of Patient Characteristics in Cohort
Our entire cohort of 30,726 unique patients admitted during the study period included 260 (0.8%) who experienced hospital-acquired VTEs (Table 3). In patients receiving no pharmacologic prophylaxis, the average APPS was 4.0 (SD, 2.4) for those without VTE and 7.1 (SD, 2.3) for those with VTE. In patients who had received pharmacologic prophylaxis, those without hospital-acquired VTE had an average APPS of 4.9 (SD, 2.6) and those with hospital-acquired VTE averaged 7.7 (SD, 2.6). APPS’ ROC curves for “no pharmacologic prophylaxis” had an AUC = 0.81 (CI, 0.79 – 0.83) that was significant (P < 0.001) with a cutoff value of 5. There was similar performance in the pharmacologic prophylaxis group with an AUC = 0.79 (CI, 0.76 – 0.82) and cutoff value of 5, as shown in the Figure. Over the entire cohort, APPS had a sensitivity of 85.4%, specificity of 53.3%, positive predictive value (PPV) of 1.5%, and a negative predictive value (NPV) of 99.8% when using a cutoff of 5. The average APPS calculation time was 0.03 seconds per encounter. Additional information on individual criteria can be found in Table 3.
DISCUSSION
Automated calculation of APPS using EHR data from prior encounters and the first 4 hours of admission was predictive of in-hospital VTE. APPS performed as well as traditional manual score calculation of the PPS. It was able to do so with no physician input, significantly lessening the burden of calculation and potentially increasing frequency of data-driven VTE risk assessment.
While automated calculation of certain scores is becoming more common, risk calculators that require data beyond vital signs and lab results have lagged,16-19 in part because of uncertainty about 2 issues. The first is whether EHR data accurately represent the current clinical picture. The second is if a machine-interpretable algorithm to determine a clinical status (eg, “active cancer”) would be similar to a doctor’s perception of that same concept. We attempted to better understand these 2 challenges through developing APPS. Concerning accuracy, EHR data correctly represent the clinical scenario: designations of VTEP and hospital-acquired VTE were accurate in approximately 90% of reviewed cases. Regarding the second concern, when comparing APPS to manual calculation, we found significant differences (P < 0.001) in how often 8 of the 11 criteria were positive, yet no significant difference in overall score and similar predictive capacity. Manual calculation appeared more likely to find data in the index encounter or in structured data. For example, “active cancer” may be documented only in a physician’s note, easily accounted for during a physician’s calculation but missed by APPS looking only for structured data. In contrast, automated calculation found historic criteria, such as “prior VTE” or “known thrombophilic condition,” positive more often. If the patient is being admitted for a problem unrelated to blood clots, the physician may have little time or interest to look through hundreds of EHR documents to discover a 2-year-old VTE. As patients’ records become larger and denser, more historic data can become buried and forgotten. While the 2 scores differ on individual criteria, they are similarly predictive and able to bifurcate the at-risk population to those who should and should not receive pharmacologic prophylaxis.
The APPS was found to have near-equal performance in the pharmacologic vs. no pharmacologic prophylaxis cohorts. This finding agrees with a study that found no significant difference in predicting 90-day VTE when looking at 86 risk factors vs. the most significant 4, none of which related to prescribed prophylaxis.18 The original PPS had a reported sensitivity of 94.6%, specificity 62%, PPV 7.5%, and NPV 99.7% in its derivation cohort.13 We matched APPS to the ratio of sensitivity to specificity, using 5 as the cutoff value. APPS performed slightly worse with sensitivity of 85.4%, specificity 53.3%, PPV 1.5%, and NPV 99.8%. This difference may have resulted from the original PPS study’s use of 90-day follow-up to determine VTE occurrence, whereas we looked only until the end of current hospitalization, an average of 9.2 days. Furthermore, the PPS had significantly poorer performance (AUC = 0.62) than that seen in the original derivation cohort in a separate study that manually calculated the score on more than 1000 patients.15
There are important limitations to our study. It was done at a single academic institution using a dataset of VTE-associated, validated research that was well-known to the researchers.20 Another major limitation is the dependence of the algorithm on data available within the first 4 hours of admission and earlier; thus, previous encounters may frequently play an important role. Patients presenting to our health system for the first time would have significantly fewer data available at the time of calculation. Additionally, our data could not reliably tell us the total doses of pharmacologic prophylaxis that a patient received. While most patients will maintain a consistent VTEP regimen once initiated in the hospital, 2 patients with the same LOS may have received differing amounts of pharmacologic prophylaxis. This research study did not assess how much time automatic calculation of VTE risk might save providers, because we did not record the time for each manual abstraction; however, from discussion with the main abstracter, chart review and manual calculation for this study took from 2 to 14 minutes per patient, depending on the number of previous interactions with the health system. Finally, although we chose data elements that are likely to exist at most institutions using an EHR, many institutions’ EHRs do not have EDW capabilities nor programmers who can assist with an automated risk score.
The EHR interventions to assist providers in determining appropriate VTEP have been able to increase rates of VTEP and decrease VTE-associated mortality.16,21 In addition to automating the calculation of guideline-adherent risk scores, there is a need for wider adoption for clinical decision support for VTE. For this reason, we chose only structured data fields from some of the most common elements within our EHR’s data warehouse to derive APPS (Appendix 1). Our study supports the idea that automated calculation of scores requiring input of more complex data such as diagnoses, recent medical events, and current clinical status remains predictive of hospital-acquired VTE risk. Because it is calculated automatically in the background while the clinician completes his or her assessment, the APPS holds the potential to significantly reduce the burden on providers while making guideline-adherent risk assessment more readily accessible. Further research is required to determine the exact amount of time automatic calculation saves, and, more important, if the relatively high predictive capacity we observed using APPS would be reproducible across institutions and could reduce incidence of hospital-acquired VTE.
Disclosures
Dr. Auerbach was supported by NHLBI K24HL098372 during the period of this study. Dr. Khanna, who is an implementation scientist at the University of California San Francisco Center for Digital Health Innovation, is the principal inventor of CareWeb, and may benefit financially from its commercialization. The other authors report no financial conflicts of interest.
1. Galson S. The Surgeon General’s call to action to prevent deep vein thrombosis and pulmonary embolism. 2008. https://www.ncbi.nlm.nih.gov/books/NBK44178/. Accessed February 11, 2016. PubMed
2. Borch KH, Nyegaard C, Hansen JB, et al. Joint effects of obesity and body height on the risk of venous thromboembolism: the Tromsø study. Arterioscler Thromb Vasc Biol. 2011;31(6):1439-44. PubMed
3. Braekkan SK, Borch KH, Mathiesen EB, Njølstad I, Wilsgaard T, Hansen JB.. Body height and risk of venous thromboembolism: the Tromsø Study. Am J Epidemiol. 2010;171(10):1109-1115. PubMed
4. Bounameaux H, Rosendaal FR. Venous thromboembolism: why does ethnicity matter? Circulation. 2011;123(200:2189-2191. PubMed
5. Spyropoulos AC, Anderson FA Jr, Fitzgerald G, et al; IMPROVE Investigators. Predictive and associative models to identify hospitalized medical patients at risk for VTE. Chest. 2011;140(3):706-714. PubMed
6. Rothberg MB, Lindenauer PK, Lahti M, Pekow PS, Selker HP. Risk factor model to predict venous thromboembolism in hospitalized medical patients. J Hosp Med. 2011;6(4):202-209. PubMed
7. Perioperative Management of Antithrombotic Therapy: Prevention of VTE in Nonsurgical Patients: Antithrombotic Therapy and Prevention of Thrombosis, 9th ed: American College of Chest Physicians Evidence-Based Clinical Practice Guidelines. Chest. 2012;141(6):1645.
8. Subbe CP, Kruger M, Rutherford P, Gemmel L. Validation of a modified Early Warning Score in medical admissions. QJM. 2001;94(10):521-526. PubMed
9. Alvarez CA, Clark CA, Zhang S, et al. Predicting out of intensive care unit cardiopulmonary arrest or death using electronic medical record data. BMC Med Inform Decis Mak. 2013;13:28. PubMed
10. Escobar GJ, LaGuardia JC, Turk BJ, Ragins A, Kipnis P, Draper D. Early detection of impending physiologic deterioration among patients who are not in intensive care: development of predictive models using data from an automated electronic medical record. J Hosp Med. 2012;7(5):388-395. PubMed
11. Umscheid CA, Hanish A, Chittams J, Weiner MG, Hecht TE. Effectiveness of a novel and scalable clinical decision support intervention to improve venous thromboembolism prophylaxis: a quasi-experimental study. BMC Med Inform Decis Mak. 2012;12:92. PubMed
12. Tepas JJ 3rd, Rimar JM, Hsiao AL, Nussbaum MS. Automated analysis of electronic medical record data reflects the pathophysiology of operative complications. Surgery. 2013;154(4):918-924. PubMed
13. Barbar S, Noventa F, Rossetto V, et al. A risk assessment model for the identification of hospitalized medical patients at risk for venous thromboembolism: the Padua Prediction Score. J Thromb Haemost. 2010; 8(11):2450-2457. PubMed
14. Khanna R, Maynard G, Sadeghi B, et al. Incidence of hospital-acquired venous thromboembolic codes in medical patients hospitalized in academic medical centers. J Hosp Med. 2014; 9(4):221-225. PubMed
15. Vardi M, Ghanem-Zoubi NO, Zidan R, Yurin V, Bitterman H. Venous thromboembolism and the utility of the Padua Prediction Score in patients with sepsis admitted to internal medicine departments. J Thromb Haemost. 2013;11(3):467-473. PubMed
16. Samama MM, Dahl OE, Mismetti P, et al. An electronic tool for venous thromboembolism prevention in medical and surgical patients. Haematologica. 2006;91(1):64-70. PubMed
17. Mann DM, Kannry JL, Edonyabo D, et al. Rationale, design, and implementation protocol of an electronic health record integrated clinical prediction rule (iCPR) randomized trial in primary care. Implement Sci. 2011;6:109. PubMed
18. Woller SC, Stevens SM, Jones JP, et al. Derivation and validation of a simple model to identify venous thromboembolism risk in medical patients. Am J Med. 2011;124(10):947-954. PubMed
19. Huang W, Anderson FA, Spencer FA, Gallus A, Goldberg RJ. Risk-assessment models for predicting venous thromboembolism among hospitalized non-surgical patients: a systematic review. J Thromb Thrombolysis. 2013;35(1):67-80. PubMed
20. Khanna RR, Kim SB, Jenkins I, et al. Predictive value of the present-on-admission indicator for hospital-acquired venous thromboembolism. Med Care. 2015;53(4):e31-e36. PubMed
21. Kucher N, Koo S, Quiroz R, et al. Electronic alerts to prevent venous thromboembolism a
Hospital-acquired venous thromboembolism (VTE) continues to be a critical quality challenge for U.S. hospitals,1 and high-risk patients are often not adequately prophylaxed. Use of VTE prophylaxis (VTEP) varies as widely as 26% to 85% of patients in various studies, as does patient outcomes and care expenditures.2-6 The 9th edition of the American College of Chest Physicians (CHEST) guidelines7 recommend the Padua Prediction Score (PPS) to select individual patients who may be at high risk for venous thromboembolism (VTE) and could benefit from thromboprophylaxis. Use of the manually calculated PPS to select patients for thromboprophylaxis has been shown to help decrease 30-day and 90-day mortality associated with VTE events after hospitalization to medical services.8 However, the PPS requires time-consuming manual calculation by a provider, who may be focused on more immediate aspects of patient care and several other risk scores competing for his attention, potentially decreasing its use.
Other risk scores that use only discrete scalar data, such as vital signs and lab results to predict early recognition of sepsis, have been successfully automated and implemented within electronic health records (EHRs).9-11 Successful automation of scores requiring input of diagnoses, recent medical events, and current clinical status such as the PPS remains difficult.12 Data representing these characteristics are more prone to error, and harder to translate clearly into a single data field than discrete elements like heart rate, potentially impacting validity of the calculated result.13 To improve usage of guideline based VTE risk assessment and decrease physician burden, we developed an algorithm called Automated Padua Prediction Score (APPS) that automatically calculates the PPS using only EHR data available within prior encounters and the first 4 hours of admission, a similar timeframe to when admitting providers would be entering orders. Our goal was to assess if an automatically calculated version of the PPS, a score that depends on criteria more complex than vital signs and labs, would accurately assess risk for hospital-acquired VTE when compared to traditional manual calculation of the Padua Prediction Score by a provider.
METHODS
Site Description and Ethics
The study was conducted at University of California, San Francisco Medical Center, a 790-bed academic hospital; its Institutional Review Board approved the study and collection of data via chart review. Handling of patient information complied with the Health Insurance Portability and Accountability Act of 1996.
Patient Inclusion
Adult patients admitted to a medical or surgical service between July 1, 2012 and April 1, 2014 were included in the study if they were candidates for VTEP, defined as: length of stay (LOS) greater than 2 days, not on hospice care, not pregnant at admission, no present on admission VTE diagnosis, no known contraindications to prophylaxis (eg, gastrointestinal bleed), and were not receiving therapeutic doses of warfarin, low molecular weight heparins, heparin, or novel anticoagulants prior to admission.
Data Sources
Clinical variables were extracted from the EHR’s enterprise data warehouse (EDW) by SQL Server query (Microsoft, Redmond, Washington) and deposited in a secure database. Chart review was conducted by a trained researcher (Mr. Jacolbia) using the EHR and a standardized protocol. Findings were recorded using REDCap (REDCap Consortium, Vanderbilt University, Nashville, Tennessee). The specific ICD-9, procedure, and lab codes used to determine each criterion of APPS are available in the Appendix.
Creation of the Automated Padua Prediction Score (APPS)
We developed APPS from the original 11 criteria that comprise the Padua Prediction Score: active cancer, previous VTE (excluding superficial vein thrombosis), reduced mobility, known thrombophilic condition, recent (1 month or less) trauma and/or surgery, age 70 years or older, heart and/or respiratory failure, acute myocardial infarction and/or ischemic stroke, acute infection and/or rheumatologic disorder, body mass index (BMI) 30 or higher, and ongoing hormonal treatment.13 APPS has the same scoring methodology as PPS: criteria are weighted from 1 to 3 points and summed with a maximum score of 20, representing highest risk of VTE. To automate the score calculation from data routinely available in the EHR, APPS checks pre-selected structured data fields for specific values within laboratory results, orders, nursing flowsheets and claims. Claims data included all ICD-9 and procedure codes used for billing purposes. If any of the predetermined data elements are found, then the specific criterion is considered positive; otherwise, it is scored as negative. The creators of the PPS were consulted in the generation of these data queries to replicate the original standards for deeming a criterion positive. The automated calculation required no use of natural language processing.
Characterization of Study Population
We recorded patient demographics (age, race, gender, BMI), LOS, and rate of hospital-acquired VTE. These patients were separated into 2 cohorts determined by the VTE prophylaxis they received. The risk profile of patients who received pharmacologic prophylaxis was hypothesized to be inherently different from those who had not. To evaluate APPS within this heterogeneous cohort, patients were divided into 2 major categories: pharmacologic vs. no pharmacologic prophylaxis. If they had a completed order or medication administration record on the institution’s approved formulary for pharmacologic VTEP, they were considered to have received pharmacologic prophylaxis. If they had only a completed order for usage of mechanical prophylaxis (sequential compression devices) or no evidence of any form of VTEP, they were considered to have received no pharmacologic prophylaxis. Patients with evidence of both pharmacologic and mechanical were placed in the pharmacologic prophylaxis group. To ensure that automated designation of prophylaxis group was accurate, we reviewed 40 randomly chosen charts because prior researchers were able to achieve sensitivity and specificity greater than 90% with that sample size.14
The primary outcome of hospital-acquired VTE was defined as an ICD-9 code for VTE (specific codes are found in the Appendix) paired with a “present on admission = no” flag on that encounter’s hospital billing data, abstracted from the EDW. A previous study at this institution used the same methodology and found 212/226 (94%) of patients with a VTE ICD-9 code on claim had evidence of a hospital-acquired VTE event upon chart review.14 Chart review was also completed to ensure that the primary outcome of newly discovered hospital-acquired VTE was differentiated from chronic VTE or history of VTE. Theoretically, ICD-9 codes and other data elements treat chronic VTE, history of VTE, and hospital-acquired VTE as distinct diagnoses, but it was unclear if this was true in our dataset. For 75 randomly selected cases of presumed hospital-acquired VTE, charts were reviewed for evidence that confirmed newly found VTE during that encounter.
Validation of APPS through Comparison to Manual Calculation of the Original PPS
To compare our automated calculation to standard clinical practice, we manually calculated the PPS through chart review within the first 2 days of admission on 300 random patients, a subsample of the entire study cohort. The largest study we could find had manually calculated the PPS of 1,080 hospitalized patients with a mean PPS of 4.86 (standard deviation [SD], 2.26).15 One researcher (Mr. Jacolbia) accessed the EHR with all patient information available to physicians, including admission notes, orders, labs, flowsheets, past medical history, and all prior encounters to calculate and record the PPS. To limit potential score bias, 2 authors (Drs. Elias and Davies) assessed 30 randomly selected charts from the cohort of 300. The standardized chart review protocol mimicked a physician’s approach to determine if a patient met a criterion, such as concluding if he/she had active cancer by examining medication lists for chemotherapy, procedure notes for radiation, and recent diagnoses on problem lists. After the original PPS was manually calculated, APPS was automatically calculated for the same 300 patients. We intended to characterize similarities and differences between APPS and manual calculation prior to investigating APPS’ predictive capacity for the entire study population, because it would not be feasible to manually calculate the PPS for all 30,726 patients.
Statistical Analysis
For the 75 randomly selected cases of presumed hospital-acquired VTE, the number of cases was chosen by powering our analysis to find a difference in proportion of 20% with 90% power, α = 0.05 (two-sided). We conducted χ2 tests on the entire study cohort to determine if there were significant differences in demographics, LOS, and incidence of hospital-acquired VTE by prophylaxis received. For both the pharmacologic and the no pharmacologic prophylaxis groups, we conducted 2-sample Student t tests to determine significant differences in demographics and LOS between patients who experienced a hospital-acquired VTE and those who did not.
For the comparison of our automated calculation to standard clinical practice, we manually calculated the PPS through chart review within the first 2 days of admission on a subsample of 300 random patients. We powered our analysis to detect a difference in mean PPS from 4.86 to 4.36, enough to alter the point value, with 90% power and α = 0.05 (two-sided) and found 300 patients to be comfortably above the required sample size. We compared APPS and manual calculation in the 300-patient cohort using: 2-sample Student t tests to compare mean scores, χ2 tests to compare the frequency with which criteria were positive, and receiver operating characteristic (ROC) curves to determine capacity to predict a hospital-acquired VTE event. Pearson’s correlation was also completed to assess score agreement between APPS and manual calculation on a per-patient basis. After comparing automated calculation of APPS to manual chart review on the same 300 patients, we used APPS to calculate scores for the entire study cohort (n = 30,726). We calculated the mean of APPS by prophylaxis group and whether hospital-acquired VTE had occurred. We analyzed APPS’ ROC curve statistics by prophylaxis group to determine its overall predictive capacity in our study population. Lastly, we computed the time required to calculate APPS per patient. Statistical analyses were conducted using SPSS Statistics (IBM, Armonk, New York) and Python 2.7 (Python Software Foundation, Beaverton, Oregon); 95% confidence intervals (CI) and (SD) were reported when appropriate.
RESULTS
Among the 30,726 unique patients in our entire cohort (all patients admitted during the time period who met the study criteria), we found 6574 (21.4%) on pharmacologic (with or without mechanical) prophylaxis, 13,511 (44.0%) on mechanical only, and 10,641 (34.6%) on no prophylaxis. χ2 tests found no significant differences in demographics, LOS, or incidence of hospital-acquired VTE between the patients who received mechanical prophylaxis only and those who received no prophylaxis (Table 1). Similarly, there were no differences in these characteristics in patients receiving pharmacologic prophylaxis with or without the addition of mechanical prophylaxis. Designation of prophylaxis group by manual chart review vs. our automated process was found to agree in categorization for 39/40 (97.5%) sampled encounters. When comparing the cohort that received pharmacologic prophylaxis against the cohort that did not, there were significant differences in racial distribution, sex, BMI, and average LOS as shown in Table 1. Those who received pharmacologic prophylaxis were found to be significantly older than those who did not (62.7 years versus 53.2 years, P < 0.001), more likely to be male (50.6% vs, 42.4%, P < 0.001), more likely to have hospital-acquired VTE (2.2% vs. 0.5%, P < 0.001), and to have a shorter LOS (7.1 days vs. 9.8, P < 0.001).
Within the cohort group receiving pharmacologic prophylaxis (n = 6574), hospital-acquired VTE occurred in patients who were significantly younger (58.2 years vs. 62.8 years, P = 0.003) with a greater LOS (23.8 days vs. 6.7, P < 0.001) than those without. Within the group receiving no pharmacologic prophylaxis (n = 24,152), hospital-acquired VTE occurred in patients who were significantly older (57.1 years vs. 53.2 years, P = 0.014) with more than twice the LOS (20.2 days vs. 9.7 days, P < 0.001) compared to those without. Sixty-six of 75 (88%) randomly selected patients in which new VTE was identified by the automated electronic query had this diagnosis confirmed during manual chart review.
As shown in Table 2, automated calculation on a subsample of 300 randomly selected patients using APPS had a mean of 5.5 (SD, 2.9) while manual calculation of the original PPS on the same patients had a mean of 5.1 (SD, 2.6). There was no significant difference in mean between manual calculation and APPS (P = 0.073). There were, however, significant differences in how often individual criteria were considered present. The largest contributors to the difference in scores between APPS and manual calculation were “prior VTE” (positive, 16% vs. 8.3%, respectively) and “reduced mobility” (positive, 74.3% vs. 66%, respectively) as shown in Table 2. In the subsample, there were a total of 6 (2.0%) hospital-acquired VTE events. APPS’ automated calculation had an AUC = 0.79 (CI, 0.63-0.95) that was significant (P = 0.016) with a cutoff value of 5. Chart review’s manual calculation of the PPS had an AUC = 0.76 (CI 0.61-0.91) that was also significant (P = 0.029).
Distribution of Patient Characteristics in Cohort
Our entire cohort of 30,726 unique patients admitted during the study period included 260 (0.8%) who experienced hospital-acquired VTEs (Table 3). In patients receiving no pharmacologic prophylaxis, the average APPS was 4.0 (SD, 2.4) for those without VTE and 7.1 (SD, 2.3) for those with VTE. In patients who had received pharmacologic prophylaxis, those without hospital-acquired VTE had an average APPS of 4.9 (SD, 2.6) and those with hospital-acquired VTE averaged 7.7 (SD, 2.6). APPS’ ROC curves for “no pharmacologic prophylaxis” had an AUC = 0.81 (CI, 0.79 – 0.83) that was significant (P < 0.001) with a cutoff value of 5. There was similar performance in the pharmacologic prophylaxis group with an AUC = 0.79 (CI, 0.76 – 0.82) and cutoff value of 5, as shown in the Figure. Over the entire cohort, APPS had a sensitivity of 85.4%, specificity of 53.3%, positive predictive value (PPV) of 1.5%, and a negative predictive value (NPV) of 99.8% when using a cutoff of 5. The average APPS calculation time was 0.03 seconds per encounter. Additional information on individual criteria can be found in Table 3.
DISCUSSION
Automated calculation of APPS using EHR data from prior encounters and the first 4 hours of admission was predictive of in-hospital VTE. APPS performed as well as traditional manual score calculation of the PPS. It was able to do so with no physician input, significantly lessening the burden of calculation and potentially increasing frequency of data-driven VTE risk assessment.
While automated calculation of certain scores is becoming more common, risk calculators that require data beyond vital signs and lab results have lagged,16-19 in part because of uncertainty about 2 issues. The first is whether EHR data accurately represent the current clinical picture. The second is if a machine-interpretable algorithm to determine a clinical status (eg, “active cancer”) would be similar to a doctor’s perception of that same concept. We attempted to better understand these 2 challenges through developing APPS. Concerning accuracy, EHR data correctly represent the clinical scenario: designations of VTEP and hospital-acquired VTE were accurate in approximately 90% of reviewed cases. Regarding the second concern, when comparing APPS to manual calculation, we found significant differences (P < 0.001) in how often 8 of the 11 criteria were positive, yet no significant difference in overall score and similar predictive capacity. Manual calculation appeared more likely to find data in the index encounter or in structured data. For example, “active cancer” may be documented only in a physician’s note, easily accounted for during a physician’s calculation but missed by APPS looking only for structured data. In contrast, automated calculation found historic criteria, such as “prior VTE” or “known thrombophilic condition,” positive more often. If the patient is being admitted for a problem unrelated to blood clots, the physician may have little time or interest to look through hundreds of EHR documents to discover a 2-year-old VTE. As patients’ records become larger and denser, more historic data can become buried and forgotten. While the 2 scores differ on individual criteria, they are similarly predictive and able to bifurcate the at-risk population to those who should and should not receive pharmacologic prophylaxis.
The APPS was found to have near-equal performance in the pharmacologic vs. no pharmacologic prophylaxis cohorts. This finding agrees with a study that found no significant difference in predicting 90-day VTE when looking at 86 risk factors vs. the most significant 4, none of which related to prescribed prophylaxis.18 The original PPS had a reported sensitivity of 94.6%, specificity 62%, PPV 7.5%, and NPV 99.7% in its derivation cohort.13 We matched APPS to the ratio of sensitivity to specificity, using 5 as the cutoff value. APPS performed slightly worse with sensitivity of 85.4%, specificity 53.3%, PPV 1.5%, and NPV 99.8%. This difference may have resulted from the original PPS study’s use of 90-day follow-up to determine VTE occurrence, whereas we looked only until the end of current hospitalization, an average of 9.2 days. Furthermore, the PPS had significantly poorer performance (AUC = 0.62) than that seen in the original derivation cohort in a separate study that manually calculated the score on more than 1000 patients.15
There are important limitations to our study. It was done at a single academic institution using a dataset of VTE-associated, validated research that was well-known to the researchers.20 Another major limitation is the dependence of the algorithm on data available within the first 4 hours of admission and earlier; thus, previous encounters may frequently play an important role. Patients presenting to our health system for the first time would have significantly fewer data available at the time of calculation. Additionally, our data could not reliably tell us the total doses of pharmacologic prophylaxis that a patient received. While most patients will maintain a consistent VTEP regimen once initiated in the hospital, 2 patients with the same LOS may have received differing amounts of pharmacologic prophylaxis. This research study did not assess how much time automatic calculation of VTE risk might save providers, because we did not record the time for each manual abstraction; however, from discussion with the main abstracter, chart review and manual calculation for this study took from 2 to 14 minutes per patient, depending on the number of previous interactions with the health system. Finally, although we chose data elements that are likely to exist at most institutions using an EHR, many institutions’ EHRs do not have EDW capabilities nor programmers who can assist with an automated risk score.
The EHR interventions to assist providers in determining appropriate VTEP have been able to increase rates of VTEP and decrease VTE-associated mortality.16,21 In addition to automating the calculation of guideline-adherent risk scores, there is a need for wider adoption for clinical decision support for VTE. For this reason, we chose only structured data fields from some of the most common elements within our EHR’s data warehouse to derive APPS (Appendix 1). Our study supports the idea that automated calculation of scores requiring input of more complex data such as diagnoses, recent medical events, and current clinical status remains predictive of hospital-acquired VTE risk. Because it is calculated automatically in the background while the clinician completes his or her assessment, the APPS holds the potential to significantly reduce the burden on providers while making guideline-adherent risk assessment more readily accessible. Further research is required to determine the exact amount of time automatic calculation saves, and, more important, if the relatively high predictive capacity we observed using APPS would be reproducible across institutions and could reduce incidence of hospital-acquired VTE.
Disclosures
Dr. Auerbach was supported by NHLBI K24HL098372 during the period of this study. Dr. Khanna, who is an implementation scientist at the University of California San Francisco Center for Digital Health Innovation, is the principal inventor of CareWeb, and may benefit financially from its commercialization. The other authors report no financial conflicts of interest.
Hospital-acquired venous thromboembolism (VTE) continues to be a critical quality challenge for U.S. hospitals,1 and high-risk patients are often not adequately prophylaxed. Use of VTE prophylaxis (VTEP) varies as widely as 26% to 85% of patients in various studies, as does patient outcomes and care expenditures.2-6 The 9th edition of the American College of Chest Physicians (CHEST) guidelines7 recommend the Padua Prediction Score (PPS) to select individual patients who may be at high risk for venous thromboembolism (VTE) and could benefit from thromboprophylaxis. Use of the manually calculated PPS to select patients for thromboprophylaxis has been shown to help decrease 30-day and 90-day mortality associated with VTE events after hospitalization to medical services.8 However, the PPS requires time-consuming manual calculation by a provider, who may be focused on more immediate aspects of patient care and several other risk scores competing for his attention, potentially decreasing its use.
Other risk scores that use only discrete scalar data, such as vital signs and lab results to predict early recognition of sepsis, have been successfully automated and implemented within electronic health records (EHRs).9-11 Successful automation of scores requiring input of diagnoses, recent medical events, and current clinical status such as the PPS remains difficult.12 Data representing these characteristics are more prone to error, and harder to translate clearly into a single data field than discrete elements like heart rate, potentially impacting validity of the calculated result.13 To improve usage of guideline based VTE risk assessment and decrease physician burden, we developed an algorithm called Automated Padua Prediction Score (APPS) that automatically calculates the PPS using only EHR data available within prior encounters and the first 4 hours of admission, a similar timeframe to when admitting providers would be entering orders. Our goal was to assess if an automatically calculated version of the PPS, a score that depends on criteria more complex than vital signs and labs, would accurately assess risk for hospital-acquired VTE when compared to traditional manual calculation of the Padua Prediction Score by a provider.
METHODS
Site Description and Ethics
The study was conducted at University of California, San Francisco Medical Center, a 790-bed academic hospital; its Institutional Review Board approved the study and collection of data via chart review. Handling of patient information complied with the Health Insurance Portability and Accountability Act of 1996.
Patient Inclusion
Adult patients admitted to a medical or surgical service between July 1, 2012 and April 1, 2014 were included in the study if they were candidates for VTEP, defined as: length of stay (LOS) greater than 2 days, not on hospice care, not pregnant at admission, no present on admission VTE diagnosis, no known contraindications to prophylaxis (eg, gastrointestinal bleed), and were not receiving therapeutic doses of warfarin, low molecular weight heparins, heparin, or novel anticoagulants prior to admission.
Data Sources
Clinical variables were extracted from the EHR’s enterprise data warehouse (EDW) by SQL Server query (Microsoft, Redmond, Washington) and deposited in a secure database. Chart review was conducted by a trained researcher (Mr. Jacolbia) using the EHR and a standardized protocol. Findings were recorded using REDCap (REDCap Consortium, Vanderbilt University, Nashville, Tennessee). The specific ICD-9, procedure, and lab codes used to determine each criterion of APPS are available in the Appendix.
Creation of the Automated Padua Prediction Score (APPS)
We developed APPS from the original 11 criteria that comprise the Padua Prediction Score: active cancer, previous VTE (excluding superficial vein thrombosis), reduced mobility, known thrombophilic condition, recent (1 month or less) trauma and/or surgery, age 70 years or older, heart and/or respiratory failure, acute myocardial infarction and/or ischemic stroke, acute infection and/or rheumatologic disorder, body mass index (BMI) 30 or higher, and ongoing hormonal treatment.13 APPS has the same scoring methodology as PPS: criteria are weighted from 1 to 3 points and summed with a maximum score of 20, representing highest risk of VTE. To automate the score calculation from data routinely available in the EHR, APPS checks pre-selected structured data fields for specific values within laboratory results, orders, nursing flowsheets and claims. Claims data included all ICD-9 and procedure codes used for billing purposes. If any of the predetermined data elements are found, then the specific criterion is considered positive; otherwise, it is scored as negative. The creators of the PPS were consulted in the generation of these data queries to replicate the original standards for deeming a criterion positive. The automated calculation required no use of natural language processing.
Characterization of Study Population
We recorded patient demographics (age, race, gender, BMI), LOS, and rate of hospital-acquired VTE. These patients were separated into 2 cohorts determined by the VTE prophylaxis they received. The risk profile of patients who received pharmacologic prophylaxis was hypothesized to be inherently different from those who had not. To evaluate APPS within this heterogeneous cohort, patients were divided into 2 major categories: pharmacologic vs. no pharmacologic prophylaxis. If they had a completed order or medication administration record on the institution’s approved formulary for pharmacologic VTEP, they were considered to have received pharmacologic prophylaxis. If they had only a completed order for usage of mechanical prophylaxis (sequential compression devices) or no evidence of any form of VTEP, they were considered to have received no pharmacologic prophylaxis. Patients with evidence of both pharmacologic and mechanical were placed in the pharmacologic prophylaxis group. To ensure that automated designation of prophylaxis group was accurate, we reviewed 40 randomly chosen charts because prior researchers were able to achieve sensitivity and specificity greater than 90% with that sample size.14
The primary outcome of hospital-acquired VTE was defined as an ICD-9 code for VTE (specific codes are found in the Appendix) paired with a “present on admission = no” flag on that encounter’s hospital billing data, abstracted from the EDW. A previous study at this institution used the same methodology and found 212/226 (94%) of patients with a VTE ICD-9 code on claim had evidence of a hospital-acquired VTE event upon chart review.14 Chart review was also completed to ensure that the primary outcome of newly discovered hospital-acquired VTE was differentiated from chronic VTE or history of VTE. Theoretically, ICD-9 codes and other data elements treat chronic VTE, history of VTE, and hospital-acquired VTE as distinct diagnoses, but it was unclear if this was true in our dataset. For 75 randomly selected cases of presumed hospital-acquired VTE, charts were reviewed for evidence that confirmed newly found VTE during that encounter.
Validation of APPS through Comparison to Manual Calculation of the Original PPS
To compare our automated calculation to standard clinical practice, we manually calculated the PPS through chart review within the first 2 days of admission on 300 random patients, a subsample of the entire study cohort. The largest study we could find had manually calculated the PPS of 1,080 hospitalized patients with a mean PPS of 4.86 (standard deviation [SD], 2.26).15 One researcher (Mr. Jacolbia) accessed the EHR with all patient information available to physicians, including admission notes, orders, labs, flowsheets, past medical history, and all prior encounters to calculate and record the PPS. To limit potential score bias, 2 authors (Drs. Elias and Davies) assessed 30 randomly selected charts from the cohort of 300. The standardized chart review protocol mimicked a physician’s approach to determine if a patient met a criterion, such as concluding if he/she had active cancer by examining medication lists for chemotherapy, procedure notes for radiation, and recent diagnoses on problem lists. After the original PPS was manually calculated, APPS was automatically calculated for the same 300 patients. We intended to characterize similarities and differences between APPS and manual calculation prior to investigating APPS’ predictive capacity for the entire study population, because it would not be feasible to manually calculate the PPS for all 30,726 patients.
Statistical Analysis
For the 75 randomly selected cases of presumed hospital-acquired VTE, the number of cases was chosen by powering our analysis to find a difference in proportion of 20% with 90% power, α = 0.05 (two-sided). We conducted χ2 tests on the entire study cohort to determine if there were significant differences in demographics, LOS, and incidence of hospital-acquired VTE by prophylaxis received. For both the pharmacologic and the no pharmacologic prophylaxis groups, we conducted 2-sample Student t tests to determine significant differences in demographics and LOS between patients who experienced a hospital-acquired VTE and those who did not.
For the comparison of our automated calculation to standard clinical practice, we manually calculated the PPS through chart review within the first 2 days of admission on a subsample of 300 random patients. We powered our analysis to detect a difference in mean PPS from 4.86 to 4.36, enough to alter the point value, with 90% power and α = 0.05 (two-sided) and found 300 patients to be comfortably above the required sample size. We compared APPS and manual calculation in the 300-patient cohort using: 2-sample Student t tests to compare mean scores, χ2 tests to compare the frequency with which criteria were positive, and receiver operating characteristic (ROC) curves to determine capacity to predict a hospital-acquired VTE event. Pearson’s correlation was also completed to assess score agreement between APPS and manual calculation on a per-patient basis. After comparing automated calculation of APPS to manual chart review on the same 300 patients, we used APPS to calculate scores for the entire study cohort (n = 30,726). We calculated the mean of APPS by prophylaxis group and whether hospital-acquired VTE had occurred. We analyzed APPS’ ROC curve statistics by prophylaxis group to determine its overall predictive capacity in our study population. Lastly, we computed the time required to calculate APPS per patient. Statistical analyses were conducted using SPSS Statistics (IBM, Armonk, New York) and Python 2.7 (Python Software Foundation, Beaverton, Oregon); 95% confidence intervals (CI) and (SD) were reported when appropriate.
RESULTS
Among the 30,726 unique patients in our entire cohort (all patients admitted during the time period who met the study criteria), we found 6574 (21.4%) on pharmacologic (with or without mechanical) prophylaxis, 13,511 (44.0%) on mechanical only, and 10,641 (34.6%) on no prophylaxis. χ2 tests found no significant differences in demographics, LOS, or incidence of hospital-acquired VTE between the patients who received mechanical prophylaxis only and those who received no prophylaxis (Table 1). Similarly, there were no differences in these characteristics in patients receiving pharmacologic prophylaxis with or without the addition of mechanical prophylaxis. Designation of prophylaxis group by manual chart review vs. our automated process was found to agree in categorization for 39/40 (97.5%) sampled encounters. When comparing the cohort that received pharmacologic prophylaxis against the cohort that did not, there were significant differences in racial distribution, sex, BMI, and average LOS as shown in Table 1. Those who received pharmacologic prophylaxis were found to be significantly older than those who did not (62.7 years versus 53.2 years, P < 0.001), more likely to be male (50.6% vs, 42.4%, P < 0.001), more likely to have hospital-acquired VTE (2.2% vs. 0.5%, P < 0.001), and to have a shorter LOS (7.1 days vs. 9.8, P < 0.001).
Within the cohort group receiving pharmacologic prophylaxis (n = 6574), hospital-acquired VTE occurred in patients who were significantly younger (58.2 years vs. 62.8 years, P = 0.003) with a greater LOS (23.8 days vs. 6.7, P < 0.001) than those without. Within the group receiving no pharmacologic prophylaxis (n = 24,152), hospital-acquired VTE occurred in patients who were significantly older (57.1 years vs. 53.2 years, P = 0.014) with more than twice the LOS (20.2 days vs. 9.7 days, P < 0.001) compared to those without. Sixty-six of 75 (88%) randomly selected patients in which new VTE was identified by the automated electronic query had this diagnosis confirmed during manual chart review.
As shown in Table 2, automated calculation on a subsample of 300 randomly selected patients using APPS had a mean of 5.5 (SD, 2.9) while manual calculation of the original PPS on the same patients had a mean of 5.1 (SD, 2.6). There was no significant difference in mean between manual calculation and APPS (P = 0.073). There were, however, significant differences in how often individual criteria were considered present. The largest contributors to the difference in scores between APPS and manual calculation were “prior VTE” (positive, 16% vs. 8.3%, respectively) and “reduced mobility” (positive, 74.3% vs. 66%, respectively) as shown in Table 2. In the subsample, there were a total of 6 (2.0%) hospital-acquired VTE events. APPS’ automated calculation had an AUC = 0.79 (CI, 0.63-0.95) that was significant (P = 0.016) with a cutoff value of 5. Chart review’s manual calculation of the PPS had an AUC = 0.76 (CI 0.61-0.91) that was also significant (P = 0.029).
Distribution of Patient Characteristics in Cohort
Our entire cohort of 30,726 unique patients admitted during the study period included 260 (0.8%) who experienced hospital-acquired VTEs (Table 3). In patients receiving no pharmacologic prophylaxis, the average APPS was 4.0 (SD, 2.4) for those without VTE and 7.1 (SD, 2.3) for those with VTE. In patients who had received pharmacologic prophylaxis, those without hospital-acquired VTE had an average APPS of 4.9 (SD, 2.6) and those with hospital-acquired VTE averaged 7.7 (SD, 2.6). APPS’ ROC curves for “no pharmacologic prophylaxis” had an AUC = 0.81 (CI, 0.79 – 0.83) that was significant (P < 0.001) with a cutoff value of 5. There was similar performance in the pharmacologic prophylaxis group with an AUC = 0.79 (CI, 0.76 – 0.82) and cutoff value of 5, as shown in the Figure. Over the entire cohort, APPS had a sensitivity of 85.4%, specificity of 53.3%, positive predictive value (PPV) of 1.5%, and a negative predictive value (NPV) of 99.8% when using a cutoff of 5. The average APPS calculation time was 0.03 seconds per encounter. Additional information on individual criteria can be found in Table 3.
DISCUSSION
Automated calculation of APPS using EHR data from prior encounters and the first 4 hours of admission was predictive of in-hospital VTE. APPS performed as well as traditional manual score calculation of the PPS. It was able to do so with no physician input, significantly lessening the burden of calculation and potentially increasing frequency of data-driven VTE risk assessment.
While automated calculation of certain scores is becoming more common, risk calculators that require data beyond vital signs and lab results have lagged,16-19 in part because of uncertainty about 2 issues. The first is whether EHR data accurately represent the current clinical picture. The second is if a machine-interpretable algorithm to determine a clinical status (eg, “active cancer”) would be similar to a doctor’s perception of that same concept. We attempted to better understand these 2 challenges through developing APPS. Concerning accuracy, EHR data correctly represent the clinical scenario: designations of VTEP and hospital-acquired VTE were accurate in approximately 90% of reviewed cases. Regarding the second concern, when comparing APPS to manual calculation, we found significant differences (P < 0.001) in how often 8 of the 11 criteria were positive, yet no significant difference in overall score and similar predictive capacity. Manual calculation appeared more likely to find data in the index encounter or in structured data. For example, “active cancer” may be documented only in a physician’s note, easily accounted for during a physician’s calculation but missed by APPS looking only for structured data. In contrast, automated calculation found historic criteria, such as “prior VTE” or “known thrombophilic condition,” positive more often. If the patient is being admitted for a problem unrelated to blood clots, the physician may have little time or interest to look through hundreds of EHR documents to discover a 2-year-old VTE. As patients’ records become larger and denser, more historic data can become buried and forgotten. While the 2 scores differ on individual criteria, they are similarly predictive and able to bifurcate the at-risk population to those who should and should not receive pharmacologic prophylaxis.
The APPS was found to have near-equal performance in the pharmacologic vs. no pharmacologic prophylaxis cohorts. This finding agrees with a study that found no significant difference in predicting 90-day VTE when looking at 86 risk factors vs. the most significant 4, none of which related to prescribed prophylaxis.18 The original PPS had a reported sensitivity of 94.6%, specificity 62%, PPV 7.5%, and NPV 99.7% in its derivation cohort.13 We matched APPS to the ratio of sensitivity to specificity, using 5 as the cutoff value. APPS performed slightly worse with sensitivity of 85.4%, specificity 53.3%, PPV 1.5%, and NPV 99.8%. This difference may have resulted from the original PPS study’s use of 90-day follow-up to determine VTE occurrence, whereas we looked only until the end of current hospitalization, an average of 9.2 days. Furthermore, the PPS had significantly poorer performance (AUC = 0.62) than that seen in the original derivation cohort in a separate study that manually calculated the score on more than 1000 patients.15
There are important limitations to our study. It was done at a single academic institution using a dataset of VTE-associated, validated research that was well-known to the researchers.20 Another major limitation is the dependence of the algorithm on data available within the first 4 hours of admission and earlier; thus, previous encounters may frequently play an important role. Patients presenting to our health system for the first time would have significantly fewer data available at the time of calculation. Additionally, our data could not reliably tell us the total doses of pharmacologic prophylaxis that a patient received. While most patients will maintain a consistent VTEP regimen once initiated in the hospital, 2 patients with the same LOS may have received differing amounts of pharmacologic prophylaxis. This research study did not assess how much time automatic calculation of VTE risk might save providers, because we did not record the time for each manual abstraction; however, from discussion with the main abstracter, chart review and manual calculation for this study took from 2 to 14 minutes per patient, depending on the number of previous interactions with the health system. Finally, although we chose data elements that are likely to exist at most institutions using an EHR, many institutions’ EHRs do not have EDW capabilities nor programmers who can assist with an automated risk score.
The EHR interventions to assist providers in determining appropriate VTEP have been able to increase rates of VTEP and decrease VTE-associated mortality.16,21 In addition to automating the calculation of guideline-adherent risk scores, there is a need for wider adoption for clinical decision support for VTE. For this reason, we chose only structured data fields from some of the most common elements within our EHR’s data warehouse to derive APPS (Appendix 1). Our study supports the idea that automated calculation of scores requiring input of more complex data such as diagnoses, recent medical events, and current clinical status remains predictive of hospital-acquired VTE risk. Because it is calculated automatically in the background while the clinician completes his or her assessment, the APPS holds the potential to significantly reduce the burden on providers while making guideline-adherent risk assessment more readily accessible. Further research is required to determine the exact amount of time automatic calculation saves, and, more important, if the relatively high predictive capacity we observed using APPS would be reproducible across institutions and could reduce incidence of hospital-acquired VTE.
Disclosures
Dr. Auerbach was supported by NHLBI K24HL098372 during the period of this study. Dr. Khanna, who is an implementation scientist at the University of California San Francisco Center for Digital Health Innovation, is the principal inventor of CareWeb, and may benefit financially from its commercialization. The other authors report no financial conflicts of interest.
1. Galson S. The Surgeon General’s call to action to prevent deep vein thrombosis and pulmonary embolism. 2008. https://www.ncbi.nlm.nih.gov/books/NBK44178/. Accessed February 11, 2016. PubMed
2. Borch KH, Nyegaard C, Hansen JB, et al. Joint effects of obesity and body height on the risk of venous thromboembolism: the Tromsø study. Arterioscler Thromb Vasc Biol. 2011;31(6):1439-44. PubMed
3. Braekkan SK, Borch KH, Mathiesen EB, Njølstad I, Wilsgaard T, Hansen JB.. Body height and risk of venous thromboembolism: the Tromsø Study. Am J Epidemiol. 2010;171(10):1109-1115. PubMed
4. Bounameaux H, Rosendaal FR. Venous thromboembolism: why does ethnicity matter? Circulation. 2011;123(200:2189-2191. PubMed
5. Spyropoulos AC, Anderson FA Jr, Fitzgerald G, et al; IMPROVE Investigators. Predictive and associative models to identify hospitalized medical patients at risk for VTE. Chest. 2011;140(3):706-714. PubMed
6. Rothberg MB, Lindenauer PK, Lahti M, Pekow PS, Selker HP. Risk factor model to predict venous thromboembolism in hospitalized medical patients. J Hosp Med. 2011;6(4):202-209. PubMed
7. Perioperative Management of Antithrombotic Therapy: Prevention of VTE in Nonsurgical Patients: Antithrombotic Therapy and Prevention of Thrombosis, 9th ed: American College of Chest Physicians Evidence-Based Clinical Practice Guidelines. Chest. 2012;141(6):1645.
8. Subbe CP, Kruger M, Rutherford P, Gemmel L. Validation of a modified Early Warning Score in medical admissions. QJM. 2001;94(10):521-526. PubMed
9. Alvarez CA, Clark CA, Zhang S, et al. Predicting out of intensive care unit cardiopulmonary arrest or death using electronic medical record data. BMC Med Inform Decis Mak. 2013;13:28. PubMed
10. Escobar GJ, LaGuardia JC, Turk BJ, Ragins A, Kipnis P, Draper D. Early detection of impending physiologic deterioration among patients who are not in intensive care: development of predictive models using data from an automated electronic medical record. J Hosp Med. 2012;7(5):388-395. PubMed
11. Umscheid CA, Hanish A, Chittams J, Weiner MG, Hecht TE. Effectiveness of a novel and scalable clinical decision support intervention to improve venous thromboembolism prophylaxis: a quasi-experimental study. BMC Med Inform Decis Mak. 2012;12:92. PubMed
12. Tepas JJ 3rd, Rimar JM, Hsiao AL, Nussbaum MS. Automated analysis of electronic medical record data reflects the pathophysiology of operative complications. Surgery. 2013;154(4):918-924. PubMed
13. Barbar S, Noventa F, Rossetto V, et al. A risk assessment model for the identification of hospitalized medical patients at risk for venous thromboembolism: the Padua Prediction Score. J Thromb Haemost. 2010; 8(11):2450-2457. PubMed
14. Khanna R, Maynard G, Sadeghi B, et al. Incidence of hospital-acquired venous thromboembolic codes in medical patients hospitalized in academic medical centers. J Hosp Med. 2014; 9(4):221-225. PubMed
15. Vardi M, Ghanem-Zoubi NO, Zidan R, Yurin V, Bitterman H. Venous thromboembolism and the utility of the Padua Prediction Score in patients with sepsis admitted to internal medicine departments. J Thromb Haemost. 2013;11(3):467-473. PubMed
16. Samama MM, Dahl OE, Mismetti P, et al. An electronic tool for venous thromboembolism prevention in medical and surgical patients. Haematologica. 2006;91(1):64-70. PubMed
17. Mann DM, Kannry JL, Edonyabo D, et al. Rationale, design, and implementation protocol of an electronic health record integrated clinical prediction rule (iCPR) randomized trial in primary care. Implement Sci. 2011;6:109. PubMed
18. Woller SC, Stevens SM, Jones JP, et al. Derivation and validation of a simple model to identify venous thromboembolism risk in medical patients. Am J Med. 2011;124(10):947-954. PubMed
19. Huang W, Anderson FA, Spencer FA, Gallus A, Goldberg RJ. Risk-assessment models for predicting venous thromboembolism among hospitalized non-surgical patients: a systematic review. J Thromb Thrombolysis. 2013;35(1):67-80. PubMed
20. Khanna RR, Kim SB, Jenkins I, et al. Predictive value of the present-on-admission indicator for hospital-acquired venous thromboembolism. Med Care. 2015;53(4):e31-e36. PubMed
21. Kucher N, Koo S, Quiroz R, et al. Electronic alerts to prevent venous thromboembolism a
1. Galson S. The Surgeon General’s call to action to prevent deep vein thrombosis and pulmonary embolism. 2008. https://www.ncbi.nlm.nih.gov/books/NBK44178/. Accessed February 11, 2016. PubMed
2. Borch KH, Nyegaard C, Hansen JB, et al. Joint effects of obesity and body height on the risk of venous thromboembolism: the Tromsø study. Arterioscler Thromb Vasc Biol. 2011;31(6):1439-44. PubMed
3. Braekkan SK, Borch KH, Mathiesen EB, Njølstad I, Wilsgaard T, Hansen JB.. Body height and risk of venous thromboembolism: the Tromsø Study. Am J Epidemiol. 2010;171(10):1109-1115. PubMed
4. Bounameaux H, Rosendaal FR. Venous thromboembolism: why does ethnicity matter? Circulation. 2011;123(200:2189-2191. PubMed
5. Spyropoulos AC, Anderson FA Jr, Fitzgerald G, et al; IMPROVE Investigators. Predictive and associative models to identify hospitalized medical patients at risk for VTE. Chest. 2011;140(3):706-714. PubMed
6. Rothberg MB, Lindenauer PK, Lahti M, Pekow PS, Selker HP. Risk factor model to predict venous thromboembolism in hospitalized medical patients. J Hosp Med. 2011;6(4):202-209. PubMed
7. Perioperative Management of Antithrombotic Therapy: Prevention of VTE in Nonsurgical Patients: Antithrombotic Therapy and Prevention of Thrombosis, 9th ed: American College of Chest Physicians Evidence-Based Clinical Practice Guidelines. Chest. 2012;141(6):1645.
8. Subbe CP, Kruger M, Rutherford P, Gemmel L. Validation of a modified Early Warning Score in medical admissions. QJM. 2001;94(10):521-526. PubMed
9. Alvarez CA, Clark CA, Zhang S, et al. Predicting out of intensive care unit cardiopulmonary arrest or death using electronic medical record data. BMC Med Inform Decis Mak. 2013;13:28. PubMed
10. Escobar GJ, LaGuardia JC, Turk BJ, Ragins A, Kipnis P, Draper D. Early detection of impending physiologic deterioration among patients who are not in intensive care: development of predictive models using data from an automated electronic medical record. J Hosp Med. 2012;7(5):388-395. PubMed
11. Umscheid CA, Hanish A, Chittams J, Weiner MG, Hecht TE. Effectiveness of a novel and scalable clinical decision support intervention to improve venous thromboembolism prophylaxis: a quasi-experimental study. BMC Med Inform Decis Mak. 2012;12:92. PubMed
12. Tepas JJ 3rd, Rimar JM, Hsiao AL, Nussbaum MS. Automated analysis of electronic medical record data reflects the pathophysiology of operative complications. Surgery. 2013;154(4):918-924. PubMed
13. Barbar S, Noventa F, Rossetto V, et al. A risk assessment model for the identification of hospitalized medical patients at risk for venous thromboembolism: the Padua Prediction Score. J Thromb Haemost. 2010; 8(11):2450-2457. PubMed
14. Khanna R, Maynard G, Sadeghi B, et al. Incidence of hospital-acquired venous thromboembolic codes in medical patients hospitalized in academic medical centers. J Hosp Med. 2014; 9(4):221-225. PubMed
15. Vardi M, Ghanem-Zoubi NO, Zidan R, Yurin V, Bitterman H. Venous thromboembolism and the utility of the Padua Prediction Score in patients with sepsis admitted to internal medicine departments. J Thromb Haemost. 2013;11(3):467-473. PubMed
16. Samama MM, Dahl OE, Mismetti P, et al. An electronic tool for venous thromboembolism prevention in medical and surgical patients. Haematologica. 2006;91(1):64-70. PubMed
17. Mann DM, Kannry JL, Edonyabo D, et al. Rationale, design, and implementation protocol of an electronic health record integrated clinical prediction rule (iCPR) randomized trial in primary care. Implement Sci. 2011;6:109. PubMed
18. Woller SC, Stevens SM, Jones JP, et al. Derivation and validation of a simple model to identify venous thromboembolism risk in medical patients. Am J Med. 2011;124(10):947-954. PubMed
19. Huang W, Anderson FA, Spencer FA, Gallus A, Goldberg RJ. Risk-assessment models for predicting venous thromboembolism among hospitalized non-surgical patients: a systematic review. J Thromb Thrombolysis. 2013;35(1):67-80. PubMed
20. Khanna RR, Kim SB, Jenkins I, et al. Predictive value of the present-on-admission indicator for hospital-acquired venous thromboembolism. Med Care. 2015;53(4):e31-e36. PubMed
21. Kucher N, Koo S, Quiroz R, et al. Electronic alerts to prevent venous thromboembolism a
© 2017 Society of Hospital Medicine
Reducing HA VTE in 5 Academic Centers
Venous thromboembolism (VTE), comprised of pulmonary embolism (PE) and deep vein thrombosis (DVT), impacts hundreds of thousands of Americans annually.[1] The complications of VTE can be severe, including the post‐thrombotic syndrome, pulmonary hypertension, and complications of anticoagulation. VTE is often a complication of hospitalization, and PE is a common preventable cause of hospital mortality.[2, 3] Pharmacologic VTE prophylaxis (VTEP) in at‐risk patients is effective and endorsed by prominent guidelines.[4, 5, 6] However, VTEP is underutilized, with only 30% to 50% of eligible patients receiving the right drug, dose, and duration.[7, 8]
Public reporting and reimbursement policies reflect the magnitude of VTE as a public health concern. The Centers for Medicare and Medicaid Services (CMS) withholds incremental payment for VTE complications.[9] The rate of hospital‐associated VTE (HA‐VTE) is used by benchmarking organizations as a quality indicator.[10, 11]
The University of California (UC) has 5 major academic medical centers, located in Irvine (UCI), Los Angeles (UCLA), Sacramento (UC Davis [UCD]), San Diego (UCSD), and San Francisco (UCSF). In both 2010 and 2011, almost 700 UC patients suffered from HA‐VTE annually. Barriers to optimal VTEP included the absence of standardized VTE risk assessment, lack of consensus on appropriate VTEP options for various inpatient populations, and a lack of collaborative infrastructure. Other barriers included poor adherence to mechanical prophylaxis and suboptimal measurement of prophylaxis and HA‐VTE outcomes.
In late 2011, leaders from the 5 medical centers, supported by an internal competitive grant from the UC Office of the President and the Center for Health Quality and Innovation, formed a collaborative to address barriers, optimize VTEP in inpatients, and reduce HA‐VTE across the system. Prior efforts at UCSD illustrated single‐center improvement, with an increase in adequate VTEP from 50% to over 95%, and a nearly 40% reduction in the incidence of HA‐VTE.[12] We set out to scale this success across all 5 sites as a coordinated collaborative.
METHODS
This was a prospective, unblinded, open‐intervention study with historical controls that assessed prespecified outcomes before, during, and after institution of multiple VTEP strategies in 5 independent, but cooperating, academic hospitals. All adult medical and surgical inpatients were included; psychiatric, obstetricsgynecology, rehabilitation, observation status, and pediatric populations were excluded. The study period was July 1, 2012 through June 30, 2015. Calendar year (CY) 2011 was the baseline year for comparison; interventions were initiated in CY 2012 to CY 2014, and CY 2014 was considered the mature postintervention period.
Hospital Collaboration
Multiprofessional teams[1] were formed at each site. Monthly webinars, regular e‐mail, minutes, and a project management plan with task lists were utilized for coordinated collaboration. Software (Dropbox) was used for sharing tools, educational materials, and measurement techniques. REDCap (Research Electronic Data Capture) was used for secure data collection and analysis of outcomes.[13] Prior experience at UCSD and the Society of Hospital Medicine informed measurement and intervention bundle strategies.[1, 12, 14] Surveys of baseline VTE prevention protocols, measures, and order sets were performed at each site. Measures were standardized, whereas the intervention bundle was tailored for use at each medical center. Institutional review board approval with a waiver for individualized informed consent was obtained.
Interventions
All sites were tasked with implementing a defined bundle of mutually reinforcing interventions that constituted a comprehensive VTE prevention program. These protocols, order sets, educational programs, and interventions were not designed or implemented in an identical fashion at each hospital, but common principles were utilized.
VTE Prevention Protocol
This protocol incorporated (1) standardized VTE risk assessment, and (2) links to a menu of appropriate prophylaxis options for each level of risk that included guidance for management of patients with contraindications to pharmacologic prophylaxis. We used simple risk‐assessment models that grouped patients into 3 levels of risk (the 3‐bucket model) rather than more complicated point‐based systems. The 3‐bucket model was designed to offer detailed guidance and avoid over‐prophylaxis. Protocol, measurement, and order set tools were modified for special populations, such as orthopedic and neurosurgery populations. Operational definitions for bleeding risk, DVT risk, and exceptions to the protocol were explicit, which allowed for classification of adequate versus inadequate prophylaxis. High‐risk patients required combination prophylaxis, moderate risk anticoagulant prophylaxis, and low risk patients no prophylaxis beyond ambulation protocols (in the absence of contraindications). Acceptable contraindications to pharmacologic prophylaxis included an international normalized ratio >1.8, platelet count <50,000, active hemorrhage within the last 3 days, known bleeding disorders, hypertensive urgencies/emergencies, comfort careonly status, and leeway times around surgery or other events (24 hours for most surgeries, 48 hours for transplant surgery or major trauma, up to a week after central nervous system surgery). Impaired mobility was considered present unless the patient could ambulate independently more than once a day. More details regarding 3‐bucket risk models and explicit criteria can be reviewed in a recent Agency for Healthcare Quality and Research (AHRQ) publication.[1] The protocol was embedded into clinical decision‐support as required elements of admission, transfer, and postoperative order sets.
Educational Programs
Nurse and physician education programs were developed that stressed the importance of VTE prevention and adherence to thromboprophylaxis, including mechanical prophylaxis. The VTEP protocol was socialized in medical staff and nursing meetings. The educational programs recommended imaging only the proximal veins in patients with symptoms of leg DVT, and avoiding screening ultrasounds in asymptomatic patients. Physicians were coached on how to use the VTEP order sets. Content for educational programs was discussed and often shared among sites, but educational programs were tailored locally to fit perceived needs and available resources.
Measure‐vention
An active surveillance and feedback program called measure‐vention was developed to provide ongoing feedback to care providers regarding the appropriate use of VTEP over the duration of hospitalization. Key features of measure‐vention were regular measurement of adherence/lapses in VTEP delivery, coupled with concurrent intervention to correct any lapses, with a nurse/pharmacist calling the primary team if VTEP was suboptimal.[1, 12] Measure‐vention was utilized to monitor both appropriateness of orders and adherence with ordered prophylaxis, and was used to correct overprophylaxis as well as underprophylaxis. For example, our protocol specified that moderate VTE risk patients with a captured contraindication to anticoagulant should be on mechanical prophylaxis. An intervention would take place if mechanical prophylaxis was not ordered, or if it was ordered but not documented as being in place. Measure‐vention examples and further description are available in AHRQ publications.[1]
Outcomes
Thromboprophylaxis Rates
We planned to perform structured chart review on at least 30 noncritical care and 15 critical care adult inpatients per month at each site. Adult inpatients with a length of stay >48 hours, stratified by critical care versus noncritical care status, were assigned a numeric value by a random number generator. Patients were selected in order of random number assignment for chart review until the desired number of audits was completed. Development of the audit tools, as well as availability of personnel, led to delays in assessing prophylaxis rates by these standards until late 2012 to early 2013 at each site. A few sites had brief lapses in data collection during personnel changes. VTE risk, bleeding risk, prophylaxis ordered at the time of the audit, and adequacy of VTEP defined by a common standard were all assessed and recorded in the REDCap data repository. VTEP was considered adequate if combined pharmacologic and mechanical prophylaxis was present in the highest‐risk patients or anticoagulant prophylaxis was present in moderate patients. Prophylaxis was considered adequate for all low‐risk patients. Patients at risk for VTE with contraindications to anticoagulants were considered to be on adequate prophylaxis if they received mechanical prophylaxis or had documented contraindications to mechanical prophylaxis. The proper administration of ordered prophylaxis was scrutinized locally and targeted by education and other interventions at each site, but these data were not collated and analyzed centrally.
Identification of HA‐VTE
HA‐VTE rates were determined by administrative coding data, using International Classification of Diseases, Ninth Revision, Clinical Modification (ICD‐9‐CM) codes in a manner similar to AHRQ Patient Safety Indicator 12 identification of postoperative VTE cases.[10] Data were submitted by each hospital, then collated and analyzed using data from Vizient (formerly the University HealthSystem Consortium). The incidence of VTE was determined using specific ICD‐9‐CM hospital discharge codes: for PE: 415.11, 415.13, 415.19, 673.24; and for DVT: proximal DVT: 451.11, 451.19, 451.81, 453.41; distal DVT: 453.42; and other DVT: 453.40, 453.8. These codes have high positive predictive value for acute VTE.[15, 16] Mean age, average length of stay (ALOS), and admission severity of illness (SOI) scores were also captured from Vizient and summarized for the inpatient cohort each year.
All VTE cases were coupled with present on admission (POA) indicators. HA‐VTE cases included patients who were readmitted to the same hospital within 30 days for a new event (POA = Y, but readmitted), as well as patients who developed PE or DVT during their hospitalization (POA = N or U). Only patients hospitalized for 3 or more days were analyzed for inpatient development of VTE, as diagnosis of VTE in the first 2 days was deemed either likely present on admission or not preventable using VTEP started within 24 hours of admission. VTE outcomes were assigned in a hierarchical fashion: if both PE and DVT were present, the case was classified as PE. Distal DVT was distinguished from proximal DVT whenever possible. Cases were stratified based on whether the patient had undergone a major operation (surgery patients) or not (medical patients). This stratification was based on the Medicare Severity Diagnosis‐Related Group (MS‐DRG) coded in patient records. The DRG type for each MS‐DRG was based on the 2015 CMS‐MS‐DRG codes for major operations,[9] except that all trauma cases were considered surgical, and cases with vena cava filter placement and no other surgical procedure were considered medical. Cancer cases were identified using ICD‐9‐CM codes 140.00‐209.99 and 210.00‐239.99.
Review of HA‐VTE
Periodic review of selected HA‐VTE cases identified by administrative coding data was recommended as a best practice, potentially adding insight to contributing factors to HA‐VTE, included lapses in prophylaxis and suboptimal mobilization. The accuracy of diagnostic coding, and assessment of how HA‐VTE cases were identified (symptoms vs screening ultrasounds) could also be assessed. Examples of audit tools were shared. Every site reviewed some HA‐VTE cases, but the extent and duration of case review was left to the discretion of each site.
Statistical Analysis
Relative risk (RR) calculations with 95% confidence intervals (CI) were used to compare the proportions of patients with PE, DVT alone, and total HA‐VTE in 2014 versus 2011. The absolute risk reduction was multiplied by the population at risk in CY 2014 to arrive at estimates of cases of VTE averted in 2014 compared to 2011.
RESULTS
Robust sampling (421 to 728 patients at each site) revealed attainment of high rates of adequate VTE prophylaxis (82% to 96% at all sites, collectively 89%) by early 2014. Common measures for adequate VTEP were not finalized and collected by all sites until early 2013, so we did not capture baseline VTEP rates, and could not compare baseline to mature prophylaxis rates. Reliable administration of mechanical and anticoagulant prophylaxis was monitored and targeted by each institution, albeit not in an identical fashion at each site. Adherence to mechanical prophylaxis was reported as improved at the sites, but these data were not collated and analyzed centrally.
Population Demographics and Severity of Illness
There were 73,941 to 79,565 discharges that met the criteria (adult medicalsurgical inpatient with >2 day length of stay each year. Mean age and ALOS were unchanged or had no change of clinical significance. For example, in 2011 versus 2014, mean age was 55.7 versus 56.4 years, and ALOS was identical in both time periods at 7.4 days. Admission SOI scores also remained fairly static from 2011 to 2014 (2.27, 2.31, 2.32, 2.26, respectively), and the admission SOI was not statistically different in 2011 versus 2014 (estimated difference of 2 means 0.01, 95% CI: 0.00‐0.02).
Hospital‐Associated VTE
There were 2431 HA‐VTE events observed in 306,906 adult inpatients across CY 2011 to 2014 (Table 1). The baseline incidence of HA‐VTE was 0.90% (667 events in 73,941 hospitalizations in 2011). The incidence of HA‐VTE in the postintervention period was 0.69% (546 HA‐VTE events in 79,565 hospitalizations in 2014, P < 0.001), an overall reduction of 24%. The absolute risk for PE decreased from 0.49% to 0.39% (RR: 0.79, 95% CI: 0.68‐0.92), a reduction of 21%, and the absolute risk of leg DVT fell from 0.41% to 0.30% (RR: 0.73, 95% CI: 0.61‐0.86), a reduction of 27%. Both proximal and distal DVT were reduced significantly. Proximal DVT was much more commonly diagnosed than distal DVT. Proximal DVT incidence decreased from 0.32% to 0.25% (RR: 0.77, 95% CI: 0.64‐0.93), whereas distal DVT incidence decreased from 0.09% to 0.05% (RR: 0.58, 95% CI: 0.39‐0.86). The lower overall VTE rate in the postimplementation period compared with the baseline period corresponds to an estimated 170 fewer cases of VTE per year (89 DVT, 81 PE).
2011 (Baseline), No./% | 2012, No./% | 2013, No./% | 2014 (Mature), No./% | 2014 Versus 2011 Relative Risk (95% CI) | 2014 Versus 2011 Estimated Averted Events (95% CI) | |
---|---|---|---|---|---|---|
| ||||||
Total discharges (medical and surgical) | 73,941 | 76,100 | 77,300 | 79,565 | ||
Total PE + leg DVT | 667/0.90% | 650/0.85% | 568/0.73% | 546/0.69% | 0.761 (0.680‐0.852) | 170 (103‐247) |
Total PE | 363/0.49% | 359/0.47% | 340/0.44% | 309/0.39% | 0.791 (0.680‐0.920) | 81 (32‐135) |
Total leg DVT | 304/0.41% | 291/0.38% | 228/0.29% | 237/0.3% | 0.725 (0.612‐0.858) | 89(40‐135) |
Medical discharges | 31,219 | 32,597 | 33,805 | 34,875 | ||
Total PE + leg DVT | 178/0.57% | 168/0.52% | 164/0.49% | 179/0.51% | 0.900 (0.732‐1.1071) | |
PE | 110/0.35% | 94/0.29% | 106/0.31% | 104/0.30% | 0.846 (0.648‐1.106) | |
Leg DVT | 68/0.22% | 74/0.23% | 58/0.17% | 75/0.22% | 0.987 (0.711‐1.371) | |
Surgical discharges | 42,722 | 43,503 | 43,495 | 44,690 | ||
Total PE + leg DVT | 489/1.14% | 482/1.11% | 404/0.93% | 367/0.82% | 0.718 (0.627‐0.821) | |
PE | 253/0.59% | 265/0.61% | 234/0.54% | 205/0.46% | 0.775 (0.645‐0.931) | |
Leg DVT | 236/0.55% | 217/0.50% | 180/0.41% | 162/0.36% | 0.656 (0.538‐0.801) |
The baseline rate of HA‐VTE and degree of improvement varied between institutions (Figure 1). UCI and UCD began the study with significantly higher VTE rates, and enjoyed the largest improvements. UCLA's VTE rate decreased to a lesser extent, whereas UCSD and UCSF rates remained relatively flat or were marginally higher. In contrast to the highly variable 2011 baseline rate of HA‐VTE (0.60%1.36%), all 5 sites had HA‐VTE rates within a very narrow range (0.65%0.73%) at maturity in 2014.
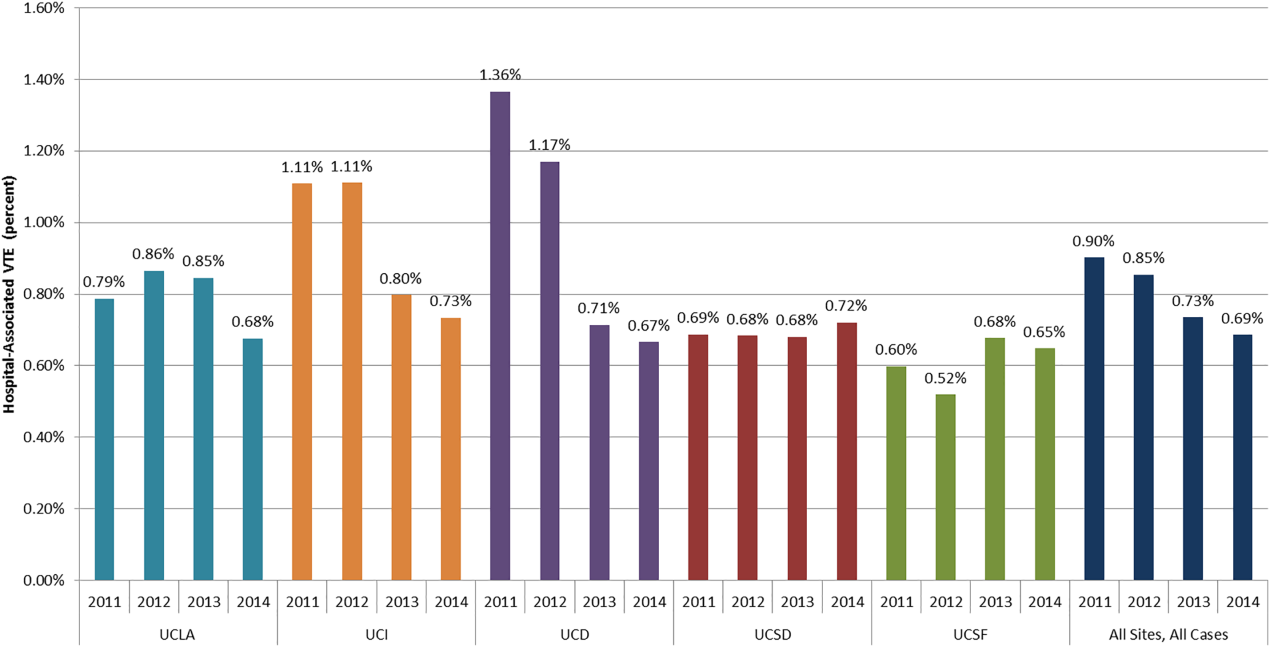
Cancer Versus Noncancer Patients
The incidence of HA‐VTE was higher in cancer patients than in noncancer patients. In 2011, 227 of 18,487 (1.23%) cancer patients developed VTE, versus 440 of 55,454 (0.79%) noncancer patients (Figure 2). After implementation of the VTE prevention initiative, the incidence of VTE in cancer patients fell by 0.21% (210 events in 20,544 patients in 2014, 1.02%), and the incidence of VTE in noncancer patients fell by 0.22% (336 events in 59,021 patients, 0.57%). The relative risk of HA‐VTE after the VTE interventions was reduced by 17% (RR: 0.83, 95% CI: 0.69‐1.00) in cancer patients and 28% (RR: 0.72, 95% CI: 0.62‐0.83) in noncancer patients.
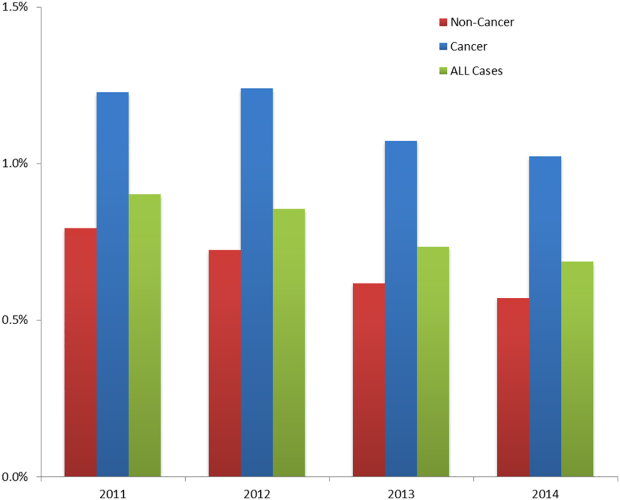
Surgical Versus Medical Patients
The impact of the VTE prevention initiative was only significant in surgical patients, for whom the risk of HA‐VTE fell by 28% (RR: 0.72, 95% CI: 0.63‐0.82) (Table 1). Medical patients experienced a nonsignificant 10% reduction in HA‐VTE (RR: 0.90, 95% CI: 0.73‐1.11). Medical patients had a significantly lower baseline incidence of HA‐VTE (0.57%) compared with surgical patients (1.14%; relative difference: 50%, P < 0.001). This finding persisted postimplementation, with a cumulative incidence in medical patients of 0.51% versus 0.82% in surgical patients (relative difference: 31%, P < 0.001).
DISCUSSION
Our initiative, comprised of a collaborative infrastructure, a proven quality‐improvement framework, and a bundle of interventions, was associated with a 24% reduction in the risk of HA‐VTE across our 5 academic medical centers. This represents avoidance of significant clinical morbidity (an estimated 81 PEs and 89 DVTs per year) and significant cost. Assuming costs of $9250 per DVT and $13,050 per PE,[17] the estimated short‐term cost savings are almost $1.9 million per year (minus expenditures on VTEP). Further savings might be expected over a longer time horizon because of the avoidance of recurrent VTE, post‐thrombotic syndrome, and the costs and complications of long‐term anticoagulation.
We believe the highly variable degree of improvement seen across our 5 sites was due to the relatively mature VTEP efforts at the onset of this collaborative improvement effort at UCSD and UCSF. As we noted earlier, the interventional bundle and methods were derived from earlier work at UCSD that had already demonstrated published marked improvement in prophylaxis and a 40% decrease in HA‐VTE.[14] The narrow range of low HA‐VTE rates in 2014 (the mature intervention time period) suggests there may be some HA‐VTE rate beyond which further prevention efforts are less productive.
Our study has several limitations. As a longitudinal collaborative improvement effort introducing a bundle of interventions, we cannot ascribe improved outcomes to individual components in the bundle; for example, we did not record the number of measure‐vention calls or resulting prophylaxis changes. We also did not measure adverse events due to VTEP, believing benefits to be greater than risks, but some adverse events likely did occur and attenuated benefits and cost savings. Although we had rigorous measures to assess the prevalence of appropriate prophylaxis, we failed to capture the baseline rate of VTEP, which means we cannot show that improved HA‐VTE rates corresponded to improvements in VTEP rates. The bundle of interventions was not implemented uniformly. Some metrics, like adherence to mechanical prophylaxis, were monitored in a decentralized fashion, without collation or collective analysis.
Were improved VTE rates due to decreases in HA‐VTE detection? We could not detect postdischarge HA‐VTE that presented to other hospitals, but we have no reason to think the proportion of missed HA‐VTE changed over the study. We discouraged the practice of routinely extending duplex ultrasound testing below the knee, and also discouraged surveillance of asymptomatic patients with Doppler ultrasound. This raises the question of ascertainment bias. Did we have fewer HA‐VTE in 2014 because our interventions worked, or did we reduce how aggressively we looked for HA‐VTE? Higher frequencies of ultrasound testing are correlated with higher rates of DVT because of surveillance bias.[18] Although some reduction in DVT was due to changes in ultrasound practices, several factors suggest the majority of improvement resulted from our interventions. First, only 1 of our 5 sites (UCD) routinely extended ultrasound testing below the knee in the baseline period. Second, we distinguished distal DVT from proximal/unspecified DVT, and the rates of both showed significant improvement. Screening asymptomatic patients with ultrasounds for DVT was limited to a few services in special circumstances (for example, the trauma service at UCSD screened patients at highest risk who could not be prophylaxed with anticoagulation). We did not have the capability to formally track which patients were being diagnosed with screening exams versus for symptoms, but screen‐detected patients were a small minority. We did not successfully dissuade these few services from stopping this approach, but we did head off some services that were considering this strategy, and think it likely that at best, we kept screening from spreading. Third, PE was reduced by over 20%, in addition to reductions in DVT, even though several of our sites acquired computed tomography scanners more sensitive for small thrombi/emncidental PE. Finally, the aggressiveness of ultrasound testing often goes up with aggressive prevention efforts, which would have led to surveillance bias with increasedrather than decreasedrates of HA‐VTE.
Our study has a number of strengths. Our effort encompassed a large and inclusive adult inpatient population over a long period of observation, with a relatively large reduction in HA‐VTE. These reductions occurred even though the proportion of patients with cancer (our most powerful predictor of VTE risk) was 34.8% in 2014 versus 33.3% in 2011. Our metrics captured patients readmitted to the hospital within 30 days of a prior VTE‐free admission as well as patients suffering VTE during the hospital stay, with the limitation that we captured only patients readmitted back to our own institutions. Our metrics for VTEP scrutinized prophylaxis rates at different points during hospitalizations, and risk‐appropriate prophylaxis was assessed, in contrast to some common regulatory measures that monitor only whether any prophylaxis is in place on the first day of admission or transfer.[11]
Our study should be instructive in terms of focusing improvement efforts. The rate of HA‐VTE was much higher in cancer and surgical patients than in medical patients, and we only achieved a nonsignificant 10% reduction in risk among medical patients (RR: 0.90, 95% CI: 0.73‐1.11). This is consistent with literature demonstrating a more limited benefit of prophylaxis in medical inpatients.[19] Although we continue to recommend prophylaxis in high‐risk medical inpatients, efforts targeting cancer and surgical populations are likely to yield greater results.
Our collaborative used methods that are portable, sustainable, and provide an excellent platform for spread of improvement across a system. The portability of these strategies is underlined by the variable baseline performance and the different stages of electronic health record development at our unique sites. Toolkits that describe the interventions (such as order sets, educational tools, measures, measure‐vention) are freely available, and reflect established guidelines.[1] Our collaborative model is consistent with successful models published in the literature.[1, 14, 20] In these models, clinical experts distill the evidence down into key best practices, and design processes that need to occur with the lowest barriers to use. Metrics, expert advice, and toolkits are assembled centrally, while each hospital identifies local barriers to implementation, educates and engages staff, executes implementation, and continually evaluates performance, modifying interventions accordingly. Embedding clinical decision and risk‐assessment into VTE prevention modules within commonly used order sets and documentation tools helps to hard‐wire the interventions, tightly linking risk assessment to appropriate prophylaxis options. The approach to standardization allows for flexibility for special populations and special needs of unique patients, while minimizing needless variation based on the ordering providers. Program management tools and regular webinars keeps sites on track, coordinate interventions, sustain enthusiasm, and provide a venue for sharing tools and lessons learned. Multiple active interventions are utilized rather than relying on passive educational techniques or order sets alone. Active surveillance (i.e., measure‐vention) deserves special attention. Measure‐vention has demonstrated utility in inpatient glycemic control and a variety of hospital‐associated infections in addition to VTE prevention, and some systems now uses measure‐ventionists as the lynchpin for a whole host of successful improvement programs.[12, 14, 21, 22] We believe high‐quality metrics, standardized protocol‐driven order sets, and measure‐vention are the crucial elements for success.
CONCLUSIONS
Hospital systems can reduce HA‐VTE by implementing a bundle of active interventions including standardized VTEP orders with embedded risk assessment and measure‐vention. Good measurement of HA‐VTE, appropriate VTEP that exceeds minimum regulatory standards, and a robust collaborative infrastructure inform and accelerate improvement. Surgical and cancer populations are at higher risk for HA‐VTE and should be a prime focus of improvement efforts.
Disclosures
Ian H Jenkins: nothing to report. Alpesh N. Amin: nothing to report. Nasim Afsarmanesh: nothing to report. Dr. Auerbach receives honorarium as Editor‐in‐Chief of the Journal of Hospital Medicine. Dr. Khanna has licensed technology to the hospital‐based electronic messaging vendor Voalte and will benefit financially from its dissemination. This does not impact this work. Dr. Maynard acts as a consultant on an expert panel overseeing a multinational trial of extended VTE prophylaxis in high‐risk medical patients (Medically Ill Patient Assessment of Rivaroxaban Versus Placebo in Reducing Post‐Discharge Venous Thrombo‐Embolism Risk), a study funded by Johnson & Johnson. Dr. White has acted as a consultant for Janssen, Boehringer‐Ingleheim, Diiachi‐Sankyo, and Bristol Meyer Squibb, and provides expert testimony for various malpractice defense lawyers for VTE, and has a grant with the Gordon and Betty Moore Foundation regarding VTE prevention.
- Preventing Hospital‐Associated Venous Thromboembolism: A Guide for Effective Quality Improvement. 2nd ed. Rockville, MD: Agency for Healthcare Research and Quality; October 2015. AHRQ Publication No. 16–001‐EF. Available at: http://www.ahrq.gov/professionals/quality‐patient‐safety/patient‐safety‐resources/resources/vtguide/index.html. Accessed June 1, 2016. .
- Relative impact of risk factors for deep vein thrombosis and pulmonary embolism. Arch Intern Med. 2002;162:1245–1248. , , , et al.
- Antithrombotic therapy practices in US hospitals in an era of practice guidelines. Arch Intern Med. 2005;165:1458–1464. , , , et al.
- Prevention of VTE in nonsurgical patients. Chest. 2012;141(2 suppl):e195S–e226S. , , , et al.
- Prevention of VTE in nonorthopedic surgical patients. Chest. 2012;141(2 suppl):e227S–e277S. , , , et al.
- Prevention of VTE in orthopedic surgery patients. Chest. 2012;141(2 suppl):e278S–e325S. , , , et al.
- The outcome after treatment of venous thromboembolism is different in surgical and acutely ill medical patients. Findings from the RIETE registry. J Thromb Haemost. 2004;2:1892–1898. , , , et al.
- Inpatient thromboprophylaxis use in U.S. hospitals: adherence to the Seventh American College of Chest Physician's recommendations for at‐risk medical and surgical patients. J Hosp Med. 2009;4:E15–E21. , , , .
- Centers for Medicare 5(1):10–18.
- Research electronic data capture (REDCap)—a metadata‐driven methodology and workflow process for providing translational research informatics support. J Biomed Inform. 2009;42:377–381. , , , et al.
- Mentored implementation: building leaders and achieving results through a collaborative improvement model. 2011 John M. Eisenberg Patient Safety and Quality Award, National Level. Jt Comm J Qual Patient Saf. 2012;38(7):301–310. , , , et al.
- Predictive value of the POA indicator for hospital‐acquired venous thromboembolism. Med Care. 2013:53(4):e31–e36. , , , et al.
- Improved coding of postoperative deep vein thrombosis and pulmonary embolism in administrative data (AHRQ patient safety indicator 12) after introduction of new ICD‐9‐CM diagnosis codes. Med Care. 2015:53(5):e37–e40. , , , et al.
- Economic burden of venous thromboembolism in hospitalized patients. Pharmacotherapy. 2009;29(8):943–953. .
- Evaluation of surveillance bias and the validity of the venous thromboembolism quality measure. JAMA. 2013;310(14):1482–1489. , , , et al.
- Venous thromboembolism prophylaxis in hospitalized medical patients and those with stroke: a background review for an American College of Physicians clinical practice guideline. Ann Intern Med. 2011;155(9):602–615. , , , et al.
- Translating evidence into practice: a model for large scale knowledge translation. BMJ. 2008; 337:a1714. , , .
- Impact of a hypoglycemia reduction bundle and a systems approach to inpatient glycemic management. Endocr Pract. 2015;21(4):355–367. , , , et al.
- Zero adverse events: how Dignity Health achieved a new standard. Becker's Hospital Review: Infection Control and Clinical Quality website. Available at: http://www.beckershospitalreview.com/quality/zero‐adverse‐events‐how‐dignity‐health‐achieved‐a‐new‐standard.html. Accessed April 19, 2016. .
Venous thromboembolism (VTE), comprised of pulmonary embolism (PE) and deep vein thrombosis (DVT), impacts hundreds of thousands of Americans annually.[1] The complications of VTE can be severe, including the post‐thrombotic syndrome, pulmonary hypertension, and complications of anticoagulation. VTE is often a complication of hospitalization, and PE is a common preventable cause of hospital mortality.[2, 3] Pharmacologic VTE prophylaxis (VTEP) in at‐risk patients is effective and endorsed by prominent guidelines.[4, 5, 6] However, VTEP is underutilized, with only 30% to 50% of eligible patients receiving the right drug, dose, and duration.[7, 8]
Public reporting and reimbursement policies reflect the magnitude of VTE as a public health concern. The Centers for Medicare and Medicaid Services (CMS) withholds incremental payment for VTE complications.[9] The rate of hospital‐associated VTE (HA‐VTE) is used by benchmarking organizations as a quality indicator.[10, 11]
The University of California (UC) has 5 major academic medical centers, located in Irvine (UCI), Los Angeles (UCLA), Sacramento (UC Davis [UCD]), San Diego (UCSD), and San Francisco (UCSF). In both 2010 and 2011, almost 700 UC patients suffered from HA‐VTE annually. Barriers to optimal VTEP included the absence of standardized VTE risk assessment, lack of consensus on appropriate VTEP options for various inpatient populations, and a lack of collaborative infrastructure. Other barriers included poor adherence to mechanical prophylaxis and suboptimal measurement of prophylaxis and HA‐VTE outcomes.
In late 2011, leaders from the 5 medical centers, supported by an internal competitive grant from the UC Office of the President and the Center for Health Quality and Innovation, formed a collaborative to address barriers, optimize VTEP in inpatients, and reduce HA‐VTE across the system. Prior efforts at UCSD illustrated single‐center improvement, with an increase in adequate VTEP from 50% to over 95%, and a nearly 40% reduction in the incidence of HA‐VTE.[12] We set out to scale this success across all 5 sites as a coordinated collaborative.
METHODS
This was a prospective, unblinded, open‐intervention study with historical controls that assessed prespecified outcomes before, during, and after institution of multiple VTEP strategies in 5 independent, but cooperating, academic hospitals. All adult medical and surgical inpatients were included; psychiatric, obstetricsgynecology, rehabilitation, observation status, and pediatric populations were excluded. The study period was July 1, 2012 through June 30, 2015. Calendar year (CY) 2011 was the baseline year for comparison; interventions were initiated in CY 2012 to CY 2014, and CY 2014 was considered the mature postintervention period.
Hospital Collaboration
Multiprofessional teams[1] were formed at each site. Monthly webinars, regular e‐mail, minutes, and a project management plan with task lists were utilized for coordinated collaboration. Software (Dropbox) was used for sharing tools, educational materials, and measurement techniques. REDCap (Research Electronic Data Capture) was used for secure data collection and analysis of outcomes.[13] Prior experience at UCSD and the Society of Hospital Medicine informed measurement and intervention bundle strategies.[1, 12, 14] Surveys of baseline VTE prevention protocols, measures, and order sets were performed at each site. Measures were standardized, whereas the intervention bundle was tailored for use at each medical center. Institutional review board approval with a waiver for individualized informed consent was obtained.
Interventions
All sites were tasked with implementing a defined bundle of mutually reinforcing interventions that constituted a comprehensive VTE prevention program. These protocols, order sets, educational programs, and interventions were not designed or implemented in an identical fashion at each hospital, but common principles were utilized.
VTE Prevention Protocol
This protocol incorporated (1) standardized VTE risk assessment, and (2) links to a menu of appropriate prophylaxis options for each level of risk that included guidance for management of patients with contraindications to pharmacologic prophylaxis. We used simple risk‐assessment models that grouped patients into 3 levels of risk (the 3‐bucket model) rather than more complicated point‐based systems. The 3‐bucket model was designed to offer detailed guidance and avoid over‐prophylaxis. Protocol, measurement, and order set tools were modified for special populations, such as orthopedic and neurosurgery populations. Operational definitions for bleeding risk, DVT risk, and exceptions to the protocol were explicit, which allowed for classification of adequate versus inadequate prophylaxis. High‐risk patients required combination prophylaxis, moderate risk anticoagulant prophylaxis, and low risk patients no prophylaxis beyond ambulation protocols (in the absence of contraindications). Acceptable contraindications to pharmacologic prophylaxis included an international normalized ratio >1.8, platelet count <50,000, active hemorrhage within the last 3 days, known bleeding disorders, hypertensive urgencies/emergencies, comfort careonly status, and leeway times around surgery or other events (24 hours for most surgeries, 48 hours for transplant surgery or major trauma, up to a week after central nervous system surgery). Impaired mobility was considered present unless the patient could ambulate independently more than once a day. More details regarding 3‐bucket risk models and explicit criteria can be reviewed in a recent Agency for Healthcare Quality and Research (AHRQ) publication.[1] The protocol was embedded into clinical decision‐support as required elements of admission, transfer, and postoperative order sets.
Educational Programs
Nurse and physician education programs were developed that stressed the importance of VTE prevention and adherence to thromboprophylaxis, including mechanical prophylaxis. The VTEP protocol was socialized in medical staff and nursing meetings. The educational programs recommended imaging only the proximal veins in patients with symptoms of leg DVT, and avoiding screening ultrasounds in asymptomatic patients. Physicians were coached on how to use the VTEP order sets. Content for educational programs was discussed and often shared among sites, but educational programs were tailored locally to fit perceived needs and available resources.
Measure‐vention
An active surveillance and feedback program called measure‐vention was developed to provide ongoing feedback to care providers regarding the appropriate use of VTEP over the duration of hospitalization. Key features of measure‐vention were regular measurement of adherence/lapses in VTEP delivery, coupled with concurrent intervention to correct any lapses, with a nurse/pharmacist calling the primary team if VTEP was suboptimal.[1, 12] Measure‐vention was utilized to monitor both appropriateness of orders and adherence with ordered prophylaxis, and was used to correct overprophylaxis as well as underprophylaxis. For example, our protocol specified that moderate VTE risk patients with a captured contraindication to anticoagulant should be on mechanical prophylaxis. An intervention would take place if mechanical prophylaxis was not ordered, or if it was ordered but not documented as being in place. Measure‐vention examples and further description are available in AHRQ publications.[1]
Outcomes
Thromboprophylaxis Rates
We planned to perform structured chart review on at least 30 noncritical care and 15 critical care adult inpatients per month at each site. Adult inpatients with a length of stay >48 hours, stratified by critical care versus noncritical care status, were assigned a numeric value by a random number generator. Patients were selected in order of random number assignment for chart review until the desired number of audits was completed. Development of the audit tools, as well as availability of personnel, led to delays in assessing prophylaxis rates by these standards until late 2012 to early 2013 at each site. A few sites had brief lapses in data collection during personnel changes. VTE risk, bleeding risk, prophylaxis ordered at the time of the audit, and adequacy of VTEP defined by a common standard were all assessed and recorded in the REDCap data repository. VTEP was considered adequate if combined pharmacologic and mechanical prophylaxis was present in the highest‐risk patients or anticoagulant prophylaxis was present in moderate patients. Prophylaxis was considered adequate for all low‐risk patients. Patients at risk for VTE with contraindications to anticoagulants were considered to be on adequate prophylaxis if they received mechanical prophylaxis or had documented contraindications to mechanical prophylaxis. The proper administration of ordered prophylaxis was scrutinized locally and targeted by education and other interventions at each site, but these data were not collated and analyzed centrally.
Identification of HA‐VTE
HA‐VTE rates were determined by administrative coding data, using International Classification of Diseases, Ninth Revision, Clinical Modification (ICD‐9‐CM) codes in a manner similar to AHRQ Patient Safety Indicator 12 identification of postoperative VTE cases.[10] Data were submitted by each hospital, then collated and analyzed using data from Vizient (formerly the University HealthSystem Consortium). The incidence of VTE was determined using specific ICD‐9‐CM hospital discharge codes: for PE: 415.11, 415.13, 415.19, 673.24; and for DVT: proximal DVT: 451.11, 451.19, 451.81, 453.41; distal DVT: 453.42; and other DVT: 453.40, 453.8. These codes have high positive predictive value for acute VTE.[15, 16] Mean age, average length of stay (ALOS), and admission severity of illness (SOI) scores were also captured from Vizient and summarized for the inpatient cohort each year.
All VTE cases were coupled with present on admission (POA) indicators. HA‐VTE cases included patients who were readmitted to the same hospital within 30 days for a new event (POA = Y, but readmitted), as well as patients who developed PE or DVT during their hospitalization (POA = N or U). Only patients hospitalized for 3 or more days were analyzed for inpatient development of VTE, as diagnosis of VTE in the first 2 days was deemed either likely present on admission or not preventable using VTEP started within 24 hours of admission. VTE outcomes were assigned in a hierarchical fashion: if both PE and DVT were present, the case was classified as PE. Distal DVT was distinguished from proximal DVT whenever possible. Cases were stratified based on whether the patient had undergone a major operation (surgery patients) or not (medical patients). This stratification was based on the Medicare Severity Diagnosis‐Related Group (MS‐DRG) coded in patient records. The DRG type for each MS‐DRG was based on the 2015 CMS‐MS‐DRG codes for major operations,[9] except that all trauma cases were considered surgical, and cases with vena cava filter placement and no other surgical procedure were considered medical. Cancer cases were identified using ICD‐9‐CM codes 140.00‐209.99 and 210.00‐239.99.
Review of HA‐VTE
Periodic review of selected HA‐VTE cases identified by administrative coding data was recommended as a best practice, potentially adding insight to contributing factors to HA‐VTE, included lapses in prophylaxis and suboptimal mobilization. The accuracy of diagnostic coding, and assessment of how HA‐VTE cases were identified (symptoms vs screening ultrasounds) could also be assessed. Examples of audit tools were shared. Every site reviewed some HA‐VTE cases, but the extent and duration of case review was left to the discretion of each site.
Statistical Analysis
Relative risk (RR) calculations with 95% confidence intervals (CI) were used to compare the proportions of patients with PE, DVT alone, and total HA‐VTE in 2014 versus 2011. The absolute risk reduction was multiplied by the population at risk in CY 2014 to arrive at estimates of cases of VTE averted in 2014 compared to 2011.
RESULTS
Robust sampling (421 to 728 patients at each site) revealed attainment of high rates of adequate VTE prophylaxis (82% to 96% at all sites, collectively 89%) by early 2014. Common measures for adequate VTEP were not finalized and collected by all sites until early 2013, so we did not capture baseline VTEP rates, and could not compare baseline to mature prophylaxis rates. Reliable administration of mechanical and anticoagulant prophylaxis was monitored and targeted by each institution, albeit not in an identical fashion at each site. Adherence to mechanical prophylaxis was reported as improved at the sites, but these data were not collated and analyzed centrally.
Population Demographics and Severity of Illness
There were 73,941 to 79,565 discharges that met the criteria (adult medicalsurgical inpatient with >2 day length of stay each year. Mean age and ALOS were unchanged or had no change of clinical significance. For example, in 2011 versus 2014, mean age was 55.7 versus 56.4 years, and ALOS was identical in both time periods at 7.4 days. Admission SOI scores also remained fairly static from 2011 to 2014 (2.27, 2.31, 2.32, 2.26, respectively), and the admission SOI was not statistically different in 2011 versus 2014 (estimated difference of 2 means 0.01, 95% CI: 0.00‐0.02).
Hospital‐Associated VTE
There were 2431 HA‐VTE events observed in 306,906 adult inpatients across CY 2011 to 2014 (Table 1). The baseline incidence of HA‐VTE was 0.90% (667 events in 73,941 hospitalizations in 2011). The incidence of HA‐VTE in the postintervention period was 0.69% (546 HA‐VTE events in 79,565 hospitalizations in 2014, P < 0.001), an overall reduction of 24%. The absolute risk for PE decreased from 0.49% to 0.39% (RR: 0.79, 95% CI: 0.68‐0.92), a reduction of 21%, and the absolute risk of leg DVT fell from 0.41% to 0.30% (RR: 0.73, 95% CI: 0.61‐0.86), a reduction of 27%. Both proximal and distal DVT were reduced significantly. Proximal DVT was much more commonly diagnosed than distal DVT. Proximal DVT incidence decreased from 0.32% to 0.25% (RR: 0.77, 95% CI: 0.64‐0.93), whereas distal DVT incidence decreased from 0.09% to 0.05% (RR: 0.58, 95% CI: 0.39‐0.86). The lower overall VTE rate in the postimplementation period compared with the baseline period corresponds to an estimated 170 fewer cases of VTE per year (89 DVT, 81 PE).
2011 (Baseline), No./% | 2012, No./% | 2013, No./% | 2014 (Mature), No./% | 2014 Versus 2011 Relative Risk (95% CI) | 2014 Versus 2011 Estimated Averted Events (95% CI) | |
---|---|---|---|---|---|---|
| ||||||
Total discharges (medical and surgical) | 73,941 | 76,100 | 77,300 | 79,565 | ||
Total PE + leg DVT | 667/0.90% | 650/0.85% | 568/0.73% | 546/0.69% | 0.761 (0.680‐0.852) | 170 (103‐247) |
Total PE | 363/0.49% | 359/0.47% | 340/0.44% | 309/0.39% | 0.791 (0.680‐0.920) | 81 (32‐135) |
Total leg DVT | 304/0.41% | 291/0.38% | 228/0.29% | 237/0.3% | 0.725 (0.612‐0.858) | 89(40‐135) |
Medical discharges | 31,219 | 32,597 | 33,805 | 34,875 | ||
Total PE + leg DVT | 178/0.57% | 168/0.52% | 164/0.49% | 179/0.51% | 0.900 (0.732‐1.1071) | |
PE | 110/0.35% | 94/0.29% | 106/0.31% | 104/0.30% | 0.846 (0.648‐1.106) | |
Leg DVT | 68/0.22% | 74/0.23% | 58/0.17% | 75/0.22% | 0.987 (0.711‐1.371) | |
Surgical discharges | 42,722 | 43,503 | 43,495 | 44,690 | ||
Total PE + leg DVT | 489/1.14% | 482/1.11% | 404/0.93% | 367/0.82% | 0.718 (0.627‐0.821) | |
PE | 253/0.59% | 265/0.61% | 234/0.54% | 205/0.46% | 0.775 (0.645‐0.931) | |
Leg DVT | 236/0.55% | 217/0.50% | 180/0.41% | 162/0.36% | 0.656 (0.538‐0.801) |
The baseline rate of HA‐VTE and degree of improvement varied between institutions (Figure 1). UCI and UCD began the study with significantly higher VTE rates, and enjoyed the largest improvements. UCLA's VTE rate decreased to a lesser extent, whereas UCSD and UCSF rates remained relatively flat or were marginally higher. In contrast to the highly variable 2011 baseline rate of HA‐VTE (0.60%1.36%), all 5 sites had HA‐VTE rates within a very narrow range (0.65%0.73%) at maturity in 2014.
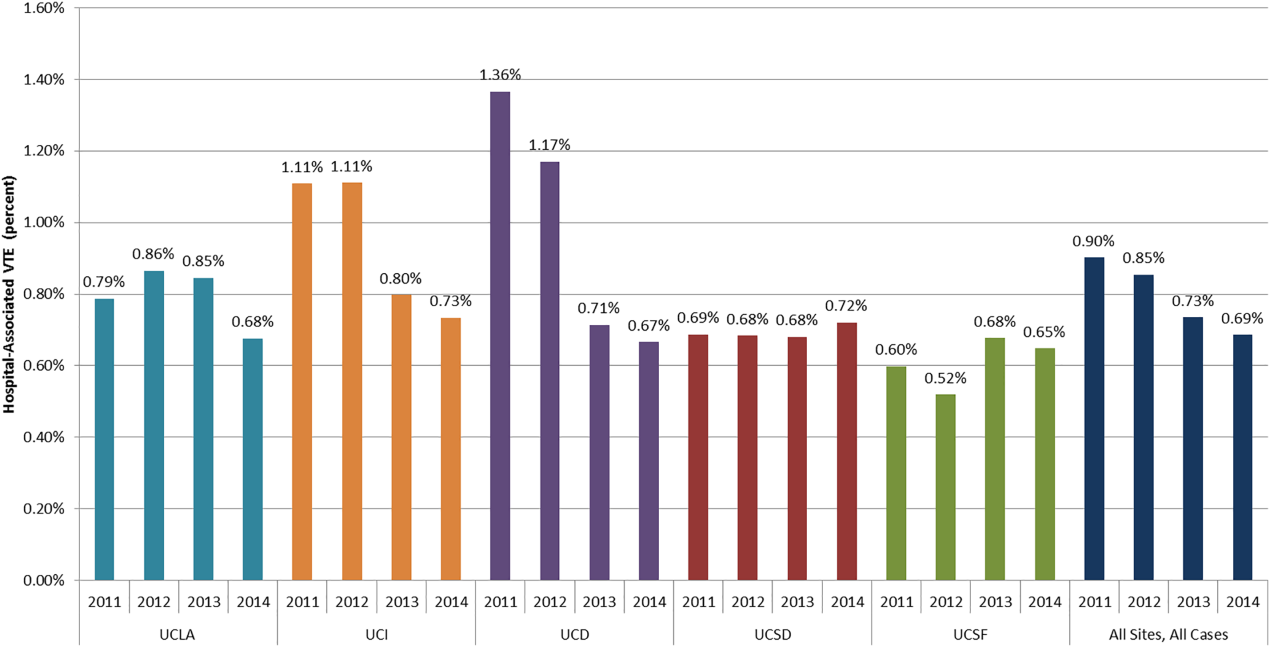
Cancer Versus Noncancer Patients
The incidence of HA‐VTE was higher in cancer patients than in noncancer patients. In 2011, 227 of 18,487 (1.23%) cancer patients developed VTE, versus 440 of 55,454 (0.79%) noncancer patients (Figure 2). After implementation of the VTE prevention initiative, the incidence of VTE in cancer patients fell by 0.21% (210 events in 20,544 patients in 2014, 1.02%), and the incidence of VTE in noncancer patients fell by 0.22% (336 events in 59,021 patients, 0.57%). The relative risk of HA‐VTE after the VTE interventions was reduced by 17% (RR: 0.83, 95% CI: 0.69‐1.00) in cancer patients and 28% (RR: 0.72, 95% CI: 0.62‐0.83) in noncancer patients.
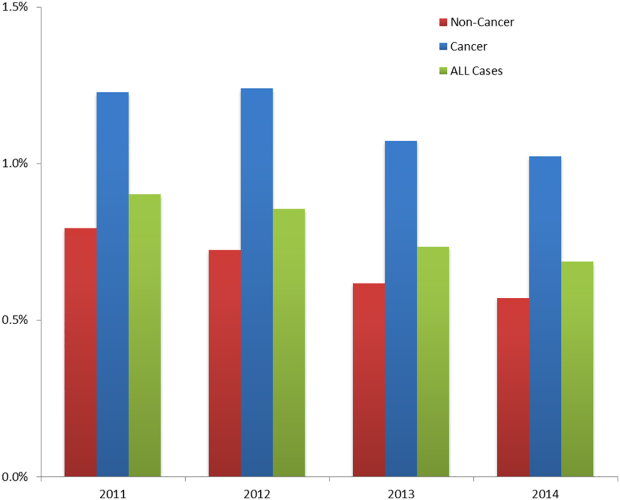
Surgical Versus Medical Patients
The impact of the VTE prevention initiative was only significant in surgical patients, for whom the risk of HA‐VTE fell by 28% (RR: 0.72, 95% CI: 0.63‐0.82) (Table 1). Medical patients experienced a nonsignificant 10% reduction in HA‐VTE (RR: 0.90, 95% CI: 0.73‐1.11). Medical patients had a significantly lower baseline incidence of HA‐VTE (0.57%) compared with surgical patients (1.14%; relative difference: 50%, P < 0.001). This finding persisted postimplementation, with a cumulative incidence in medical patients of 0.51% versus 0.82% in surgical patients (relative difference: 31%, P < 0.001).
DISCUSSION
Our initiative, comprised of a collaborative infrastructure, a proven quality‐improvement framework, and a bundle of interventions, was associated with a 24% reduction in the risk of HA‐VTE across our 5 academic medical centers. This represents avoidance of significant clinical morbidity (an estimated 81 PEs and 89 DVTs per year) and significant cost. Assuming costs of $9250 per DVT and $13,050 per PE,[17] the estimated short‐term cost savings are almost $1.9 million per year (minus expenditures on VTEP). Further savings might be expected over a longer time horizon because of the avoidance of recurrent VTE, post‐thrombotic syndrome, and the costs and complications of long‐term anticoagulation.
We believe the highly variable degree of improvement seen across our 5 sites was due to the relatively mature VTEP efforts at the onset of this collaborative improvement effort at UCSD and UCSF. As we noted earlier, the interventional bundle and methods were derived from earlier work at UCSD that had already demonstrated published marked improvement in prophylaxis and a 40% decrease in HA‐VTE.[14] The narrow range of low HA‐VTE rates in 2014 (the mature intervention time period) suggests there may be some HA‐VTE rate beyond which further prevention efforts are less productive.
Our study has several limitations. As a longitudinal collaborative improvement effort introducing a bundle of interventions, we cannot ascribe improved outcomes to individual components in the bundle; for example, we did not record the number of measure‐vention calls or resulting prophylaxis changes. We also did not measure adverse events due to VTEP, believing benefits to be greater than risks, but some adverse events likely did occur and attenuated benefits and cost savings. Although we had rigorous measures to assess the prevalence of appropriate prophylaxis, we failed to capture the baseline rate of VTEP, which means we cannot show that improved HA‐VTE rates corresponded to improvements in VTEP rates. The bundle of interventions was not implemented uniformly. Some metrics, like adherence to mechanical prophylaxis, were monitored in a decentralized fashion, without collation or collective analysis.
Were improved VTE rates due to decreases in HA‐VTE detection? We could not detect postdischarge HA‐VTE that presented to other hospitals, but we have no reason to think the proportion of missed HA‐VTE changed over the study. We discouraged the practice of routinely extending duplex ultrasound testing below the knee, and also discouraged surveillance of asymptomatic patients with Doppler ultrasound. This raises the question of ascertainment bias. Did we have fewer HA‐VTE in 2014 because our interventions worked, or did we reduce how aggressively we looked for HA‐VTE? Higher frequencies of ultrasound testing are correlated with higher rates of DVT because of surveillance bias.[18] Although some reduction in DVT was due to changes in ultrasound practices, several factors suggest the majority of improvement resulted from our interventions. First, only 1 of our 5 sites (UCD) routinely extended ultrasound testing below the knee in the baseline period. Second, we distinguished distal DVT from proximal/unspecified DVT, and the rates of both showed significant improvement. Screening asymptomatic patients with ultrasounds for DVT was limited to a few services in special circumstances (for example, the trauma service at UCSD screened patients at highest risk who could not be prophylaxed with anticoagulation). We did not have the capability to formally track which patients were being diagnosed with screening exams versus for symptoms, but screen‐detected patients were a small minority. We did not successfully dissuade these few services from stopping this approach, but we did head off some services that were considering this strategy, and think it likely that at best, we kept screening from spreading. Third, PE was reduced by over 20%, in addition to reductions in DVT, even though several of our sites acquired computed tomography scanners more sensitive for small thrombi/emncidental PE. Finally, the aggressiveness of ultrasound testing often goes up with aggressive prevention efforts, which would have led to surveillance bias with increasedrather than decreasedrates of HA‐VTE.
Our study has a number of strengths. Our effort encompassed a large and inclusive adult inpatient population over a long period of observation, with a relatively large reduction in HA‐VTE. These reductions occurred even though the proportion of patients with cancer (our most powerful predictor of VTE risk) was 34.8% in 2014 versus 33.3% in 2011. Our metrics captured patients readmitted to the hospital within 30 days of a prior VTE‐free admission as well as patients suffering VTE during the hospital stay, with the limitation that we captured only patients readmitted back to our own institutions. Our metrics for VTEP scrutinized prophylaxis rates at different points during hospitalizations, and risk‐appropriate prophylaxis was assessed, in contrast to some common regulatory measures that monitor only whether any prophylaxis is in place on the first day of admission or transfer.[11]
Our study should be instructive in terms of focusing improvement efforts. The rate of HA‐VTE was much higher in cancer and surgical patients than in medical patients, and we only achieved a nonsignificant 10% reduction in risk among medical patients (RR: 0.90, 95% CI: 0.73‐1.11). This is consistent with literature demonstrating a more limited benefit of prophylaxis in medical inpatients.[19] Although we continue to recommend prophylaxis in high‐risk medical inpatients, efforts targeting cancer and surgical populations are likely to yield greater results.
Our collaborative used methods that are portable, sustainable, and provide an excellent platform for spread of improvement across a system. The portability of these strategies is underlined by the variable baseline performance and the different stages of electronic health record development at our unique sites. Toolkits that describe the interventions (such as order sets, educational tools, measures, measure‐vention) are freely available, and reflect established guidelines.[1] Our collaborative model is consistent with successful models published in the literature.[1, 14, 20] In these models, clinical experts distill the evidence down into key best practices, and design processes that need to occur with the lowest barriers to use. Metrics, expert advice, and toolkits are assembled centrally, while each hospital identifies local barriers to implementation, educates and engages staff, executes implementation, and continually evaluates performance, modifying interventions accordingly. Embedding clinical decision and risk‐assessment into VTE prevention modules within commonly used order sets and documentation tools helps to hard‐wire the interventions, tightly linking risk assessment to appropriate prophylaxis options. The approach to standardization allows for flexibility for special populations and special needs of unique patients, while minimizing needless variation based on the ordering providers. Program management tools and regular webinars keeps sites on track, coordinate interventions, sustain enthusiasm, and provide a venue for sharing tools and lessons learned. Multiple active interventions are utilized rather than relying on passive educational techniques or order sets alone. Active surveillance (i.e., measure‐vention) deserves special attention. Measure‐vention has demonstrated utility in inpatient glycemic control and a variety of hospital‐associated infections in addition to VTE prevention, and some systems now uses measure‐ventionists as the lynchpin for a whole host of successful improvement programs.[12, 14, 21, 22] We believe high‐quality metrics, standardized protocol‐driven order sets, and measure‐vention are the crucial elements for success.
CONCLUSIONS
Hospital systems can reduce HA‐VTE by implementing a bundle of active interventions including standardized VTEP orders with embedded risk assessment and measure‐vention. Good measurement of HA‐VTE, appropriate VTEP that exceeds minimum regulatory standards, and a robust collaborative infrastructure inform and accelerate improvement. Surgical and cancer populations are at higher risk for HA‐VTE and should be a prime focus of improvement efforts.
Disclosures
Ian H Jenkins: nothing to report. Alpesh N. Amin: nothing to report. Nasim Afsarmanesh: nothing to report. Dr. Auerbach receives honorarium as Editor‐in‐Chief of the Journal of Hospital Medicine. Dr. Khanna has licensed technology to the hospital‐based electronic messaging vendor Voalte and will benefit financially from its dissemination. This does not impact this work. Dr. Maynard acts as a consultant on an expert panel overseeing a multinational trial of extended VTE prophylaxis in high‐risk medical patients (Medically Ill Patient Assessment of Rivaroxaban Versus Placebo in Reducing Post‐Discharge Venous Thrombo‐Embolism Risk), a study funded by Johnson & Johnson. Dr. White has acted as a consultant for Janssen, Boehringer‐Ingleheim, Diiachi‐Sankyo, and Bristol Meyer Squibb, and provides expert testimony for various malpractice defense lawyers for VTE, and has a grant with the Gordon and Betty Moore Foundation regarding VTE prevention.
Venous thromboembolism (VTE), comprised of pulmonary embolism (PE) and deep vein thrombosis (DVT), impacts hundreds of thousands of Americans annually.[1] The complications of VTE can be severe, including the post‐thrombotic syndrome, pulmonary hypertension, and complications of anticoagulation. VTE is often a complication of hospitalization, and PE is a common preventable cause of hospital mortality.[2, 3] Pharmacologic VTE prophylaxis (VTEP) in at‐risk patients is effective and endorsed by prominent guidelines.[4, 5, 6] However, VTEP is underutilized, with only 30% to 50% of eligible patients receiving the right drug, dose, and duration.[7, 8]
Public reporting and reimbursement policies reflect the magnitude of VTE as a public health concern. The Centers for Medicare and Medicaid Services (CMS) withholds incremental payment for VTE complications.[9] The rate of hospital‐associated VTE (HA‐VTE) is used by benchmarking organizations as a quality indicator.[10, 11]
The University of California (UC) has 5 major academic medical centers, located in Irvine (UCI), Los Angeles (UCLA), Sacramento (UC Davis [UCD]), San Diego (UCSD), and San Francisco (UCSF). In both 2010 and 2011, almost 700 UC patients suffered from HA‐VTE annually. Barriers to optimal VTEP included the absence of standardized VTE risk assessment, lack of consensus on appropriate VTEP options for various inpatient populations, and a lack of collaborative infrastructure. Other barriers included poor adherence to mechanical prophylaxis and suboptimal measurement of prophylaxis and HA‐VTE outcomes.
In late 2011, leaders from the 5 medical centers, supported by an internal competitive grant from the UC Office of the President and the Center for Health Quality and Innovation, formed a collaborative to address barriers, optimize VTEP in inpatients, and reduce HA‐VTE across the system. Prior efforts at UCSD illustrated single‐center improvement, with an increase in adequate VTEP from 50% to over 95%, and a nearly 40% reduction in the incidence of HA‐VTE.[12] We set out to scale this success across all 5 sites as a coordinated collaborative.
METHODS
This was a prospective, unblinded, open‐intervention study with historical controls that assessed prespecified outcomes before, during, and after institution of multiple VTEP strategies in 5 independent, but cooperating, academic hospitals. All adult medical and surgical inpatients were included; psychiatric, obstetricsgynecology, rehabilitation, observation status, and pediatric populations were excluded. The study period was July 1, 2012 through June 30, 2015. Calendar year (CY) 2011 was the baseline year for comparison; interventions were initiated in CY 2012 to CY 2014, and CY 2014 was considered the mature postintervention period.
Hospital Collaboration
Multiprofessional teams[1] were formed at each site. Monthly webinars, regular e‐mail, minutes, and a project management plan with task lists were utilized for coordinated collaboration. Software (Dropbox) was used for sharing tools, educational materials, and measurement techniques. REDCap (Research Electronic Data Capture) was used for secure data collection and analysis of outcomes.[13] Prior experience at UCSD and the Society of Hospital Medicine informed measurement and intervention bundle strategies.[1, 12, 14] Surveys of baseline VTE prevention protocols, measures, and order sets were performed at each site. Measures were standardized, whereas the intervention bundle was tailored for use at each medical center. Institutional review board approval with a waiver for individualized informed consent was obtained.
Interventions
All sites were tasked with implementing a defined bundle of mutually reinforcing interventions that constituted a comprehensive VTE prevention program. These protocols, order sets, educational programs, and interventions were not designed or implemented in an identical fashion at each hospital, but common principles were utilized.
VTE Prevention Protocol
This protocol incorporated (1) standardized VTE risk assessment, and (2) links to a menu of appropriate prophylaxis options for each level of risk that included guidance for management of patients with contraindications to pharmacologic prophylaxis. We used simple risk‐assessment models that grouped patients into 3 levels of risk (the 3‐bucket model) rather than more complicated point‐based systems. The 3‐bucket model was designed to offer detailed guidance and avoid over‐prophylaxis. Protocol, measurement, and order set tools were modified for special populations, such as orthopedic and neurosurgery populations. Operational definitions for bleeding risk, DVT risk, and exceptions to the protocol were explicit, which allowed for classification of adequate versus inadequate prophylaxis. High‐risk patients required combination prophylaxis, moderate risk anticoagulant prophylaxis, and low risk patients no prophylaxis beyond ambulation protocols (in the absence of contraindications). Acceptable contraindications to pharmacologic prophylaxis included an international normalized ratio >1.8, platelet count <50,000, active hemorrhage within the last 3 days, known bleeding disorders, hypertensive urgencies/emergencies, comfort careonly status, and leeway times around surgery or other events (24 hours for most surgeries, 48 hours for transplant surgery or major trauma, up to a week after central nervous system surgery). Impaired mobility was considered present unless the patient could ambulate independently more than once a day. More details regarding 3‐bucket risk models and explicit criteria can be reviewed in a recent Agency for Healthcare Quality and Research (AHRQ) publication.[1] The protocol was embedded into clinical decision‐support as required elements of admission, transfer, and postoperative order sets.
Educational Programs
Nurse and physician education programs were developed that stressed the importance of VTE prevention and adherence to thromboprophylaxis, including mechanical prophylaxis. The VTEP protocol was socialized in medical staff and nursing meetings. The educational programs recommended imaging only the proximal veins in patients with symptoms of leg DVT, and avoiding screening ultrasounds in asymptomatic patients. Physicians were coached on how to use the VTEP order sets. Content for educational programs was discussed and often shared among sites, but educational programs were tailored locally to fit perceived needs and available resources.
Measure‐vention
An active surveillance and feedback program called measure‐vention was developed to provide ongoing feedback to care providers regarding the appropriate use of VTEP over the duration of hospitalization. Key features of measure‐vention were regular measurement of adherence/lapses in VTEP delivery, coupled with concurrent intervention to correct any lapses, with a nurse/pharmacist calling the primary team if VTEP was suboptimal.[1, 12] Measure‐vention was utilized to monitor both appropriateness of orders and adherence with ordered prophylaxis, and was used to correct overprophylaxis as well as underprophylaxis. For example, our protocol specified that moderate VTE risk patients with a captured contraindication to anticoagulant should be on mechanical prophylaxis. An intervention would take place if mechanical prophylaxis was not ordered, or if it was ordered but not documented as being in place. Measure‐vention examples and further description are available in AHRQ publications.[1]
Outcomes
Thromboprophylaxis Rates
We planned to perform structured chart review on at least 30 noncritical care and 15 critical care adult inpatients per month at each site. Adult inpatients with a length of stay >48 hours, stratified by critical care versus noncritical care status, were assigned a numeric value by a random number generator. Patients were selected in order of random number assignment for chart review until the desired number of audits was completed. Development of the audit tools, as well as availability of personnel, led to delays in assessing prophylaxis rates by these standards until late 2012 to early 2013 at each site. A few sites had brief lapses in data collection during personnel changes. VTE risk, bleeding risk, prophylaxis ordered at the time of the audit, and adequacy of VTEP defined by a common standard were all assessed and recorded in the REDCap data repository. VTEP was considered adequate if combined pharmacologic and mechanical prophylaxis was present in the highest‐risk patients or anticoagulant prophylaxis was present in moderate patients. Prophylaxis was considered adequate for all low‐risk patients. Patients at risk for VTE with contraindications to anticoagulants were considered to be on adequate prophylaxis if they received mechanical prophylaxis or had documented contraindications to mechanical prophylaxis. The proper administration of ordered prophylaxis was scrutinized locally and targeted by education and other interventions at each site, but these data were not collated and analyzed centrally.
Identification of HA‐VTE
HA‐VTE rates were determined by administrative coding data, using International Classification of Diseases, Ninth Revision, Clinical Modification (ICD‐9‐CM) codes in a manner similar to AHRQ Patient Safety Indicator 12 identification of postoperative VTE cases.[10] Data were submitted by each hospital, then collated and analyzed using data from Vizient (formerly the University HealthSystem Consortium). The incidence of VTE was determined using specific ICD‐9‐CM hospital discharge codes: for PE: 415.11, 415.13, 415.19, 673.24; and for DVT: proximal DVT: 451.11, 451.19, 451.81, 453.41; distal DVT: 453.42; and other DVT: 453.40, 453.8. These codes have high positive predictive value for acute VTE.[15, 16] Mean age, average length of stay (ALOS), and admission severity of illness (SOI) scores were also captured from Vizient and summarized for the inpatient cohort each year.
All VTE cases were coupled with present on admission (POA) indicators. HA‐VTE cases included patients who were readmitted to the same hospital within 30 days for a new event (POA = Y, but readmitted), as well as patients who developed PE or DVT during their hospitalization (POA = N or U). Only patients hospitalized for 3 or more days were analyzed for inpatient development of VTE, as diagnosis of VTE in the first 2 days was deemed either likely present on admission or not preventable using VTEP started within 24 hours of admission. VTE outcomes were assigned in a hierarchical fashion: if both PE and DVT were present, the case was classified as PE. Distal DVT was distinguished from proximal DVT whenever possible. Cases were stratified based on whether the patient had undergone a major operation (surgery patients) or not (medical patients). This stratification was based on the Medicare Severity Diagnosis‐Related Group (MS‐DRG) coded in patient records. The DRG type for each MS‐DRG was based on the 2015 CMS‐MS‐DRG codes for major operations,[9] except that all trauma cases were considered surgical, and cases with vena cava filter placement and no other surgical procedure were considered medical. Cancer cases were identified using ICD‐9‐CM codes 140.00‐209.99 and 210.00‐239.99.
Review of HA‐VTE
Periodic review of selected HA‐VTE cases identified by administrative coding data was recommended as a best practice, potentially adding insight to contributing factors to HA‐VTE, included lapses in prophylaxis and suboptimal mobilization. The accuracy of diagnostic coding, and assessment of how HA‐VTE cases were identified (symptoms vs screening ultrasounds) could also be assessed. Examples of audit tools were shared. Every site reviewed some HA‐VTE cases, but the extent and duration of case review was left to the discretion of each site.
Statistical Analysis
Relative risk (RR) calculations with 95% confidence intervals (CI) were used to compare the proportions of patients with PE, DVT alone, and total HA‐VTE in 2014 versus 2011. The absolute risk reduction was multiplied by the population at risk in CY 2014 to arrive at estimates of cases of VTE averted in 2014 compared to 2011.
RESULTS
Robust sampling (421 to 728 patients at each site) revealed attainment of high rates of adequate VTE prophylaxis (82% to 96% at all sites, collectively 89%) by early 2014. Common measures for adequate VTEP were not finalized and collected by all sites until early 2013, so we did not capture baseline VTEP rates, and could not compare baseline to mature prophylaxis rates. Reliable administration of mechanical and anticoagulant prophylaxis was monitored and targeted by each institution, albeit not in an identical fashion at each site. Adherence to mechanical prophylaxis was reported as improved at the sites, but these data were not collated and analyzed centrally.
Population Demographics and Severity of Illness
There were 73,941 to 79,565 discharges that met the criteria (adult medicalsurgical inpatient with >2 day length of stay each year. Mean age and ALOS were unchanged or had no change of clinical significance. For example, in 2011 versus 2014, mean age was 55.7 versus 56.4 years, and ALOS was identical in both time periods at 7.4 days. Admission SOI scores also remained fairly static from 2011 to 2014 (2.27, 2.31, 2.32, 2.26, respectively), and the admission SOI was not statistically different in 2011 versus 2014 (estimated difference of 2 means 0.01, 95% CI: 0.00‐0.02).
Hospital‐Associated VTE
There were 2431 HA‐VTE events observed in 306,906 adult inpatients across CY 2011 to 2014 (Table 1). The baseline incidence of HA‐VTE was 0.90% (667 events in 73,941 hospitalizations in 2011). The incidence of HA‐VTE in the postintervention period was 0.69% (546 HA‐VTE events in 79,565 hospitalizations in 2014, P < 0.001), an overall reduction of 24%. The absolute risk for PE decreased from 0.49% to 0.39% (RR: 0.79, 95% CI: 0.68‐0.92), a reduction of 21%, and the absolute risk of leg DVT fell from 0.41% to 0.30% (RR: 0.73, 95% CI: 0.61‐0.86), a reduction of 27%. Both proximal and distal DVT were reduced significantly. Proximal DVT was much more commonly diagnosed than distal DVT. Proximal DVT incidence decreased from 0.32% to 0.25% (RR: 0.77, 95% CI: 0.64‐0.93), whereas distal DVT incidence decreased from 0.09% to 0.05% (RR: 0.58, 95% CI: 0.39‐0.86). The lower overall VTE rate in the postimplementation period compared with the baseline period corresponds to an estimated 170 fewer cases of VTE per year (89 DVT, 81 PE).
2011 (Baseline), No./% | 2012, No./% | 2013, No./% | 2014 (Mature), No./% | 2014 Versus 2011 Relative Risk (95% CI) | 2014 Versus 2011 Estimated Averted Events (95% CI) | |
---|---|---|---|---|---|---|
| ||||||
Total discharges (medical and surgical) | 73,941 | 76,100 | 77,300 | 79,565 | ||
Total PE + leg DVT | 667/0.90% | 650/0.85% | 568/0.73% | 546/0.69% | 0.761 (0.680‐0.852) | 170 (103‐247) |
Total PE | 363/0.49% | 359/0.47% | 340/0.44% | 309/0.39% | 0.791 (0.680‐0.920) | 81 (32‐135) |
Total leg DVT | 304/0.41% | 291/0.38% | 228/0.29% | 237/0.3% | 0.725 (0.612‐0.858) | 89(40‐135) |
Medical discharges | 31,219 | 32,597 | 33,805 | 34,875 | ||
Total PE + leg DVT | 178/0.57% | 168/0.52% | 164/0.49% | 179/0.51% | 0.900 (0.732‐1.1071) | |
PE | 110/0.35% | 94/0.29% | 106/0.31% | 104/0.30% | 0.846 (0.648‐1.106) | |
Leg DVT | 68/0.22% | 74/0.23% | 58/0.17% | 75/0.22% | 0.987 (0.711‐1.371) | |
Surgical discharges | 42,722 | 43,503 | 43,495 | 44,690 | ||
Total PE + leg DVT | 489/1.14% | 482/1.11% | 404/0.93% | 367/0.82% | 0.718 (0.627‐0.821) | |
PE | 253/0.59% | 265/0.61% | 234/0.54% | 205/0.46% | 0.775 (0.645‐0.931) | |
Leg DVT | 236/0.55% | 217/0.50% | 180/0.41% | 162/0.36% | 0.656 (0.538‐0.801) |
The baseline rate of HA‐VTE and degree of improvement varied between institutions (Figure 1). UCI and UCD began the study with significantly higher VTE rates, and enjoyed the largest improvements. UCLA's VTE rate decreased to a lesser extent, whereas UCSD and UCSF rates remained relatively flat or were marginally higher. In contrast to the highly variable 2011 baseline rate of HA‐VTE (0.60%1.36%), all 5 sites had HA‐VTE rates within a very narrow range (0.65%0.73%) at maturity in 2014.
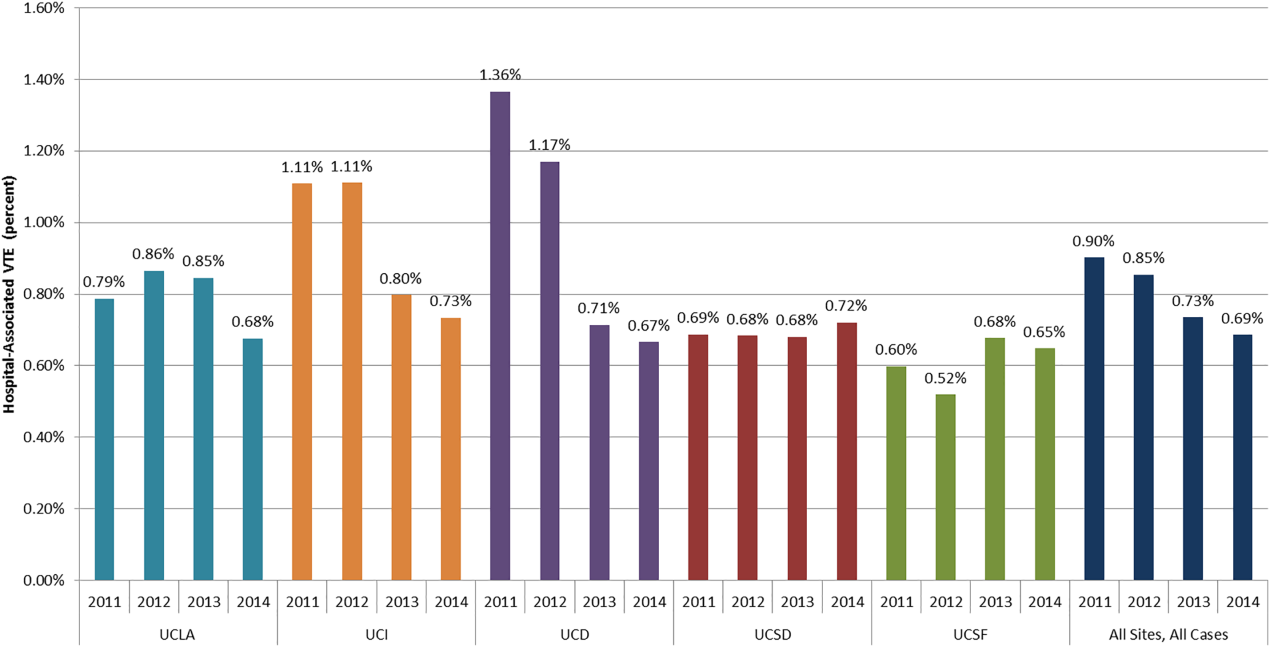
Cancer Versus Noncancer Patients
The incidence of HA‐VTE was higher in cancer patients than in noncancer patients. In 2011, 227 of 18,487 (1.23%) cancer patients developed VTE, versus 440 of 55,454 (0.79%) noncancer patients (Figure 2). After implementation of the VTE prevention initiative, the incidence of VTE in cancer patients fell by 0.21% (210 events in 20,544 patients in 2014, 1.02%), and the incidence of VTE in noncancer patients fell by 0.22% (336 events in 59,021 patients, 0.57%). The relative risk of HA‐VTE after the VTE interventions was reduced by 17% (RR: 0.83, 95% CI: 0.69‐1.00) in cancer patients and 28% (RR: 0.72, 95% CI: 0.62‐0.83) in noncancer patients.
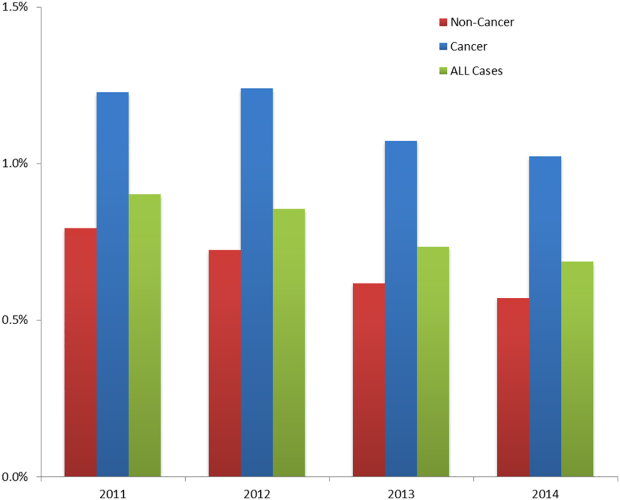
Surgical Versus Medical Patients
The impact of the VTE prevention initiative was only significant in surgical patients, for whom the risk of HA‐VTE fell by 28% (RR: 0.72, 95% CI: 0.63‐0.82) (Table 1). Medical patients experienced a nonsignificant 10% reduction in HA‐VTE (RR: 0.90, 95% CI: 0.73‐1.11). Medical patients had a significantly lower baseline incidence of HA‐VTE (0.57%) compared with surgical patients (1.14%; relative difference: 50%, P < 0.001). This finding persisted postimplementation, with a cumulative incidence in medical patients of 0.51% versus 0.82% in surgical patients (relative difference: 31%, P < 0.001).
DISCUSSION
Our initiative, comprised of a collaborative infrastructure, a proven quality‐improvement framework, and a bundle of interventions, was associated with a 24% reduction in the risk of HA‐VTE across our 5 academic medical centers. This represents avoidance of significant clinical morbidity (an estimated 81 PEs and 89 DVTs per year) and significant cost. Assuming costs of $9250 per DVT and $13,050 per PE,[17] the estimated short‐term cost savings are almost $1.9 million per year (minus expenditures on VTEP). Further savings might be expected over a longer time horizon because of the avoidance of recurrent VTE, post‐thrombotic syndrome, and the costs and complications of long‐term anticoagulation.
We believe the highly variable degree of improvement seen across our 5 sites was due to the relatively mature VTEP efforts at the onset of this collaborative improvement effort at UCSD and UCSF. As we noted earlier, the interventional bundle and methods were derived from earlier work at UCSD that had already demonstrated published marked improvement in prophylaxis and a 40% decrease in HA‐VTE.[14] The narrow range of low HA‐VTE rates in 2014 (the mature intervention time period) suggests there may be some HA‐VTE rate beyond which further prevention efforts are less productive.
Our study has several limitations. As a longitudinal collaborative improvement effort introducing a bundle of interventions, we cannot ascribe improved outcomes to individual components in the bundle; for example, we did not record the number of measure‐vention calls or resulting prophylaxis changes. We also did not measure adverse events due to VTEP, believing benefits to be greater than risks, but some adverse events likely did occur and attenuated benefits and cost savings. Although we had rigorous measures to assess the prevalence of appropriate prophylaxis, we failed to capture the baseline rate of VTEP, which means we cannot show that improved HA‐VTE rates corresponded to improvements in VTEP rates. The bundle of interventions was not implemented uniformly. Some metrics, like adherence to mechanical prophylaxis, were monitored in a decentralized fashion, without collation or collective analysis.
Were improved VTE rates due to decreases in HA‐VTE detection? We could not detect postdischarge HA‐VTE that presented to other hospitals, but we have no reason to think the proportion of missed HA‐VTE changed over the study. We discouraged the practice of routinely extending duplex ultrasound testing below the knee, and also discouraged surveillance of asymptomatic patients with Doppler ultrasound. This raises the question of ascertainment bias. Did we have fewer HA‐VTE in 2014 because our interventions worked, or did we reduce how aggressively we looked for HA‐VTE? Higher frequencies of ultrasound testing are correlated with higher rates of DVT because of surveillance bias.[18] Although some reduction in DVT was due to changes in ultrasound practices, several factors suggest the majority of improvement resulted from our interventions. First, only 1 of our 5 sites (UCD) routinely extended ultrasound testing below the knee in the baseline period. Second, we distinguished distal DVT from proximal/unspecified DVT, and the rates of both showed significant improvement. Screening asymptomatic patients with ultrasounds for DVT was limited to a few services in special circumstances (for example, the trauma service at UCSD screened patients at highest risk who could not be prophylaxed with anticoagulation). We did not have the capability to formally track which patients were being diagnosed with screening exams versus for symptoms, but screen‐detected patients were a small minority. We did not successfully dissuade these few services from stopping this approach, but we did head off some services that were considering this strategy, and think it likely that at best, we kept screening from spreading. Third, PE was reduced by over 20%, in addition to reductions in DVT, even though several of our sites acquired computed tomography scanners more sensitive for small thrombi/emncidental PE. Finally, the aggressiveness of ultrasound testing often goes up with aggressive prevention efforts, which would have led to surveillance bias with increasedrather than decreasedrates of HA‐VTE.
Our study has a number of strengths. Our effort encompassed a large and inclusive adult inpatient population over a long period of observation, with a relatively large reduction in HA‐VTE. These reductions occurred even though the proportion of patients with cancer (our most powerful predictor of VTE risk) was 34.8% in 2014 versus 33.3% in 2011. Our metrics captured patients readmitted to the hospital within 30 days of a prior VTE‐free admission as well as patients suffering VTE during the hospital stay, with the limitation that we captured only patients readmitted back to our own institutions. Our metrics for VTEP scrutinized prophylaxis rates at different points during hospitalizations, and risk‐appropriate prophylaxis was assessed, in contrast to some common regulatory measures that monitor only whether any prophylaxis is in place on the first day of admission or transfer.[11]
Our study should be instructive in terms of focusing improvement efforts. The rate of HA‐VTE was much higher in cancer and surgical patients than in medical patients, and we only achieved a nonsignificant 10% reduction in risk among medical patients (RR: 0.90, 95% CI: 0.73‐1.11). This is consistent with literature demonstrating a more limited benefit of prophylaxis in medical inpatients.[19] Although we continue to recommend prophylaxis in high‐risk medical inpatients, efforts targeting cancer and surgical populations are likely to yield greater results.
Our collaborative used methods that are portable, sustainable, and provide an excellent platform for spread of improvement across a system. The portability of these strategies is underlined by the variable baseline performance and the different stages of electronic health record development at our unique sites. Toolkits that describe the interventions (such as order sets, educational tools, measures, measure‐vention) are freely available, and reflect established guidelines.[1] Our collaborative model is consistent with successful models published in the literature.[1, 14, 20] In these models, clinical experts distill the evidence down into key best practices, and design processes that need to occur with the lowest barriers to use. Metrics, expert advice, and toolkits are assembled centrally, while each hospital identifies local barriers to implementation, educates and engages staff, executes implementation, and continually evaluates performance, modifying interventions accordingly. Embedding clinical decision and risk‐assessment into VTE prevention modules within commonly used order sets and documentation tools helps to hard‐wire the interventions, tightly linking risk assessment to appropriate prophylaxis options. The approach to standardization allows for flexibility for special populations and special needs of unique patients, while minimizing needless variation based on the ordering providers. Program management tools and regular webinars keeps sites on track, coordinate interventions, sustain enthusiasm, and provide a venue for sharing tools and lessons learned. Multiple active interventions are utilized rather than relying on passive educational techniques or order sets alone. Active surveillance (i.e., measure‐vention) deserves special attention. Measure‐vention has demonstrated utility in inpatient glycemic control and a variety of hospital‐associated infections in addition to VTE prevention, and some systems now uses measure‐ventionists as the lynchpin for a whole host of successful improvement programs.[12, 14, 21, 22] We believe high‐quality metrics, standardized protocol‐driven order sets, and measure‐vention are the crucial elements for success.
CONCLUSIONS
Hospital systems can reduce HA‐VTE by implementing a bundle of active interventions including standardized VTEP orders with embedded risk assessment and measure‐vention. Good measurement of HA‐VTE, appropriate VTEP that exceeds minimum regulatory standards, and a robust collaborative infrastructure inform and accelerate improvement. Surgical and cancer populations are at higher risk for HA‐VTE and should be a prime focus of improvement efforts.
Disclosures
Ian H Jenkins: nothing to report. Alpesh N. Amin: nothing to report. Nasim Afsarmanesh: nothing to report. Dr. Auerbach receives honorarium as Editor‐in‐Chief of the Journal of Hospital Medicine. Dr. Khanna has licensed technology to the hospital‐based electronic messaging vendor Voalte and will benefit financially from its dissemination. This does not impact this work. Dr. Maynard acts as a consultant on an expert panel overseeing a multinational trial of extended VTE prophylaxis in high‐risk medical patients (Medically Ill Patient Assessment of Rivaroxaban Versus Placebo in Reducing Post‐Discharge Venous Thrombo‐Embolism Risk), a study funded by Johnson & Johnson. Dr. White has acted as a consultant for Janssen, Boehringer‐Ingleheim, Diiachi‐Sankyo, and Bristol Meyer Squibb, and provides expert testimony for various malpractice defense lawyers for VTE, and has a grant with the Gordon and Betty Moore Foundation regarding VTE prevention.
- Preventing Hospital‐Associated Venous Thromboembolism: A Guide for Effective Quality Improvement. 2nd ed. Rockville, MD: Agency for Healthcare Research and Quality; October 2015. AHRQ Publication No. 16–001‐EF. Available at: http://www.ahrq.gov/professionals/quality‐patient‐safety/patient‐safety‐resources/resources/vtguide/index.html. Accessed June 1, 2016. .
- Relative impact of risk factors for deep vein thrombosis and pulmonary embolism. Arch Intern Med. 2002;162:1245–1248. , , , et al.
- Antithrombotic therapy practices in US hospitals in an era of practice guidelines. Arch Intern Med. 2005;165:1458–1464. , , , et al.
- Prevention of VTE in nonsurgical patients. Chest. 2012;141(2 suppl):e195S–e226S. , , , et al.
- Prevention of VTE in nonorthopedic surgical patients. Chest. 2012;141(2 suppl):e227S–e277S. , , , et al.
- Prevention of VTE in orthopedic surgery patients. Chest. 2012;141(2 suppl):e278S–e325S. , , , et al.
- The outcome after treatment of venous thromboembolism is different in surgical and acutely ill medical patients. Findings from the RIETE registry. J Thromb Haemost. 2004;2:1892–1898. , , , et al.
- Inpatient thromboprophylaxis use in U.S. hospitals: adherence to the Seventh American College of Chest Physician's recommendations for at‐risk medical and surgical patients. J Hosp Med. 2009;4:E15–E21. , , , .
- Centers for Medicare 5(1):10–18.
- Research electronic data capture (REDCap)—a metadata‐driven methodology and workflow process for providing translational research informatics support. J Biomed Inform. 2009;42:377–381. , , , et al.
- Mentored implementation: building leaders and achieving results through a collaborative improvement model. 2011 John M. Eisenberg Patient Safety and Quality Award, National Level. Jt Comm J Qual Patient Saf. 2012;38(7):301–310. , , , et al.
- Predictive value of the POA indicator for hospital‐acquired venous thromboembolism. Med Care. 2013:53(4):e31–e36. , , , et al.
- Improved coding of postoperative deep vein thrombosis and pulmonary embolism in administrative data (AHRQ patient safety indicator 12) after introduction of new ICD‐9‐CM diagnosis codes. Med Care. 2015:53(5):e37–e40. , , , et al.
- Economic burden of venous thromboembolism in hospitalized patients. Pharmacotherapy. 2009;29(8):943–953. .
- Evaluation of surveillance bias and the validity of the venous thromboembolism quality measure. JAMA. 2013;310(14):1482–1489. , , , et al.
- Venous thromboembolism prophylaxis in hospitalized medical patients and those with stroke: a background review for an American College of Physicians clinical practice guideline. Ann Intern Med. 2011;155(9):602–615. , , , et al.
- Translating evidence into practice: a model for large scale knowledge translation. BMJ. 2008; 337:a1714. , , .
- Impact of a hypoglycemia reduction bundle and a systems approach to inpatient glycemic management. Endocr Pract. 2015;21(4):355–367. , , , et al.
- Zero adverse events: how Dignity Health achieved a new standard. Becker's Hospital Review: Infection Control and Clinical Quality website. Available at: http://www.beckershospitalreview.com/quality/zero‐adverse‐events‐how‐dignity‐health‐achieved‐a‐new‐standard.html. Accessed April 19, 2016. .
- Preventing Hospital‐Associated Venous Thromboembolism: A Guide for Effective Quality Improvement. 2nd ed. Rockville, MD: Agency for Healthcare Research and Quality; October 2015. AHRQ Publication No. 16–001‐EF. Available at: http://www.ahrq.gov/professionals/quality‐patient‐safety/patient‐safety‐resources/resources/vtguide/index.html. Accessed June 1, 2016. .
- Relative impact of risk factors for deep vein thrombosis and pulmonary embolism. Arch Intern Med. 2002;162:1245–1248. , , , et al.
- Antithrombotic therapy practices in US hospitals in an era of practice guidelines. Arch Intern Med. 2005;165:1458–1464. , , , et al.
- Prevention of VTE in nonsurgical patients. Chest. 2012;141(2 suppl):e195S–e226S. , , , et al.
- Prevention of VTE in nonorthopedic surgical patients. Chest. 2012;141(2 suppl):e227S–e277S. , , , et al.
- Prevention of VTE in orthopedic surgery patients. Chest. 2012;141(2 suppl):e278S–e325S. , , , et al.
- The outcome after treatment of venous thromboembolism is different in surgical and acutely ill medical patients. Findings from the RIETE registry. J Thromb Haemost. 2004;2:1892–1898. , , , et al.
- Inpatient thromboprophylaxis use in U.S. hospitals: adherence to the Seventh American College of Chest Physician's recommendations for at‐risk medical and surgical patients. J Hosp Med. 2009;4:E15–E21. , , , .
- Centers for Medicare 5(1):10–18.
- Research electronic data capture (REDCap)—a metadata‐driven methodology and workflow process for providing translational research informatics support. J Biomed Inform. 2009;42:377–381. , , , et al.
- Mentored implementation: building leaders and achieving results through a collaborative improvement model. 2011 John M. Eisenberg Patient Safety and Quality Award, National Level. Jt Comm J Qual Patient Saf. 2012;38(7):301–310. , , , et al.
- Predictive value of the POA indicator for hospital‐acquired venous thromboembolism. Med Care. 2013:53(4):e31–e36. , , , et al.
- Improved coding of postoperative deep vein thrombosis and pulmonary embolism in administrative data (AHRQ patient safety indicator 12) after introduction of new ICD‐9‐CM diagnosis codes. Med Care. 2015:53(5):e37–e40. , , , et al.
- Economic burden of venous thromboembolism in hospitalized patients. Pharmacotherapy. 2009;29(8):943–953. .
- Evaluation of surveillance bias and the validity of the venous thromboembolism quality measure. JAMA. 2013;310(14):1482–1489. , , , et al.
- Venous thromboembolism prophylaxis in hospitalized medical patients and those with stroke: a background review for an American College of Physicians clinical practice guideline. Ann Intern Med. 2011;155(9):602–615. , , , et al.
- Translating evidence into practice: a model for large scale knowledge translation. BMJ. 2008; 337:a1714. , , .
- Impact of a hypoglycemia reduction bundle and a systems approach to inpatient glycemic management. Endocr Pract. 2015;21(4):355–367. , , , et al.
- Zero adverse events: how Dignity Health achieved a new standard. Becker's Hospital Review: Infection Control and Clinical Quality website. Available at: http://www.beckershospitalreview.com/quality/zero‐adverse‐events‐how‐dignity‐health‐achieved‐a‐new‐standard.html. Accessed April 19, 2016. .
© 2016 Society of Hospital Medicine
VTE Codes in Academic Medical Centers
Pulmonary embolism (PE) and deep venous thrombosis (DVT), historically referred to together as venous thromboembolism (VTE), are common, treatable, sometimes fatal, and potentially preventable medical problems.[1] Such thromboses can both precipitate a hospitalization as well as complicate it (either during or soon after discharge). Preventing such thrombosis as a complication of medical care has become a national imperative. Landmark studies such as Prophylaxis in Medical Patients With Enoxaparin (MEDENOX)[2] and Prospective Evaluation of Dalteparin Efficacy for Prevention of VTE in Immobilized Patients Trial (PREVENT)[3] demonstrated both a high incidence of thrombosis in a hospitalized high‐risk medical population (15% and 5% in the 2 trials' placebo arms, respectively) as well as significant relative risk reduction through venous thromboembolism pharmacoprophylaxis (VTEP)63% and 45%, respectively. The Joint Commission,[4] the Society of Hospital Medicine,[5] and the American College of Chest Physicians[6, 7] have thus all strived to ensure the appropriate provision of VTEP in order to reduce the morbidity and mortality associated with thrombosis in hospitalized patients, including those on medical services.
Ideally, the global success of these efforts would be assessed by measuring the rate of hospital‐associated VTE (potentially including superficial venous thrombosis [SVT], which, like upper‐extremity deep venous thrombosis [UE‐DVT], is commonly a central venous catheter [CVC]‐associated, or peripherally inserted central catheter [PICC]‐associated, complication)thrombosis acquired and diagnosed during either the index hospitalization (hospital‐acquired, or HA‐VTE/SVT) or up to 30 days postdischarge. Unfortunately, postdischarge VTE/SVT is difficult to measure because patients developing it may not present to the original hospital, or at all (eg, if they do not seek care, are treated as outpatients, or, in the most extreme case, die at home). In this context, despite being far less comprehensive, HA‐VTE/SVT is a useful subset of hospital‐associated VTE/SVT, for several reasons. First, the Centers for Medicare & Medicaid Services (CMS) have mandated hospitals to qualify all medical diagnoses as present‐on‐admission (POA = Y) or not (POA = N) since 2008, such that all medical diagnoses coded POA = N can be considered hospital acquired.[8] Second, refinements made to the International Classification of Diseases, 9th Revision (ICD‐9) codes now allow differentiation of UE‐DVT and SVT from lower‐extremity (LE) DVT/PE, whereas the former were sometimes obscured by nonspecific coding.[9] Third, recent studies have shown that medical diagnoses administratively coded as HA‐VTE/SVT correlated well with HA‐VTE/SVT ascertained through chart review.[9, 10] Finally, previous work has estimated that approximately half of all hospital‐associated VTE are HA‐VTE and the other half are postdischarge VTE.[11] Thus, HA‐VTE, though comprising only approximately half of all hospital‐associated VTE, is often used as a surrogate for measuring the success of ongoing VTE prevention programs.[12]
Our study aimed to assess the incidence of HA‐VTE plus HA‐SVT in the era of mandatory POA coding and newer ICD‐9 codes for VTE.
METHODS
Setting and Cases
We conducted a retrospective analysis of discharges from the 83 academic medical centers belonging to the UHC (formerly, the University HealthSystem Consortium,
Patients in our analysis were age 18 years and discharged with a medical medical severity diagnostic‐related group (MS‐DRG) code, hospitalized for 48 hours, and did not have a surgical or obstetric MS‐DRG code (except when assigned a surgical MS‐DRG code solely due to insertion of an inferior vena cava filter, with no other major procedures performed). Cases excluded discharges with a principal diagnosis of acute VTE/SVT (defined here as including PE, LE‐DVT, UE‐DVT, SVT, chronic VTE, and thrombosis not otherwise specified), as coding guidelines prohibit assigning a HA‐VTE as the principal diagnosis for the index hospitalization.[14]
Hospital‐Acquired Venous Thromboembolism or Superficial Venous Thrombosis
Cases were classified as having a HA‐VTE/SVT if there was 1 VTE/SVT coded in a secondary diagnosis position (other diagnosis) with a corresponding POA indicator equal to either N (not POA) or U (documentation insufficient to clarify whether VTE was POA or not). This usage corresponds to CMS guidelines and reimbursement policies for hospital‐acquired conditions.[15] Among cases with 1 HA‐VTE (or SVT), we assigned 1 HA‐VTE diagnosis using a hierarchy based on the highest level of clinical importance: first, PE; then LE‐DVT; then UE‐DVT; then SVT; then chronic VTE; then, finally, unspecified VTE. We subsequently excluded cases with primarily chronic VTE from our analysis because these were likely miscodes (ie, it is unclear how a chronic VTE could not be POA) and there were only 30 such cases. Cases with HA‐PE or HA‐LE DVT were analyzed separately as an important subset of HA‐VTE (plus SVT), because HA‐PE/LE‐DVT is both life‐threatening and theoretically preventable with VTEP.
Severity of Illness and Other Measures of Comorbidity
For each case we used proprietary software (3M Health Information Systems, Murray UT) to classify severity of illness (SOI). The SOI scale, based on physiologic derangement and organ system loss of function,[16] has 4 levels: minor, major, severe, and extreme. Defined within specific disease groups (All Patient Refined DRGs), it is often compared across diseases as well.[17] We also assessed whether patients had a cancer diagnosis, spent time in the intensive care unit (ICU), and died in the hospital.
Central Venous Catheter Use in Patients With Upper‐Extremity Deep Venous Thrombosis or Superficial Venous Thrombosis
Because UE‐DVT and SVT are frequently associated with a CVC or PICC, we assessed central venous catheterization among patients with an UE‐DVT or SVT of the cephalic, basilic, or antecubital veins using diagnosis codes for complications related to dialysis devices, implants, and grafts.
Pharmacologic Thromboprophylaxis
Pharmacy records of the subset of HA‐VTE/SVT cases with PE or LE‐DVT were analyzed to determine if VTEP was administered on hospital day 1 or 2, as per Joint Commission performance requirements.[4] Medications that met criteria as VTEP included unfractionated heparin, 5000 IU, given 2 or 3 a day; enoxaparin, 40 mg, given daily; dalteparin, 2500 or 5000 IU, given daily; fondaparinux, 2.5 mg, given daily; and warfarin. We could not reliably determine if VTEP was used throughout the entire hospitalization, or whether mechanical prophylaxis was used at all.
Statistical Analysis
This was a descriptive analysis to determine the incidence of HA‐VTE/SVT and describe the demographic and clinical characteristics of this population. We calculated means and standard deviations (SD) for continuous variables and proportions for binary variables (including HA‐VTE/SVT incidence). All comparisons between populations were performed as either 2‐tailed t tests or 2 analyses. All analysis was conducted using SAS software, version 9.2 (SAS Institute, Inc., Cary, NC).
RESULTS
For the 18‐month period between October 1, 2009, and March 31, 2011, across 83 UHC hospitals, there were 2,525,068 cases. Among these, 12,847 (0.51%) had 1 HA‐VTE/SVT coded. As per the clinical importance hierarchy described above, 2449 (19.1%) cases had at least a PE coded; 3848 (30%) had at least a LE‐DVT (but not a PE) coded; 2893 (22.5%) had at least an UE‐DVT coded; 3248 (25.3%) had at least an SVT coded; 30 had at least a chronic VTE coded; and 379 had at least a VTE coded with no specified location. Of those with SVT, 192 (5.8%) were LE‐SVT codes, whereas the rest were SVT/thrombophlebitis of the upper extremities or not otherwise specified. There were 11,882 (92.5%) hospitalizations with a single HA‐VTE/SVT code and an additional 965 (7.5%) with multiple codes, for a total of 13,749 HA‐VTE/SVT events (see Supporting Information, Table S1, in the online version of this article for more specific data for the individual ICD‐9 codes used to specify HA‐VTE events).
Compared with those who did not develop any HA‐VTE/SVT, patients with HA‐PE/LE‐DVT were more likely to be Caucasian (65% vs 58%, P < 0.001) and were older (age 62 vs 48 years, P < 0.001) and sicker (79.9% vs 44.9% with a severe or extreme SOI, P < 0.001). They also were more likely to have cancer, have longer lengths of stay, be more likely to stay in the ICU, and die in the hospital (P < 0.001 for all comparisons; Table 1).
Characteristic | No HA‐VTE, n = 2,512,221 | HA‐PE/LE DVT, n = 6,297a | P Valueb |
---|---|---|---|
| |||
Proportion of hospitalizations, % | 99.49 | 0.25 | |
Age, y | 48.2 27.1 | 62.5 20.0 | <0.001 |
Female sex | 1,347,219 (53.6) | 3,104 (49.3) | <0.001 |
Race | <0.001 | ||
Caucasian | 1,455,215 (57.9) | 3,963 (64.7) | |
Black | 600,991 (23.9) | 1,425 (23.3) | |
Hispanic | 206,553 (8.2) | 263 (4.3) | |
API | 59,560 (2.4) | 88 (1.4) | |
Other | 189,902 (7.6) | 389 (6.4) | |
Admission SOI | <0.001 | ||
Minor | 461,411 (18.4) | 181 (2.9) | |
Major | 922,734 (36.7) | 1,081 (17.2) | |
Severe | 880,542 (35.1) | 2,975 (47.2) | |
Extreme | 247,244 (9.8) | 2,060 (32.7) | |
Unknown | 290 (0.01) | 0 (0.0) | |
Had an active diagnosis of cancer | 331,705 (13.2) | 2,162 (34.3) | <0.001 |
Length of stay, d | 7.31 9.31 | 18.7 19.5 | <0.001 |
Spent time in the ICU | 441,412 (17.6) | 3,011 (47.8) | <0.001 |
Died in hospital | 57,954 (2.3) | 1,036 (16.5) | <0.001 |
Received prophylaxisc | c | 3,454 (54.9) | c |
Among cases with a code for UE‐DVT (22.5% of all patients with HA‐VTE), 74% were noted to also have a code for a CVC, as did 60% of cases with a HA‐SVT of the antecubital, basilic, or cephalic veins (71% of SVT events; see Supporting Information, Table S1, in the online version of this article).
Of those with HA‐PE/LE‐DVT, 54.9% received pharmacologic prophylaxis on hospital day 1 or 2 (mostly with low‐molecular‐weight heparin or unfractionated heparin).
DISCUSSION
In this study of medical patients admitted to academic medical centers throughout the United States, we found that HA‐VTE/SVT was coded in approximately 0.51% of discharges, and the incidence of HA‐PE/LE‐DVT was 0.25%. Patients with a HA‐PE/LE‐DVT code were, in general, older and sicker than those who did not develop VTE. We further found that close to half of all HA‐VTE/SVT occurred in the upper extremity, with the majority of these occurring in patients who had CVCs. Finally, the majority of patients diagnosed with HA‐PE/LE‐DVT were started on VTEP on the first or second hospital day.
The overall incidence of HA‐VTE/SVT we discovered corresponds well to other studies, even those with disparate populations. A single‐institution study found a HA‐VTE/SVT incidence of approximately 0.6% among hospitalized patients on medical and nonmedical services.[12] The study by Barba found a rate of 0.93%,[18] whereas the study by Lederle found a rate of approximately 1%.[19] Spyropolous found an HA‐VTE incidence of 0.55%.[11] Rothberg found a lower rate of 0.25% in his risk‐stratification study, though in the pre‐POA and preupdated code era.[20] Our findings extend and provide context for, in a much larger population, the results of these prior studies, and represent the first national examination of HA‐VTE/SVT in the setting of numerous quality‐improvement and other efforts to reduce hospital‐associated VTE.
The incidence of HA‐VTE/SVT codes we observed likely underestimates the incidence of hospital‐associated VTE/SVT by a factor of approximately 4, for 2 reasons. First, although VTE/SVT codes with a POA flag set to No are truly hospital‐acquired events on chart review approximately 75% of the time, and thus overestimate HA‐VTE/SVT, 25% of POA = Yes codes are actually HA‐VTE/SVT events on chart review, and therefore lead to underestimation of HA‐VTE/SVT.[9] Because VTE/SVT codes with a POA flag set to Yes outnumber those flagged No by 3 or 4 to 1, events mis‐flagged Yes contribute a much greater number of undercounted HA‐VTE/SVT, elevating the actual HA‐VTE/SVT event rate by a factor of approximately 2. Second, HA‐VTE events do not include hospital‐associated VTE events that are diagnosed after the index hospitalization. In the Spyropolous study, 45% of hospital‐associated VTE events occurred after discharge, so translating HA‐VTE/SVT events to hospital‐associated VTE/SVT events would again involve multiplying by a factor of 2.[11] Thus, the overall incidence of hospital‐associated VTE/SVT events in our sample may have been approximately 2% (0.51% 4), and the overall incidence of hospital‐associated PE or LE‐DVT events may have been approximately 1%, though there may be significant variation around these estimates given that individual institutions were themselves quite variable in their POA flag accuracy in our study.[9] There is additionally the possibility that hospitals may have deliberately left some VTE/SVT uncoded, but in the absence of financial incentives to do so for anything other than postsurgical VTE, and in the presence of penalties from CMS for undercoding, we believe this to be unlikely, at least at present.
Despite these upward extrapolations, the estimated incidence of hospital‐associated VTE/SVT in our study may seem low compared with that reported in the MEDENOX[2] and PREVENT studies.[3] Much of this discrepancy vanishes on closer examination. In the large randomized trials, patients were uniformly and routinely assessed for LE‐DVT using vascular ultrasound; in contrast, in our population of hospitalizations patients may have only had diagnostic studies done for signs or symptoms. Clinically apparent hospital‐associated VTE is less common than all hospital‐associated VTE, as it was even in PREVENT,[3] and increased surveillance may even be partially driving increased hospital‐associated VTE/SVT at some hospitals.[21] Our findings suggest that success or failure in preventing administratively coded, clinically apparent HA LE‐VTE/PE should be judged, broadly, against numbers in the range established in our study (eg, 0.25%), not the 5% or 15% of chart‐abstracted, aggressively ascertained (and sometimes clinically silent) hospital‐associated VTE in the large randomized controlled trials. That is, 0.25% is not an achievement, but rather the average, expected value.
Almost 25% of the observed HA‐VTE/SVTs coded were UE‐DVT, with roughly 75% of these being likely related to central venous catheterization (including those peripherally inserted). An additional 1/5 were upper‐extremity SVT of the antecubital, cephalic, and basilic veins, with the majority of these (60%) also listed as catheter‐related. Such thrombosis is best prevented by decreased use of central catheters or perhaps by using smaller‐caliber catheters.[22] It is unclear if VTEP can prevent such clots, though in cancer patients at least one recent trial seems promising.[23]
We found that patients with a coded HA‐PE/LE‐DVT were remarkably different from those not developing HA‐VTE/SVT. Patients with HA‐PE or HA‐LE‐DVT were older, sicker, more likely to have cancer, significantly more likely to spend time in the ICU, and much more likely to die in the hospital; risk factors for HA‐VTE overlap significantly with risk factors for death in the hospital. A small majority (55%) of patients in the HA‐PE/LE‐DVT group had actually received VTEP on at least day 1 or 2 of hospitalization. It may be the case that the dose of VTEP was insufficient to suppress clot formation in these patients, or that HA‐PE/LE‐DVT in patients with this degree of comorbidity is difficult to prevent.
There are a number of limitations to our study. We analyzed administrative codes, which underestimate hospital‐associated VTE/SVT events as noted above. This was a descriptive study, cross‐sectional across each hospitalization, and we were unable to draw any causal inference for differences in HA‐VTE/SVT incidence that might exist between subpopulations. We estimated VTEP from medication usage in just the first 2 days of hospitalization; we could not assess mechanical prophylaxis in this dataset; and we did not have any VTEP data for the first 2 days of hospitalization on the patients who did not develop a HA‐VTE/SVT, which made it impossible to compare the 2 populations on this measure. For those who did not receive VTEP, we were unable to obtain data regarding possible contraindications to VTEP, such as ongoing gastrointestinal or intracerebral hemorrhage. Additionally, our data are based on academic hospitals only and may not generalize to nonacademic settings. Extrapolating from HA‐VTE/SVT to hospital‐associated VTE/SVT may not be possible due to heterogeneity of clotting events and perhaps variability in whether patients would return to the hospital for all of them (eg, superficial or UE VTE may not result in readmission). Finally, it is unclear whether a switch to ICD Tenth Revision (ICD‐10) codes will impact our measured baseline in the coming year. The strengths of our analysis included stratification by type of HA‐VTE/SVT and our ability to assess the incidence of HA‐VTE/SVT in a large national population, and the provision of a baseline for VTE incidenceeasily usable by any individual hospital, network, or researcher with access to administrative datagoing forward.
In conclusion, among patients hospitalized in academic medical centers, HA‐VTE/SVT was coded in approximately 0.51% of patients with a medical illness staying >2 days, with approximately half of the events due to HA‐PE/LE‐DVT. Patients who developed HA‐PE/LE‐DVT were more acutely ill than those who did not, and VTE developed despite 55% of these patients receiving VTEP on day 1 or 2. Hospitals can reasonably treat the 0.25% figure as the baseline around which to assess their own performance in preventing HA‐PE/LE‐DVT, and can measure their own performance using administrative data. Further research is needed to determine how best to achieve further reductions in HA‐VTE/SVT through risk stratification and/or through other interventions.
Disclosures
Nothing to report.
- American College of Chest Physicians AntithromboticTherapy and Prevention of Thrombosis Panel. Executive summary: Antithrombotic Therapy and Prevention of Thrombosis, 9th ed: American College of Chest Physicians Evidence‐Based Clinical Practice Guidelines [published corrections appear in Chest. 2012;141(4):1129 and 2012;142(6):1698]. Chest. 2012;141(2 suppl):7S–47S. , , , , ;
- Prophylaxis in Medical Patients with Enoxaparin Study Group. A comparison of enoxaparin with placebo for the prevention of venous thromboembolism in acutely ill medical patients. N Engl J Med. 1999;341(11):793–800. , , , et al;
- Randomized, placebo‐controlled trial of dalteparin for the prevention of venous thromboembolism in acutely ill medical patients. Circulation. 2004;110(7):874–879. , , , , , .
- The Joint Commission.Specifications Manual for National Hospital Inpatient Quality Measures. Available at: http://www.jointcommission.org/specifications_manual_for_national_hospital_inpatient_quality_measures.aspx. Accessed July 18, 2012.
- Preventing Hospital‐Acquired Venous Thromboembolism: A Guide for Effective Quality Improvement. Prepared by the Society of Hospital Medicine. Rockville, MD: Agency for Healthcare Research and Quality; AHRQ Publication No. 08‐0075. , .
- Prevention of venous thromboembolism: the Seventh ACCP Conference on Antithrombotic and Thrombolytic Therapy. Chest. 2004;126(3 suppl):338S–400S. , , , et al.
- Prevention of VTE in nonsurgical patients: Antithrombotic Therapy and Prevention of Thrombosis, 9th ed: American College of Chest Physicians Evidence‐Based Clinical Practice Guidelines. Chest. 2012;141(2 suppl):e195S–e226S. , , , et al.
- Centers for Medicare 46(6 part 1):1946–1962.
- Predictive and associative models to identify hospitalized medical patients at risk for VTE. Chest. 2011;140(3):706–714. , , , et al.
- Unintended consequences of a standard admission order set on venous thromboembolism prophylaxis and patient outcomes. J Gen Intern Med. 2012;27(3):318–324. , , , .
- United Health Consortium Website. Available at: https://www.uhc.edu. Accessed March 8, 2012.
- ICD‐9‐CM Official Guidelines for Coding and Reporting. Available at: http://www.cdc.gov/nchs/data/icd9/icdguide10.pdf. Published 2010. Accessed June 4, 2013.
- Centers for Medicare 27(5):587–612.
- Overview of Disease Severity Measures Disseminated with the Nationwide Inpatient Sample (NIS) and Kids' Inpatient Database (KID). Available at: http://www.hcup‐us.ahrq.gov/db/nation/nis/OverviewofSeveritySystems.pdf. Published December 9, 2005. Accessed June 4, 2013.
- Venous thromboembolism in acutely ill hospitalized medical patients. Thromb Res. 2010;126(4):276–279. , , , et al.
- Venous thromboembolism prophylaxis in hospitalized medical patients and those with stroke: a background review for an American College of Physicians Clinical Practice Guideline. Ann Intern Med. 2011;155(9):602–615. , , , .
- Risk factor model to predict venous thromboembolism in hospitalized medical patients. J Hosp Med. 2011;6(4):202–209. , , , , .
- Evaluation of surveillance bias and the validity of the venous thromboembolism quality measure. JAMA. 2013;310(14):1482–1489. , , , et al.
- Reduction of peripherally inserted central catheter‐associated DVT. Chest. 2013;143(3):627–633. , , , et al.
- Prophylaxis of catheter‐related deep vein thrombosis in cancer patients with low‐dose warfarin, low molecular weight heparin, or control: a randomized, controlled, phase III study. Cancer Chemother Pharmacol. 2013;72(1):65–73. , , , et al.
Pulmonary embolism (PE) and deep venous thrombosis (DVT), historically referred to together as venous thromboembolism (VTE), are common, treatable, sometimes fatal, and potentially preventable medical problems.[1] Such thromboses can both precipitate a hospitalization as well as complicate it (either during or soon after discharge). Preventing such thrombosis as a complication of medical care has become a national imperative. Landmark studies such as Prophylaxis in Medical Patients With Enoxaparin (MEDENOX)[2] and Prospective Evaluation of Dalteparin Efficacy for Prevention of VTE in Immobilized Patients Trial (PREVENT)[3] demonstrated both a high incidence of thrombosis in a hospitalized high‐risk medical population (15% and 5% in the 2 trials' placebo arms, respectively) as well as significant relative risk reduction through venous thromboembolism pharmacoprophylaxis (VTEP)63% and 45%, respectively. The Joint Commission,[4] the Society of Hospital Medicine,[5] and the American College of Chest Physicians[6, 7] have thus all strived to ensure the appropriate provision of VTEP in order to reduce the morbidity and mortality associated with thrombosis in hospitalized patients, including those on medical services.
Ideally, the global success of these efforts would be assessed by measuring the rate of hospital‐associated VTE (potentially including superficial venous thrombosis [SVT], which, like upper‐extremity deep venous thrombosis [UE‐DVT], is commonly a central venous catheter [CVC]‐associated, or peripherally inserted central catheter [PICC]‐associated, complication)thrombosis acquired and diagnosed during either the index hospitalization (hospital‐acquired, or HA‐VTE/SVT) or up to 30 days postdischarge. Unfortunately, postdischarge VTE/SVT is difficult to measure because patients developing it may not present to the original hospital, or at all (eg, if they do not seek care, are treated as outpatients, or, in the most extreme case, die at home). In this context, despite being far less comprehensive, HA‐VTE/SVT is a useful subset of hospital‐associated VTE/SVT, for several reasons. First, the Centers for Medicare & Medicaid Services (CMS) have mandated hospitals to qualify all medical diagnoses as present‐on‐admission (POA = Y) or not (POA = N) since 2008, such that all medical diagnoses coded POA = N can be considered hospital acquired.[8] Second, refinements made to the International Classification of Diseases, 9th Revision (ICD‐9) codes now allow differentiation of UE‐DVT and SVT from lower‐extremity (LE) DVT/PE, whereas the former were sometimes obscured by nonspecific coding.[9] Third, recent studies have shown that medical diagnoses administratively coded as HA‐VTE/SVT correlated well with HA‐VTE/SVT ascertained through chart review.[9, 10] Finally, previous work has estimated that approximately half of all hospital‐associated VTE are HA‐VTE and the other half are postdischarge VTE.[11] Thus, HA‐VTE, though comprising only approximately half of all hospital‐associated VTE, is often used as a surrogate for measuring the success of ongoing VTE prevention programs.[12]
Our study aimed to assess the incidence of HA‐VTE plus HA‐SVT in the era of mandatory POA coding and newer ICD‐9 codes for VTE.
METHODS
Setting and Cases
We conducted a retrospective analysis of discharges from the 83 academic medical centers belonging to the UHC (formerly, the University HealthSystem Consortium,
Patients in our analysis were age 18 years and discharged with a medical medical severity diagnostic‐related group (MS‐DRG) code, hospitalized for 48 hours, and did not have a surgical or obstetric MS‐DRG code (except when assigned a surgical MS‐DRG code solely due to insertion of an inferior vena cava filter, with no other major procedures performed). Cases excluded discharges with a principal diagnosis of acute VTE/SVT (defined here as including PE, LE‐DVT, UE‐DVT, SVT, chronic VTE, and thrombosis not otherwise specified), as coding guidelines prohibit assigning a HA‐VTE as the principal diagnosis for the index hospitalization.[14]
Hospital‐Acquired Venous Thromboembolism or Superficial Venous Thrombosis
Cases were classified as having a HA‐VTE/SVT if there was 1 VTE/SVT coded in a secondary diagnosis position (other diagnosis) with a corresponding POA indicator equal to either N (not POA) or U (documentation insufficient to clarify whether VTE was POA or not). This usage corresponds to CMS guidelines and reimbursement policies for hospital‐acquired conditions.[15] Among cases with 1 HA‐VTE (or SVT), we assigned 1 HA‐VTE diagnosis using a hierarchy based on the highest level of clinical importance: first, PE; then LE‐DVT; then UE‐DVT; then SVT; then chronic VTE; then, finally, unspecified VTE. We subsequently excluded cases with primarily chronic VTE from our analysis because these were likely miscodes (ie, it is unclear how a chronic VTE could not be POA) and there were only 30 such cases. Cases with HA‐PE or HA‐LE DVT were analyzed separately as an important subset of HA‐VTE (plus SVT), because HA‐PE/LE‐DVT is both life‐threatening and theoretically preventable with VTEP.
Severity of Illness and Other Measures of Comorbidity
For each case we used proprietary software (3M Health Information Systems, Murray UT) to classify severity of illness (SOI). The SOI scale, based on physiologic derangement and organ system loss of function,[16] has 4 levels: minor, major, severe, and extreme. Defined within specific disease groups (All Patient Refined DRGs), it is often compared across diseases as well.[17] We also assessed whether patients had a cancer diagnosis, spent time in the intensive care unit (ICU), and died in the hospital.
Central Venous Catheter Use in Patients With Upper‐Extremity Deep Venous Thrombosis or Superficial Venous Thrombosis
Because UE‐DVT and SVT are frequently associated with a CVC or PICC, we assessed central venous catheterization among patients with an UE‐DVT or SVT of the cephalic, basilic, or antecubital veins using diagnosis codes for complications related to dialysis devices, implants, and grafts.
Pharmacologic Thromboprophylaxis
Pharmacy records of the subset of HA‐VTE/SVT cases with PE or LE‐DVT were analyzed to determine if VTEP was administered on hospital day 1 or 2, as per Joint Commission performance requirements.[4] Medications that met criteria as VTEP included unfractionated heparin, 5000 IU, given 2 or 3 a day; enoxaparin, 40 mg, given daily; dalteparin, 2500 or 5000 IU, given daily; fondaparinux, 2.5 mg, given daily; and warfarin. We could not reliably determine if VTEP was used throughout the entire hospitalization, or whether mechanical prophylaxis was used at all.
Statistical Analysis
This was a descriptive analysis to determine the incidence of HA‐VTE/SVT and describe the demographic and clinical characteristics of this population. We calculated means and standard deviations (SD) for continuous variables and proportions for binary variables (including HA‐VTE/SVT incidence). All comparisons between populations were performed as either 2‐tailed t tests or 2 analyses. All analysis was conducted using SAS software, version 9.2 (SAS Institute, Inc., Cary, NC).
RESULTS
For the 18‐month period between October 1, 2009, and March 31, 2011, across 83 UHC hospitals, there were 2,525,068 cases. Among these, 12,847 (0.51%) had 1 HA‐VTE/SVT coded. As per the clinical importance hierarchy described above, 2449 (19.1%) cases had at least a PE coded; 3848 (30%) had at least a LE‐DVT (but not a PE) coded; 2893 (22.5%) had at least an UE‐DVT coded; 3248 (25.3%) had at least an SVT coded; 30 had at least a chronic VTE coded; and 379 had at least a VTE coded with no specified location. Of those with SVT, 192 (5.8%) were LE‐SVT codes, whereas the rest were SVT/thrombophlebitis of the upper extremities or not otherwise specified. There were 11,882 (92.5%) hospitalizations with a single HA‐VTE/SVT code and an additional 965 (7.5%) with multiple codes, for a total of 13,749 HA‐VTE/SVT events (see Supporting Information, Table S1, in the online version of this article for more specific data for the individual ICD‐9 codes used to specify HA‐VTE events).
Compared with those who did not develop any HA‐VTE/SVT, patients with HA‐PE/LE‐DVT were more likely to be Caucasian (65% vs 58%, P < 0.001) and were older (age 62 vs 48 years, P < 0.001) and sicker (79.9% vs 44.9% with a severe or extreme SOI, P < 0.001). They also were more likely to have cancer, have longer lengths of stay, be more likely to stay in the ICU, and die in the hospital (P < 0.001 for all comparisons; Table 1).
Characteristic | No HA‐VTE, n = 2,512,221 | HA‐PE/LE DVT, n = 6,297a | P Valueb |
---|---|---|---|
| |||
Proportion of hospitalizations, % | 99.49 | 0.25 | |
Age, y | 48.2 27.1 | 62.5 20.0 | <0.001 |
Female sex | 1,347,219 (53.6) | 3,104 (49.3) | <0.001 |
Race | <0.001 | ||
Caucasian | 1,455,215 (57.9) | 3,963 (64.7) | |
Black | 600,991 (23.9) | 1,425 (23.3) | |
Hispanic | 206,553 (8.2) | 263 (4.3) | |
API | 59,560 (2.4) | 88 (1.4) | |
Other | 189,902 (7.6) | 389 (6.4) | |
Admission SOI | <0.001 | ||
Minor | 461,411 (18.4) | 181 (2.9) | |
Major | 922,734 (36.7) | 1,081 (17.2) | |
Severe | 880,542 (35.1) | 2,975 (47.2) | |
Extreme | 247,244 (9.8) | 2,060 (32.7) | |
Unknown | 290 (0.01) | 0 (0.0) | |
Had an active diagnosis of cancer | 331,705 (13.2) | 2,162 (34.3) | <0.001 |
Length of stay, d | 7.31 9.31 | 18.7 19.5 | <0.001 |
Spent time in the ICU | 441,412 (17.6) | 3,011 (47.8) | <0.001 |
Died in hospital | 57,954 (2.3) | 1,036 (16.5) | <0.001 |
Received prophylaxisc | c | 3,454 (54.9) | c |
Among cases with a code for UE‐DVT (22.5% of all patients with HA‐VTE), 74% were noted to also have a code for a CVC, as did 60% of cases with a HA‐SVT of the antecubital, basilic, or cephalic veins (71% of SVT events; see Supporting Information, Table S1, in the online version of this article).
Of those with HA‐PE/LE‐DVT, 54.9% received pharmacologic prophylaxis on hospital day 1 or 2 (mostly with low‐molecular‐weight heparin or unfractionated heparin).
DISCUSSION
In this study of medical patients admitted to academic medical centers throughout the United States, we found that HA‐VTE/SVT was coded in approximately 0.51% of discharges, and the incidence of HA‐PE/LE‐DVT was 0.25%. Patients with a HA‐PE/LE‐DVT code were, in general, older and sicker than those who did not develop VTE. We further found that close to half of all HA‐VTE/SVT occurred in the upper extremity, with the majority of these occurring in patients who had CVCs. Finally, the majority of patients diagnosed with HA‐PE/LE‐DVT were started on VTEP on the first or second hospital day.
The overall incidence of HA‐VTE/SVT we discovered corresponds well to other studies, even those with disparate populations. A single‐institution study found a HA‐VTE/SVT incidence of approximately 0.6% among hospitalized patients on medical and nonmedical services.[12] The study by Barba found a rate of 0.93%,[18] whereas the study by Lederle found a rate of approximately 1%.[19] Spyropolous found an HA‐VTE incidence of 0.55%.[11] Rothberg found a lower rate of 0.25% in his risk‐stratification study, though in the pre‐POA and preupdated code era.[20] Our findings extend and provide context for, in a much larger population, the results of these prior studies, and represent the first national examination of HA‐VTE/SVT in the setting of numerous quality‐improvement and other efforts to reduce hospital‐associated VTE.
The incidence of HA‐VTE/SVT codes we observed likely underestimates the incidence of hospital‐associated VTE/SVT by a factor of approximately 4, for 2 reasons. First, although VTE/SVT codes with a POA flag set to No are truly hospital‐acquired events on chart review approximately 75% of the time, and thus overestimate HA‐VTE/SVT, 25% of POA = Yes codes are actually HA‐VTE/SVT events on chart review, and therefore lead to underestimation of HA‐VTE/SVT.[9] Because VTE/SVT codes with a POA flag set to Yes outnumber those flagged No by 3 or 4 to 1, events mis‐flagged Yes contribute a much greater number of undercounted HA‐VTE/SVT, elevating the actual HA‐VTE/SVT event rate by a factor of approximately 2. Second, HA‐VTE events do not include hospital‐associated VTE events that are diagnosed after the index hospitalization. In the Spyropolous study, 45% of hospital‐associated VTE events occurred after discharge, so translating HA‐VTE/SVT events to hospital‐associated VTE/SVT events would again involve multiplying by a factor of 2.[11] Thus, the overall incidence of hospital‐associated VTE/SVT events in our sample may have been approximately 2% (0.51% 4), and the overall incidence of hospital‐associated PE or LE‐DVT events may have been approximately 1%, though there may be significant variation around these estimates given that individual institutions were themselves quite variable in their POA flag accuracy in our study.[9] There is additionally the possibility that hospitals may have deliberately left some VTE/SVT uncoded, but in the absence of financial incentives to do so for anything other than postsurgical VTE, and in the presence of penalties from CMS for undercoding, we believe this to be unlikely, at least at present.
Despite these upward extrapolations, the estimated incidence of hospital‐associated VTE/SVT in our study may seem low compared with that reported in the MEDENOX[2] and PREVENT studies.[3] Much of this discrepancy vanishes on closer examination. In the large randomized trials, patients were uniformly and routinely assessed for LE‐DVT using vascular ultrasound; in contrast, in our population of hospitalizations patients may have only had diagnostic studies done for signs or symptoms. Clinically apparent hospital‐associated VTE is less common than all hospital‐associated VTE, as it was even in PREVENT,[3] and increased surveillance may even be partially driving increased hospital‐associated VTE/SVT at some hospitals.[21] Our findings suggest that success or failure in preventing administratively coded, clinically apparent HA LE‐VTE/PE should be judged, broadly, against numbers in the range established in our study (eg, 0.25%), not the 5% or 15% of chart‐abstracted, aggressively ascertained (and sometimes clinically silent) hospital‐associated VTE in the large randomized controlled trials. That is, 0.25% is not an achievement, but rather the average, expected value.
Almost 25% of the observed HA‐VTE/SVTs coded were UE‐DVT, with roughly 75% of these being likely related to central venous catheterization (including those peripherally inserted). An additional 1/5 were upper‐extremity SVT of the antecubital, cephalic, and basilic veins, with the majority of these (60%) also listed as catheter‐related. Such thrombosis is best prevented by decreased use of central catheters or perhaps by using smaller‐caliber catheters.[22] It is unclear if VTEP can prevent such clots, though in cancer patients at least one recent trial seems promising.[23]
We found that patients with a coded HA‐PE/LE‐DVT were remarkably different from those not developing HA‐VTE/SVT. Patients with HA‐PE or HA‐LE‐DVT were older, sicker, more likely to have cancer, significantly more likely to spend time in the ICU, and much more likely to die in the hospital; risk factors for HA‐VTE overlap significantly with risk factors for death in the hospital. A small majority (55%) of patients in the HA‐PE/LE‐DVT group had actually received VTEP on at least day 1 or 2 of hospitalization. It may be the case that the dose of VTEP was insufficient to suppress clot formation in these patients, or that HA‐PE/LE‐DVT in patients with this degree of comorbidity is difficult to prevent.
There are a number of limitations to our study. We analyzed administrative codes, which underestimate hospital‐associated VTE/SVT events as noted above. This was a descriptive study, cross‐sectional across each hospitalization, and we were unable to draw any causal inference for differences in HA‐VTE/SVT incidence that might exist between subpopulations. We estimated VTEP from medication usage in just the first 2 days of hospitalization; we could not assess mechanical prophylaxis in this dataset; and we did not have any VTEP data for the first 2 days of hospitalization on the patients who did not develop a HA‐VTE/SVT, which made it impossible to compare the 2 populations on this measure. For those who did not receive VTEP, we were unable to obtain data regarding possible contraindications to VTEP, such as ongoing gastrointestinal or intracerebral hemorrhage. Additionally, our data are based on academic hospitals only and may not generalize to nonacademic settings. Extrapolating from HA‐VTE/SVT to hospital‐associated VTE/SVT may not be possible due to heterogeneity of clotting events and perhaps variability in whether patients would return to the hospital for all of them (eg, superficial or UE VTE may not result in readmission). Finally, it is unclear whether a switch to ICD Tenth Revision (ICD‐10) codes will impact our measured baseline in the coming year. The strengths of our analysis included stratification by type of HA‐VTE/SVT and our ability to assess the incidence of HA‐VTE/SVT in a large national population, and the provision of a baseline for VTE incidenceeasily usable by any individual hospital, network, or researcher with access to administrative datagoing forward.
In conclusion, among patients hospitalized in academic medical centers, HA‐VTE/SVT was coded in approximately 0.51% of patients with a medical illness staying >2 days, with approximately half of the events due to HA‐PE/LE‐DVT. Patients who developed HA‐PE/LE‐DVT were more acutely ill than those who did not, and VTE developed despite 55% of these patients receiving VTEP on day 1 or 2. Hospitals can reasonably treat the 0.25% figure as the baseline around which to assess their own performance in preventing HA‐PE/LE‐DVT, and can measure their own performance using administrative data. Further research is needed to determine how best to achieve further reductions in HA‐VTE/SVT through risk stratification and/or through other interventions.
Disclosures
Nothing to report.
Pulmonary embolism (PE) and deep venous thrombosis (DVT), historically referred to together as venous thromboembolism (VTE), are common, treatable, sometimes fatal, and potentially preventable medical problems.[1] Such thromboses can both precipitate a hospitalization as well as complicate it (either during or soon after discharge). Preventing such thrombosis as a complication of medical care has become a national imperative. Landmark studies such as Prophylaxis in Medical Patients With Enoxaparin (MEDENOX)[2] and Prospective Evaluation of Dalteparin Efficacy for Prevention of VTE in Immobilized Patients Trial (PREVENT)[3] demonstrated both a high incidence of thrombosis in a hospitalized high‐risk medical population (15% and 5% in the 2 trials' placebo arms, respectively) as well as significant relative risk reduction through venous thromboembolism pharmacoprophylaxis (VTEP)63% and 45%, respectively. The Joint Commission,[4] the Society of Hospital Medicine,[5] and the American College of Chest Physicians[6, 7] have thus all strived to ensure the appropriate provision of VTEP in order to reduce the morbidity and mortality associated with thrombosis in hospitalized patients, including those on medical services.
Ideally, the global success of these efforts would be assessed by measuring the rate of hospital‐associated VTE (potentially including superficial venous thrombosis [SVT], which, like upper‐extremity deep venous thrombosis [UE‐DVT], is commonly a central venous catheter [CVC]‐associated, or peripherally inserted central catheter [PICC]‐associated, complication)thrombosis acquired and diagnosed during either the index hospitalization (hospital‐acquired, or HA‐VTE/SVT) or up to 30 days postdischarge. Unfortunately, postdischarge VTE/SVT is difficult to measure because patients developing it may not present to the original hospital, or at all (eg, if they do not seek care, are treated as outpatients, or, in the most extreme case, die at home). In this context, despite being far less comprehensive, HA‐VTE/SVT is a useful subset of hospital‐associated VTE/SVT, for several reasons. First, the Centers for Medicare & Medicaid Services (CMS) have mandated hospitals to qualify all medical diagnoses as present‐on‐admission (POA = Y) or not (POA = N) since 2008, such that all medical diagnoses coded POA = N can be considered hospital acquired.[8] Second, refinements made to the International Classification of Diseases, 9th Revision (ICD‐9) codes now allow differentiation of UE‐DVT and SVT from lower‐extremity (LE) DVT/PE, whereas the former were sometimes obscured by nonspecific coding.[9] Third, recent studies have shown that medical diagnoses administratively coded as HA‐VTE/SVT correlated well with HA‐VTE/SVT ascertained through chart review.[9, 10] Finally, previous work has estimated that approximately half of all hospital‐associated VTE are HA‐VTE and the other half are postdischarge VTE.[11] Thus, HA‐VTE, though comprising only approximately half of all hospital‐associated VTE, is often used as a surrogate for measuring the success of ongoing VTE prevention programs.[12]
Our study aimed to assess the incidence of HA‐VTE plus HA‐SVT in the era of mandatory POA coding and newer ICD‐9 codes for VTE.
METHODS
Setting and Cases
We conducted a retrospective analysis of discharges from the 83 academic medical centers belonging to the UHC (formerly, the University HealthSystem Consortium,
Patients in our analysis were age 18 years and discharged with a medical medical severity diagnostic‐related group (MS‐DRG) code, hospitalized for 48 hours, and did not have a surgical or obstetric MS‐DRG code (except when assigned a surgical MS‐DRG code solely due to insertion of an inferior vena cava filter, with no other major procedures performed). Cases excluded discharges with a principal diagnosis of acute VTE/SVT (defined here as including PE, LE‐DVT, UE‐DVT, SVT, chronic VTE, and thrombosis not otherwise specified), as coding guidelines prohibit assigning a HA‐VTE as the principal diagnosis for the index hospitalization.[14]
Hospital‐Acquired Venous Thromboembolism or Superficial Venous Thrombosis
Cases were classified as having a HA‐VTE/SVT if there was 1 VTE/SVT coded in a secondary diagnosis position (other diagnosis) with a corresponding POA indicator equal to either N (not POA) or U (documentation insufficient to clarify whether VTE was POA or not). This usage corresponds to CMS guidelines and reimbursement policies for hospital‐acquired conditions.[15] Among cases with 1 HA‐VTE (or SVT), we assigned 1 HA‐VTE diagnosis using a hierarchy based on the highest level of clinical importance: first, PE; then LE‐DVT; then UE‐DVT; then SVT; then chronic VTE; then, finally, unspecified VTE. We subsequently excluded cases with primarily chronic VTE from our analysis because these were likely miscodes (ie, it is unclear how a chronic VTE could not be POA) and there were only 30 such cases. Cases with HA‐PE or HA‐LE DVT were analyzed separately as an important subset of HA‐VTE (plus SVT), because HA‐PE/LE‐DVT is both life‐threatening and theoretically preventable with VTEP.
Severity of Illness and Other Measures of Comorbidity
For each case we used proprietary software (3M Health Information Systems, Murray UT) to classify severity of illness (SOI). The SOI scale, based on physiologic derangement and organ system loss of function,[16] has 4 levels: minor, major, severe, and extreme. Defined within specific disease groups (All Patient Refined DRGs), it is often compared across diseases as well.[17] We also assessed whether patients had a cancer diagnosis, spent time in the intensive care unit (ICU), and died in the hospital.
Central Venous Catheter Use in Patients With Upper‐Extremity Deep Venous Thrombosis or Superficial Venous Thrombosis
Because UE‐DVT and SVT are frequently associated with a CVC or PICC, we assessed central venous catheterization among patients with an UE‐DVT or SVT of the cephalic, basilic, or antecubital veins using diagnosis codes for complications related to dialysis devices, implants, and grafts.
Pharmacologic Thromboprophylaxis
Pharmacy records of the subset of HA‐VTE/SVT cases with PE or LE‐DVT were analyzed to determine if VTEP was administered on hospital day 1 or 2, as per Joint Commission performance requirements.[4] Medications that met criteria as VTEP included unfractionated heparin, 5000 IU, given 2 or 3 a day; enoxaparin, 40 mg, given daily; dalteparin, 2500 or 5000 IU, given daily; fondaparinux, 2.5 mg, given daily; and warfarin. We could not reliably determine if VTEP was used throughout the entire hospitalization, or whether mechanical prophylaxis was used at all.
Statistical Analysis
This was a descriptive analysis to determine the incidence of HA‐VTE/SVT and describe the demographic and clinical characteristics of this population. We calculated means and standard deviations (SD) for continuous variables and proportions for binary variables (including HA‐VTE/SVT incidence). All comparisons between populations were performed as either 2‐tailed t tests or 2 analyses. All analysis was conducted using SAS software, version 9.2 (SAS Institute, Inc., Cary, NC).
RESULTS
For the 18‐month period between October 1, 2009, and March 31, 2011, across 83 UHC hospitals, there were 2,525,068 cases. Among these, 12,847 (0.51%) had 1 HA‐VTE/SVT coded. As per the clinical importance hierarchy described above, 2449 (19.1%) cases had at least a PE coded; 3848 (30%) had at least a LE‐DVT (but not a PE) coded; 2893 (22.5%) had at least an UE‐DVT coded; 3248 (25.3%) had at least an SVT coded; 30 had at least a chronic VTE coded; and 379 had at least a VTE coded with no specified location. Of those with SVT, 192 (5.8%) were LE‐SVT codes, whereas the rest were SVT/thrombophlebitis of the upper extremities or not otherwise specified. There were 11,882 (92.5%) hospitalizations with a single HA‐VTE/SVT code and an additional 965 (7.5%) with multiple codes, for a total of 13,749 HA‐VTE/SVT events (see Supporting Information, Table S1, in the online version of this article for more specific data for the individual ICD‐9 codes used to specify HA‐VTE events).
Compared with those who did not develop any HA‐VTE/SVT, patients with HA‐PE/LE‐DVT were more likely to be Caucasian (65% vs 58%, P < 0.001) and were older (age 62 vs 48 years, P < 0.001) and sicker (79.9% vs 44.9% with a severe or extreme SOI, P < 0.001). They also were more likely to have cancer, have longer lengths of stay, be more likely to stay in the ICU, and die in the hospital (P < 0.001 for all comparisons; Table 1).
Characteristic | No HA‐VTE, n = 2,512,221 | HA‐PE/LE DVT, n = 6,297a | P Valueb |
---|---|---|---|
| |||
Proportion of hospitalizations, % | 99.49 | 0.25 | |
Age, y | 48.2 27.1 | 62.5 20.0 | <0.001 |
Female sex | 1,347,219 (53.6) | 3,104 (49.3) | <0.001 |
Race | <0.001 | ||
Caucasian | 1,455,215 (57.9) | 3,963 (64.7) | |
Black | 600,991 (23.9) | 1,425 (23.3) | |
Hispanic | 206,553 (8.2) | 263 (4.3) | |
API | 59,560 (2.4) | 88 (1.4) | |
Other | 189,902 (7.6) | 389 (6.4) | |
Admission SOI | <0.001 | ||
Minor | 461,411 (18.4) | 181 (2.9) | |
Major | 922,734 (36.7) | 1,081 (17.2) | |
Severe | 880,542 (35.1) | 2,975 (47.2) | |
Extreme | 247,244 (9.8) | 2,060 (32.7) | |
Unknown | 290 (0.01) | 0 (0.0) | |
Had an active diagnosis of cancer | 331,705 (13.2) | 2,162 (34.3) | <0.001 |
Length of stay, d | 7.31 9.31 | 18.7 19.5 | <0.001 |
Spent time in the ICU | 441,412 (17.6) | 3,011 (47.8) | <0.001 |
Died in hospital | 57,954 (2.3) | 1,036 (16.5) | <0.001 |
Received prophylaxisc | c | 3,454 (54.9) | c |
Among cases with a code for UE‐DVT (22.5% of all patients with HA‐VTE), 74% were noted to also have a code for a CVC, as did 60% of cases with a HA‐SVT of the antecubital, basilic, or cephalic veins (71% of SVT events; see Supporting Information, Table S1, in the online version of this article).
Of those with HA‐PE/LE‐DVT, 54.9% received pharmacologic prophylaxis on hospital day 1 or 2 (mostly with low‐molecular‐weight heparin or unfractionated heparin).
DISCUSSION
In this study of medical patients admitted to academic medical centers throughout the United States, we found that HA‐VTE/SVT was coded in approximately 0.51% of discharges, and the incidence of HA‐PE/LE‐DVT was 0.25%. Patients with a HA‐PE/LE‐DVT code were, in general, older and sicker than those who did not develop VTE. We further found that close to half of all HA‐VTE/SVT occurred in the upper extremity, with the majority of these occurring in patients who had CVCs. Finally, the majority of patients diagnosed with HA‐PE/LE‐DVT were started on VTEP on the first or second hospital day.
The overall incidence of HA‐VTE/SVT we discovered corresponds well to other studies, even those with disparate populations. A single‐institution study found a HA‐VTE/SVT incidence of approximately 0.6% among hospitalized patients on medical and nonmedical services.[12] The study by Barba found a rate of 0.93%,[18] whereas the study by Lederle found a rate of approximately 1%.[19] Spyropolous found an HA‐VTE incidence of 0.55%.[11] Rothberg found a lower rate of 0.25% in his risk‐stratification study, though in the pre‐POA and preupdated code era.[20] Our findings extend and provide context for, in a much larger population, the results of these prior studies, and represent the first national examination of HA‐VTE/SVT in the setting of numerous quality‐improvement and other efforts to reduce hospital‐associated VTE.
The incidence of HA‐VTE/SVT codes we observed likely underestimates the incidence of hospital‐associated VTE/SVT by a factor of approximately 4, for 2 reasons. First, although VTE/SVT codes with a POA flag set to No are truly hospital‐acquired events on chart review approximately 75% of the time, and thus overestimate HA‐VTE/SVT, 25% of POA = Yes codes are actually HA‐VTE/SVT events on chart review, and therefore lead to underestimation of HA‐VTE/SVT.[9] Because VTE/SVT codes with a POA flag set to Yes outnumber those flagged No by 3 or 4 to 1, events mis‐flagged Yes contribute a much greater number of undercounted HA‐VTE/SVT, elevating the actual HA‐VTE/SVT event rate by a factor of approximately 2. Second, HA‐VTE events do not include hospital‐associated VTE events that are diagnosed after the index hospitalization. In the Spyropolous study, 45% of hospital‐associated VTE events occurred after discharge, so translating HA‐VTE/SVT events to hospital‐associated VTE/SVT events would again involve multiplying by a factor of 2.[11] Thus, the overall incidence of hospital‐associated VTE/SVT events in our sample may have been approximately 2% (0.51% 4), and the overall incidence of hospital‐associated PE or LE‐DVT events may have been approximately 1%, though there may be significant variation around these estimates given that individual institutions were themselves quite variable in their POA flag accuracy in our study.[9] There is additionally the possibility that hospitals may have deliberately left some VTE/SVT uncoded, but in the absence of financial incentives to do so for anything other than postsurgical VTE, and in the presence of penalties from CMS for undercoding, we believe this to be unlikely, at least at present.
Despite these upward extrapolations, the estimated incidence of hospital‐associated VTE/SVT in our study may seem low compared with that reported in the MEDENOX[2] and PREVENT studies.[3] Much of this discrepancy vanishes on closer examination. In the large randomized trials, patients were uniformly and routinely assessed for LE‐DVT using vascular ultrasound; in contrast, in our population of hospitalizations patients may have only had diagnostic studies done for signs or symptoms. Clinically apparent hospital‐associated VTE is less common than all hospital‐associated VTE, as it was even in PREVENT,[3] and increased surveillance may even be partially driving increased hospital‐associated VTE/SVT at some hospitals.[21] Our findings suggest that success or failure in preventing administratively coded, clinically apparent HA LE‐VTE/PE should be judged, broadly, against numbers in the range established in our study (eg, 0.25%), not the 5% or 15% of chart‐abstracted, aggressively ascertained (and sometimes clinically silent) hospital‐associated VTE in the large randomized controlled trials. That is, 0.25% is not an achievement, but rather the average, expected value.
Almost 25% of the observed HA‐VTE/SVTs coded were UE‐DVT, with roughly 75% of these being likely related to central venous catheterization (including those peripherally inserted). An additional 1/5 were upper‐extremity SVT of the antecubital, cephalic, and basilic veins, with the majority of these (60%) also listed as catheter‐related. Such thrombosis is best prevented by decreased use of central catheters or perhaps by using smaller‐caliber catheters.[22] It is unclear if VTEP can prevent such clots, though in cancer patients at least one recent trial seems promising.[23]
We found that patients with a coded HA‐PE/LE‐DVT were remarkably different from those not developing HA‐VTE/SVT. Patients with HA‐PE or HA‐LE‐DVT were older, sicker, more likely to have cancer, significantly more likely to spend time in the ICU, and much more likely to die in the hospital; risk factors for HA‐VTE overlap significantly with risk factors for death in the hospital. A small majority (55%) of patients in the HA‐PE/LE‐DVT group had actually received VTEP on at least day 1 or 2 of hospitalization. It may be the case that the dose of VTEP was insufficient to suppress clot formation in these patients, or that HA‐PE/LE‐DVT in patients with this degree of comorbidity is difficult to prevent.
There are a number of limitations to our study. We analyzed administrative codes, which underestimate hospital‐associated VTE/SVT events as noted above. This was a descriptive study, cross‐sectional across each hospitalization, and we were unable to draw any causal inference for differences in HA‐VTE/SVT incidence that might exist between subpopulations. We estimated VTEP from medication usage in just the first 2 days of hospitalization; we could not assess mechanical prophylaxis in this dataset; and we did not have any VTEP data for the first 2 days of hospitalization on the patients who did not develop a HA‐VTE/SVT, which made it impossible to compare the 2 populations on this measure. For those who did not receive VTEP, we were unable to obtain data regarding possible contraindications to VTEP, such as ongoing gastrointestinal or intracerebral hemorrhage. Additionally, our data are based on academic hospitals only and may not generalize to nonacademic settings. Extrapolating from HA‐VTE/SVT to hospital‐associated VTE/SVT may not be possible due to heterogeneity of clotting events and perhaps variability in whether patients would return to the hospital for all of them (eg, superficial or UE VTE may not result in readmission). Finally, it is unclear whether a switch to ICD Tenth Revision (ICD‐10) codes will impact our measured baseline in the coming year. The strengths of our analysis included stratification by type of HA‐VTE/SVT and our ability to assess the incidence of HA‐VTE/SVT in a large national population, and the provision of a baseline for VTE incidenceeasily usable by any individual hospital, network, or researcher with access to administrative datagoing forward.
In conclusion, among patients hospitalized in academic medical centers, HA‐VTE/SVT was coded in approximately 0.51% of patients with a medical illness staying >2 days, with approximately half of the events due to HA‐PE/LE‐DVT. Patients who developed HA‐PE/LE‐DVT were more acutely ill than those who did not, and VTE developed despite 55% of these patients receiving VTEP on day 1 or 2. Hospitals can reasonably treat the 0.25% figure as the baseline around which to assess their own performance in preventing HA‐PE/LE‐DVT, and can measure their own performance using administrative data. Further research is needed to determine how best to achieve further reductions in HA‐VTE/SVT through risk stratification and/or through other interventions.
Disclosures
Nothing to report.
- American College of Chest Physicians AntithromboticTherapy and Prevention of Thrombosis Panel. Executive summary: Antithrombotic Therapy and Prevention of Thrombosis, 9th ed: American College of Chest Physicians Evidence‐Based Clinical Practice Guidelines [published corrections appear in Chest. 2012;141(4):1129 and 2012;142(6):1698]. Chest. 2012;141(2 suppl):7S–47S. , , , , ;
- Prophylaxis in Medical Patients with Enoxaparin Study Group. A comparison of enoxaparin with placebo for the prevention of venous thromboembolism in acutely ill medical patients. N Engl J Med. 1999;341(11):793–800. , , , et al;
- Randomized, placebo‐controlled trial of dalteparin for the prevention of venous thromboembolism in acutely ill medical patients. Circulation. 2004;110(7):874–879. , , , , , .
- The Joint Commission.Specifications Manual for National Hospital Inpatient Quality Measures. Available at: http://www.jointcommission.org/specifications_manual_for_national_hospital_inpatient_quality_measures.aspx. Accessed July 18, 2012.
- Preventing Hospital‐Acquired Venous Thromboembolism: A Guide for Effective Quality Improvement. Prepared by the Society of Hospital Medicine. Rockville, MD: Agency for Healthcare Research and Quality; AHRQ Publication No. 08‐0075. , .
- Prevention of venous thromboembolism: the Seventh ACCP Conference on Antithrombotic and Thrombolytic Therapy. Chest. 2004;126(3 suppl):338S–400S. , , , et al.
- Prevention of VTE in nonsurgical patients: Antithrombotic Therapy and Prevention of Thrombosis, 9th ed: American College of Chest Physicians Evidence‐Based Clinical Practice Guidelines. Chest. 2012;141(2 suppl):e195S–e226S. , , , et al.
- Centers for Medicare 46(6 part 1):1946–1962.
- Predictive and associative models to identify hospitalized medical patients at risk for VTE. Chest. 2011;140(3):706–714. , , , et al.
- Unintended consequences of a standard admission order set on venous thromboembolism prophylaxis and patient outcomes. J Gen Intern Med. 2012;27(3):318–324. , , , .
- United Health Consortium Website. Available at: https://www.uhc.edu. Accessed March 8, 2012.
- ICD‐9‐CM Official Guidelines for Coding and Reporting. Available at: http://www.cdc.gov/nchs/data/icd9/icdguide10.pdf. Published 2010. Accessed June 4, 2013.
- Centers for Medicare 27(5):587–612.
- Overview of Disease Severity Measures Disseminated with the Nationwide Inpatient Sample (NIS) and Kids' Inpatient Database (KID). Available at: http://www.hcup‐us.ahrq.gov/db/nation/nis/OverviewofSeveritySystems.pdf. Published December 9, 2005. Accessed June 4, 2013.
- Venous thromboembolism in acutely ill hospitalized medical patients. Thromb Res. 2010;126(4):276–279. , , , et al.
- Venous thromboembolism prophylaxis in hospitalized medical patients and those with stroke: a background review for an American College of Physicians Clinical Practice Guideline. Ann Intern Med. 2011;155(9):602–615. , , , .
- Risk factor model to predict venous thromboembolism in hospitalized medical patients. J Hosp Med. 2011;6(4):202–209. , , , , .
- Evaluation of surveillance bias and the validity of the venous thromboembolism quality measure. JAMA. 2013;310(14):1482–1489. , , , et al.
- Reduction of peripherally inserted central catheter‐associated DVT. Chest. 2013;143(3):627–633. , , , et al.
- Prophylaxis of catheter‐related deep vein thrombosis in cancer patients with low‐dose warfarin, low molecular weight heparin, or control: a randomized, controlled, phase III study. Cancer Chemother Pharmacol. 2013;72(1):65–73. , , , et al.
- American College of Chest Physicians AntithromboticTherapy and Prevention of Thrombosis Panel. Executive summary: Antithrombotic Therapy and Prevention of Thrombosis, 9th ed: American College of Chest Physicians Evidence‐Based Clinical Practice Guidelines [published corrections appear in Chest. 2012;141(4):1129 and 2012;142(6):1698]. Chest. 2012;141(2 suppl):7S–47S. , , , , ;
- Prophylaxis in Medical Patients with Enoxaparin Study Group. A comparison of enoxaparin with placebo for the prevention of venous thromboembolism in acutely ill medical patients. N Engl J Med. 1999;341(11):793–800. , , , et al;
- Randomized, placebo‐controlled trial of dalteparin for the prevention of venous thromboembolism in acutely ill medical patients. Circulation. 2004;110(7):874–879. , , , , , .
- The Joint Commission.Specifications Manual for National Hospital Inpatient Quality Measures. Available at: http://www.jointcommission.org/specifications_manual_for_national_hospital_inpatient_quality_measures.aspx. Accessed July 18, 2012.
- Preventing Hospital‐Acquired Venous Thromboembolism: A Guide for Effective Quality Improvement. Prepared by the Society of Hospital Medicine. Rockville, MD: Agency for Healthcare Research and Quality; AHRQ Publication No. 08‐0075. , .
- Prevention of venous thromboembolism: the Seventh ACCP Conference on Antithrombotic and Thrombolytic Therapy. Chest. 2004;126(3 suppl):338S–400S. , , , et al.
- Prevention of VTE in nonsurgical patients: Antithrombotic Therapy and Prevention of Thrombosis, 9th ed: American College of Chest Physicians Evidence‐Based Clinical Practice Guidelines. Chest. 2012;141(2 suppl):e195S–e226S. , , , et al.
- Centers for Medicare 46(6 part 1):1946–1962.
- Predictive and associative models to identify hospitalized medical patients at risk for VTE. Chest. 2011;140(3):706–714. , , , et al.
- Unintended consequences of a standard admission order set on venous thromboembolism prophylaxis and patient outcomes. J Gen Intern Med. 2012;27(3):318–324. , , , .
- United Health Consortium Website. Available at: https://www.uhc.edu. Accessed March 8, 2012.
- ICD‐9‐CM Official Guidelines for Coding and Reporting. Available at: http://www.cdc.gov/nchs/data/icd9/icdguide10.pdf. Published 2010. Accessed June 4, 2013.
- Centers for Medicare 27(5):587–612.
- Overview of Disease Severity Measures Disseminated with the Nationwide Inpatient Sample (NIS) and Kids' Inpatient Database (KID). Available at: http://www.hcup‐us.ahrq.gov/db/nation/nis/OverviewofSeveritySystems.pdf. Published December 9, 2005. Accessed June 4, 2013.
- Venous thromboembolism in acutely ill hospitalized medical patients. Thromb Res. 2010;126(4):276–279. , , , et al.
- Venous thromboembolism prophylaxis in hospitalized medical patients and those with stroke: a background review for an American College of Physicians Clinical Practice Guideline. Ann Intern Med. 2011;155(9):602–615. , , , .
- Risk factor model to predict venous thromboembolism in hospitalized medical patients. J Hosp Med. 2011;6(4):202–209. , , , , .
- Evaluation of surveillance bias and the validity of the venous thromboembolism quality measure. JAMA. 2013;310(14):1482–1489. , , , et al.
- Reduction of peripherally inserted central catheter‐associated DVT. Chest. 2013;143(3):627–633. , , , et al.
- Prophylaxis of catheter‐related deep vein thrombosis in cancer patients with low‐dose warfarin, low molecular weight heparin, or control: a randomized, controlled, phase III study. Cancer Chemother Pharmacol. 2013;72(1):65–73. , , , et al.
© 2014 Society of Hospital Medicine
Rounding Practices and AGME Competencies
In 1999, the Accreditation Council for Graduate Medical Education (ACGME) established the requirement for residency programs to assess trainees' competencies in 6 core domains: patient care, medical knowledge, practice‐based learning, systems‐based practice, interpersonal and communication skills, and professionalism.[1] With the rollout of the Next Accreditation System (NAS), and a focus of graduate medical education turning to the assessment of milestones within the ACGME core competencies, it is essential for clinician educators to reflect on how current educational activities meet the needs of our learners and enable compliance with the new recommendations.[1]
On internal medicine services in the inpatient setting, clinician educators routinely supervise and teach trainees during attending rounds. The long‐standing practice of rounding innately offers a forum for making patient care decisions and for sharing medical knowledge.[2] However, the rounding process may also afford clinician educators opportunities to teach material relevant to the other 4 ACGME core competencies.[3] Despite the ubiquitous presence of rounds on internal medicine services, rounding practices vary markedly among and within institutions.[4, 5] Furthermore, there is no consensus with respect to best practices for rounds in general, or more specifically as they pertain to graduate medical education and teaching within the 6 core competencies.
We have conducted a multicenter survey study of internal medicine rounding practices at academic institutions from all US regions. As part of a larger investigation of rounding practices, we surveyed attending physicians regarding the frequency with which they participated in different rounding models (card‐flipping rounds [CFR], hallway rounds [HR], or bedside rounds [BR]), and the perceived capacity of each of these models to promote teaching of material relevant to the 6 ACGME core competencies.
METHODS
Sites and Subjects
We disseminated a survey using internal medicine educational leadership and hospital medicine clinical leadership electronic mailing lists (eg, the Society of General Internal Medicine [SGIM] and the Society of Hospital Medicine [SHM]). These listservs gave us access to leaders in the field at institutions affiliated with residency programs. Our initial survey distribution included attending physicians from 58 institutions. We asked these leaders for their assistance in distributing the survey within their respective institutions to physicians who attend on inpatient medicine teaching services.
Survey Development and Domains
The survey was composed of 24 multiple‐choice questions and 1 open‐ended question, and was adapted with permission from a survey created by Mittal and colleagues.[6] We initially piloted the survey with attending physicians in the Division of Hospital Medicine at the University of California, San Francisco. We defined the following 3 models for attending rounds based on our review of the literature, as well as interviews with inpatient clinician educators and internal medicine residency leadership at 3 different institutions: (1) BR, where the discussion of the patient and care plan occur in the presence of the patient with his or her active participation; (2) HR, where the discussion of the patient and care plan occurs partially outside the patient's room and partially at the patient's bedside in the presence of the patient; and (3) CFR, where the discussion of the patient and care plan occurs entirely outside of the patient's room and the team does not see the patient together. The survey asked respondents for their perceptions about how well each model promotes teaching content relevant to the 6 ACGME core competencies (options: very poorly, poorly, neutral, well, very well).
Survey Process
The survey was administered electronically using SurveyMonkey (SurveyMonkey, Menlo Park, CA). We sent an initial survey request to 58 institutional contacts. These contacts were designated clinical and educational leaders in the SHM and SGIM, and were an invited working group. Those leaders were asked to reach out to physicians within their institutions who attended on teaching services. We left the survey open for accrual for a total period of 80 days. Participants received 2 reminder emails asking for their assistance in distributing the survey. The study received approval by our institutional review board.
Data Analysis
We employed means and standard deviations to classify rounding model preference and prevalence. We used Pearson's [2] test to assess the association among the 3 rounding models and the perceptions of how well they worked for teaching material relevant to the ACGME competencies. We dichotomized measures with well and very well, forming the well category. All analysis was conducted using Stata 11.0 (StateCorp, College Station, TX).
RESULTS
Attending Characteristics
We received 153 completed surveys from attending physicians representing 34 unique institutions. All respondents were internal medicine physicians who attend on inpatient medicine teaching services. Institutions spanned all regions of the United States. The characteristics of the surveyed population are described in Table 1.
Variable | Category | Percent |
---|---|---|
| ||
Age, y | 40 | 62% |
4150 | 21% | |
5160 | 13% | |
>60 | 3% | |
Sex | Female | 46% |
Male | 54% | |
Job description | Hospitalist | 61% |
Outpatient internist | 10% | |
Mixed internist* | 14% | |
Specialist | 15% | |
Experience, y | 2 | 21% |
3+ | 79% | |
Months teaching/year | 3 | 50% |
3+ | 50% | |
Decisions requiring attending input | 30% | 40% |
>30% | 60% | |
Team cap | <20 | 47% |
20 | 53% | |
Average daily census | 10 | 51% |
>10 | 49% | |
Region | Midwest | 8% |
Northeast | 19% | |
South | 28% | |
West | 44% | |
Hospital type | University | 82% |
Community | 8% | |
Hospital size, beds | <300 | 23% |
300500 | 32% | |
>500 | 55% |
Rounding Characteristics
HR proved to be the model employed most frequently for both new and established patients (61% and 43%, respectively) (Table 2). The next most frequently utilized rounding models were CFR for established patients (36%) and BR for new patients (22%). Of attending physicians, 53% never used BR for established patients, and 46% never used them at all. When asked about barriers to bedside rounding, respondents cited time constraints, patient psychosocial complexities, and patient privacy as the most significant barriers to performing BR (64%, 39%, and 38%, respectively). Only 6% felt that patient preference was a barrier to bedside rounding.
New Patients | Old Patients | |
---|---|---|
Card‐flipping rounds | 17% (12%22%) | 36% (31%42%) |
Hallway rounds | 61% (55%68%) | 43% (37%48%) |
Bedside rounds | 22% (16%27%) | 21% (15%26%) |
Rounding Models and Core Competencies
Most attending physicians surveyed perceived CFR to perform well or very well for teaching medical knowledge (78%), practice‐based learning (59%), and systems‐based practice (53%). Conversely, a minority thought CFR performed well or very well with respect to teaching patient care (21%), professionalism (27%), or interpersonal skills and communication (16%).
The majority of respondents perceived HR to perform well or very well across all ACGME domains, including teaching patient care (91%), medical knowledge (87%), practice‐based learning (74%), systems‐based practice (69%), professionalism (87%), and interpersonal skills and communication (90%).
Most attending physicians surveyed felt BR performed well or very well with respect to teaching patient care (88%), medical knowledge (58%), professionalism (92%), and interpersonal skills and communication (95%). A minority of participants perceived BR to perform well or very well in teaching practice‐based learning (47%) or systems‐based practice (47%).
Compared with CFR, both HR and BR were perceived to be significantly more effective in teaching patient care, professionalism, and interpersonal skills and communication (Figure 1). Respondents rated BR as significantly inferior to both CFR and HR in teaching medical knowledge. In addition, BR was perceived to be inferior to HR with respect to teaching systems‐based practice and practice‐based learning.
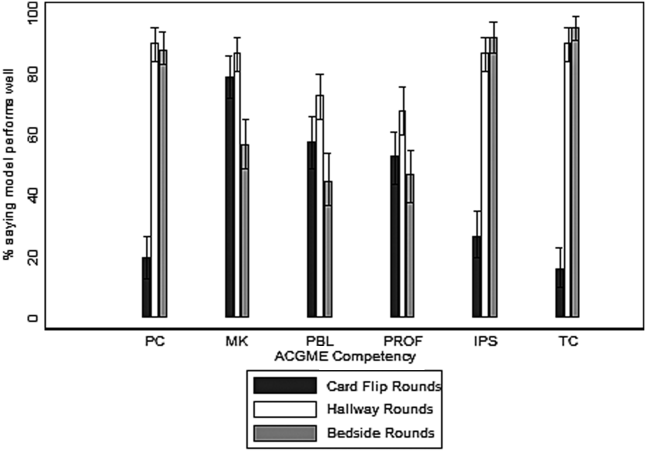
DISCUSSION
In the inpatient setting, attending rounds may offer a primary means for attending physician teaching of trainees. Although all trainees are assessed by their knowledge and skills within the 6 ACGME core competencies, little attention has been paid as to how various rounding models support resident education across these domains.[4] To our knowledge, this is the first cross‐national, multicenter survey study that examines how well the 3 most commonly employed internal medicine rounding practices promote teaching of material relevant to the 6 ACGME core competencies. We found that significant heterogeneity exists in current rounding practices, and different models are perceived to perform variably in their promotion of teaching content within the educational competencies.
In general, with respect to teaching across ACGME domains, CFR were perceived to be less effective compared with HR or BR, and significantly less so in the teaching of patient care, professionalism, and interpersonal skills and communication. Yet, CFR remain widely employed and are used by 17% of attending physicians for new patients and more than 36% for old patients. The reason for their ongoing use was not assessed by our survey; however, this practice does not appear to be driven by educational objectives. The prevalence of CFR may be related to a perception of improved efficiency and a frequent preference among trainees and attending physicians to do this model of rounding. There may be other perceived benefits, including physical comfort of providers or access to the electronic health record, but these qualities were not captured in this study. To our knowledge, there are no prior studies specifically examining CFR as a rounding model.
HR was the most commonly utilized rounding method for the majority of respondents. Attending physicians considered HR particularly effective in teaching patient care, medical knowledge, professionalism, and interpersonal skills and communication. The perceived value of HR may be related to the bimodal nature of the encounter. The discussion of the patient and the care plan outside of the room may include, but is not limited to, formulating the care plan through the formal oral case presentation, focusing on the patient management component of patient care, and sharing medical knowledge without fear of provoking patient anxiety or causing confusion. Subsequently, the time spent in the room may allow for observation and instruction of the physical examination, observation and modeling of professionalism in the patient interaction, and observation and modeling of effective communication with the patient and family members.
We found it interesting that attending physicians also considered HR superior to BR in their capacity to teach practice‐based learning and systems‐based practice. This requires further exploration, as it would seem that increased patient involvement in care plans could offer advantages for the teaching of both of these competencies. Despite the popularity of this rounding structure, there is little prior evidence examining the pros and cons of HR.
Conversely, there is significant literature exploring BR as a rounding model. Prior research has elucidated the multiple benefits of bedside rounding including, but not limited to, teaching history taking, physical examination skills, and clinical ethics; modeling humanism and professionalism; and promoting effective communication.[3, 7, 8, 9, 10, 11] Furthermore, the majority of patients may prefer bedside presentations.[11, 12, 13] Despite their apparent merits, roughly half of attendings surveyed never conduct BR. This may reflect the trend reported in the literature of diminishing bedside teaching, and more specifically, reports that in the United States, less than 5% of time is spent on observing learners' clinical skills and correcting faulty exam techniques.[2, 14] The perception that HR and CFR were superior to BR in teaching medical knowledge suggests that attending physicians value the teaching that occurs away from the patient's bedside. Prior studies suggest that, of the core clinical skills taught on the wards, trainees may find teaching of differential diagnosis to be most challenged by BR, and residents may not appreciate the educational benefits of BR in general.[11, 13] Although time constraints were cited as a significant barrier to BR, recent studies have suggested that BR do not necessarily take more time overall.[15] The notion that patient psychosocial complexities may limit BR has been reflected in the literature,[16, 17, 18, 19, 20] but these situations may also afford unique bedside teaching opportunities.[21] Finally, faculty, and in particular more junior attendings, may be uncomfortable teaching in the presence of the patient.[13] This barrier may be overcome through faculty development efforts.[15]
As internal medicine training transitions to the NAS and a milestone‐based assessment framework,[1] residency programs will need to consider how rounding can be structured to help trainees achieve the required milestones, and to help programs meaningfully assess trainee performance. Our survey indicates that HR may be effective across all of the competencies and the potential for this should be further explored. Yet, HR may allow for a limited ability to observe learners with patients, as there may only be cursory data gathering from the patient, a brief physical exam, and limited communication with patients and/or family members. Furthermore, the patient‐centeredness of HR may be called into question, given restricted emphasis on shared decision making. Finally, as efficiency remains crucial in the wake of duty‐hour reform, HR may also prove to be more time consuming than BR, given that it often requires information shared outside of the patient's room to be repeated in the patient's presence. Ultimately, there may not be a 1 size fits all solution, and institutions should ensure the organization and structure of their rounding models are optimally designed to enable the achievement and assessment of ACGME milestones.
Our study has several limitations. Due to our employment of snowball sampling, we could not calculate a response rate. We also recruited a self‐selected sample of internal medicine attending physicians, raising the possibility of selection bias. However, we captured a wide range of experience and opinion, and do not have reason to believe that any particular viewpoints are over‐ or under‐represented. Further, our study may have been influenced by sampling bias, reaching primarily attending physicians at university‐affiliated medical centers, calling into question the generalizability of our results and making any comparisons between academic and community health centers less meaningful. Nonetheless, we received responses from both large and small medical centers, as well as quaternary care and community‐based hospitals. The reported benefits and barriers were respondents' personal perceptions, rather than measured outcomes. Moreover, we focused primarily on the effectiveness of teaching of the ACGME competencies and did not explore other outcomes that could be impacted by rounding structure (eg, patient satisfaction, trainee satisfaction, length of stay, time of discharge). Our study also did not address the variety of complex factors that influence the location and methods of attending rounds. For example, the various institutions surveyed have a variety of team sizes and compositions, admitting schedules, geographic layouts, and time allotted for attending rounds, all of which can influence choices for rounding practices. Finally, we did not assess resident perceptions, an area of future study that would allow us to corroborate the findings of our survey.
In conclusion, in this cross‐national, multicenter survey study of the 3 most prevalent internal medicine rounding practices, respondents utilized HR most commonly and believed this model was effective in teaching across the 6 ACGME core competencies. Those surveyed identified the benefits and barriers to BR, and a substantial number continue to use CFR despite recognizing its educational limitations. Future studies should explore factors that promote various rounding models and assess the relationship between rounding structure and educational outcomes for trainees.
Disclosure: Nothing to report.
- The next GME accreditation system—rationale and benefits. N Engl J Med. 2012;366(11):1051–1056. , , , .
- Teaching the resident in internal medicine. Present practices and suggestions for the future. JAMA. 1986;256(6):725–729. , .
- Attending rounds and bedside case presentations: medical student and medicine resident experiences and attitudes. Teach Learn Med. 2009;21(2):105–110. , , , .
- Attending rounds in the current era: what is and is not happening. JAMA Intern Med. 2013;173(12):1084–1089. , , , et al.
- Relationships of the location and content of rounds to specialty, institution, patient‐census, and team size. PLoS One. 2010;5(6):e11246. , , , .
- Family‐centered rounds on pediatric wards: a PRIS network survey of US and Canadian hospitalists. Pediatrics. 2010;126(1):37–43. , , , et al.
- Educational strategies to promote clinical diagnostic reasoning. N Engl J Med. 2006;355(21):2217–2225. .
- Role modeling humanistic behavior: learning bedside manner from the experts. Acad Med. 2006;81(7):661–667. , , , , .
- Teaching approaches that reflect and promote professionalism. Acad Med. 2003;78(7):709–713. .
- Bedside rounds revisited. N Engl J Med. 1997;336(16):1174–1175. .
- Sounding boards. The case of bedside rounds. N Engl J Med. 1980;303(21):1230–1233. , .
- The effect of bedside case presentations on patients' perceptions of their medical care. N Engl J Med. 1997;336(16):1150–1155. , , , , .
- Student and patient perspectives on bedside teaching. Med Educ. 1997;31(5):341–346. , , .
- An observational study of attending rounds. J Gen Intern Med. 1992;7(6):646–648. , , , , .
- The return of bedside rounds: an educational intervention. J Gen Intern Med. 2010;25(8):792–798. , , , .
- The attending physician as teacher. N Engl J Med. 1983;308(19):1129–1132. , , .
- Bedside case presentations: why patients like them but learners don't. J Gen Intern Med. 1989;4(4):284–287. , , , , .
- On bedside teaching. Ann Intern Med. 1997;126(3):217–220. .
- Teaching at the bedside: a new model. Med Teach. 2003;25(2):127–130. , .
- Twelve tips to improve bedside teaching. Med Teach. 2003;25(2):112–115. .
- Wiese J, ed. Teaching in the Hospital. Philadelphia, PA: American College of Physicians; 2010.
In 1999, the Accreditation Council for Graduate Medical Education (ACGME) established the requirement for residency programs to assess trainees' competencies in 6 core domains: patient care, medical knowledge, practice‐based learning, systems‐based practice, interpersonal and communication skills, and professionalism.[1] With the rollout of the Next Accreditation System (NAS), and a focus of graduate medical education turning to the assessment of milestones within the ACGME core competencies, it is essential for clinician educators to reflect on how current educational activities meet the needs of our learners and enable compliance with the new recommendations.[1]
On internal medicine services in the inpatient setting, clinician educators routinely supervise and teach trainees during attending rounds. The long‐standing practice of rounding innately offers a forum for making patient care decisions and for sharing medical knowledge.[2] However, the rounding process may also afford clinician educators opportunities to teach material relevant to the other 4 ACGME core competencies.[3] Despite the ubiquitous presence of rounds on internal medicine services, rounding practices vary markedly among and within institutions.[4, 5] Furthermore, there is no consensus with respect to best practices for rounds in general, or more specifically as they pertain to graduate medical education and teaching within the 6 core competencies.
We have conducted a multicenter survey study of internal medicine rounding practices at academic institutions from all US regions. As part of a larger investigation of rounding practices, we surveyed attending physicians regarding the frequency with which they participated in different rounding models (card‐flipping rounds [CFR], hallway rounds [HR], or bedside rounds [BR]), and the perceived capacity of each of these models to promote teaching of material relevant to the 6 ACGME core competencies.
METHODS
Sites and Subjects
We disseminated a survey using internal medicine educational leadership and hospital medicine clinical leadership electronic mailing lists (eg, the Society of General Internal Medicine [SGIM] and the Society of Hospital Medicine [SHM]). These listservs gave us access to leaders in the field at institutions affiliated with residency programs. Our initial survey distribution included attending physicians from 58 institutions. We asked these leaders for their assistance in distributing the survey within their respective institutions to physicians who attend on inpatient medicine teaching services.
Survey Development and Domains
The survey was composed of 24 multiple‐choice questions and 1 open‐ended question, and was adapted with permission from a survey created by Mittal and colleagues.[6] We initially piloted the survey with attending physicians in the Division of Hospital Medicine at the University of California, San Francisco. We defined the following 3 models for attending rounds based on our review of the literature, as well as interviews with inpatient clinician educators and internal medicine residency leadership at 3 different institutions: (1) BR, where the discussion of the patient and care plan occur in the presence of the patient with his or her active participation; (2) HR, where the discussion of the patient and care plan occurs partially outside the patient's room and partially at the patient's bedside in the presence of the patient; and (3) CFR, where the discussion of the patient and care plan occurs entirely outside of the patient's room and the team does not see the patient together. The survey asked respondents for their perceptions about how well each model promotes teaching content relevant to the 6 ACGME core competencies (options: very poorly, poorly, neutral, well, very well).
Survey Process
The survey was administered electronically using SurveyMonkey (SurveyMonkey, Menlo Park, CA). We sent an initial survey request to 58 institutional contacts. These contacts were designated clinical and educational leaders in the SHM and SGIM, and were an invited working group. Those leaders were asked to reach out to physicians within their institutions who attended on teaching services. We left the survey open for accrual for a total period of 80 days. Participants received 2 reminder emails asking for their assistance in distributing the survey. The study received approval by our institutional review board.
Data Analysis
We employed means and standard deviations to classify rounding model preference and prevalence. We used Pearson's [2] test to assess the association among the 3 rounding models and the perceptions of how well they worked for teaching material relevant to the ACGME competencies. We dichotomized measures with well and very well, forming the well category. All analysis was conducted using Stata 11.0 (StateCorp, College Station, TX).
RESULTS
Attending Characteristics
We received 153 completed surveys from attending physicians representing 34 unique institutions. All respondents were internal medicine physicians who attend on inpatient medicine teaching services. Institutions spanned all regions of the United States. The characteristics of the surveyed population are described in Table 1.
Variable | Category | Percent |
---|---|---|
| ||
Age, y | 40 | 62% |
4150 | 21% | |
5160 | 13% | |
>60 | 3% | |
Sex | Female | 46% |
Male | 54% | |
Job description | Hospitalist | 61% |
Outpatient internist | 10% | |
Mixed internist* | 14% | |
Specialist | 15% | |
Experience, y | 2 | 21% |
3+ | 79% | |
Months teaching/year | 3 | 50% |
3+ | 50% | |
Decisions requiring attending input | 30% | 40% |
>30% | 60% | |
Team cap | <20 | 47% |
20 | 53% | |
Average daily census | 10 | 51% |
>10 | 49% | |
Region | Midwest | 8% |
Northeast | 19% | |
South | 28% | |
West | 44% | |
Hospital type | University | 82% |
Community | 8% | |
Hospital size, beds | <300 | 23% |
300500 | 32% | |
>500 | 55% |
Rounding Characteristics
HR proved to be the model employed most frequently for both new and established patients (61% and 43%, respectively) (Table 2). The next most frequently utilized rounding models were CFR for established patients (36%) and BR for new patients (22%). Of attending physicians, 53% never used BR for established patients, and 46% never used them at all. When asked about barriers to bedside rounding, respondents cited time constraints, patient psychosocial complexities, and patient privacy as the most significant barriers to performing BR (64%, 39%, and 38%, respectively). Only 6% felt that patient preference was a barrier to bedside rounding.
New Patients | Old Patients | |
---|---|---|
Card‐flipping rounds | 17% (12%22%) | 36% (31%42%) |
Hallway rounds | 61% (55%68%) | 43% (37%48%) |
Bedside rounds | 22% (16%27%) | 21% (15%26%) |
Rounding Models and Core Competencies
Most attending physicians surveyed perceived CFR to perform well or very well for teaching medical knowledge (78%), practice‐based learning (59%), and systems‐based practice (53%). Conversely, a minority thought CFR performed well or very well with respect to teaching patient care (21%), professionalism (27%), or interpersonal skills and communication (16%).
The majority of respondents perceived HR to perform well or very well across all ACGME domains, including teaching patient care (91%), medical knowledge (87%), practice‐based learning (74%), systems‐based practice (69%), professionalism (87%), and interpersonal skills and communication (90%).
Most attending physicians surveyed felt BR performed well or very well with respect to teaching patient care (88%), medical knowledge (58%), professionalism (92%), and interpersonal skills and communication (95%). A minority of participants perceived BR to perform well or very well in teaching practice‐based learning (47%) or systems‐based practice (47%).
Compared with CFR, both HR and BR were perceived to be significantly more effective in teaching patient care, professionalism, and interpersonal skills and communication (Figure 1). Respondents rated BR as significantly inferior to both CFR and HR in teaching medical knowledge. In addition, BR was perceived to be inferior to HR with respect to teaching systems‐based practice and practice‐based learning.
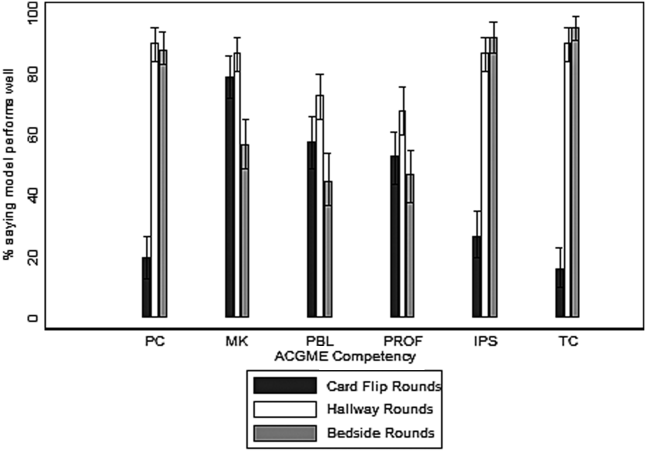
DISCUSSION
In the inpatient setting, attending rounds may offer a primary means for attending physician teaching of trainees. Although all trainees are assessed by their knowledge and skills within the 6 ACGME core competencies, little attention has been paid as to how various rounding models support resident education across these domains.[4] To our knowledge, this is the first cross‐national, multicenter survey study that examines how well the 3 most commonly employed internal medicine rounding practices promote teaching of material relevant to the 6 ACGME core competencies. We found that significant heterogeneity exists in current rounding practices, and different models are perceived to perform variably in their promotion of teaching content within the educational competencies.
In general, with respect to teaching across ACGME domains, CFR were perceived to be less effective compared with HR or BR, and significantly less so in the teaching of patient care, professionalism, and interpersonal skills and communication. Yet, CFR remain widely employed and are used by 17% of attending physicians for new patients and more than 36% for old patients. The reason for their ongoing use was not assessed by our survey; however, this practice does not appear to be driven by educational objectives. The prevalence of CFR may be related to a perception of improved efficiency and a frequent preference among trainees and attending physicians to do this model of rounding. There may be other perceived benefits, including physical comfort of providers or access to the electronic health record, but these qualities were not captured in this study. To our knowledge, there are no prior studies specifically examining CFR as a rounding model.
HR was the most commonly utilized rounding method for the majority of respondents. Attending physicians considered HR particularly effective in teaching patient care, medical knowledge, professionalism, and interpersonal skills and communication. The perceived value of HR may be related to the bimodal nature of the encounter. The discussion of the patient and the care plan outside of the room may include, but is not limited to, formulating the care plan through the formal oral case presentation, focusing on the patient management component of patient care, and sharing medical knowledge without fear of provoking patient anxiety or causing confusion. Subsequently, the time spent in the room may allow for observation and instruction of the physical examination, observation and modeling of professionalism in the patient interaction, and observation and modeling of effective communication with the patient and family members.
We found it interesting that attending physicians also considered HR superior to BR in their capacity to teach practice‐based learning and systems‐based practice. This requires further exploration, as it would seem that increased patient involvement in care plans could offer advantages for the teaching of both of these competencies. Despite the popularity of this rounding structure, there is little prior evidence examining the pros and cons of HR.
Conversely, there is significant literature exploring BR as a rounding model. Prior research has elucidated the multiple benefits of bedside rounding including, but not limited to, teaching history taking, physical examination skills, and clinical ethics; modeling humanism and professionalism; and promoting effective communication.[3, 7, 8, 9, 10, 11] Furthermore, the majority of patients may prefer bedside presentations.[11, 12, 13] Despite their apparent merits, roughly half of attendings surveyed never conduct BR. This may reflect the trend reported in the literature of diminishing bedside teaching, and more specifically, reports that in the United States, less than 5% of time is spent on observing learners' clinical skills and correcting faulty exam techniques.[2, 14] The perception that HR and CFR were superior to BR in teaching medical knowledge suggests that attending physicians value the teaching that occurs away from the patient's bedside. Prior studies suggest that, of the core clinical skills taught on the wards, trainees may find teaching of differential diagnosis to be most challenged by BR, and residents may not appreciate the educational benefits of BR in general.[11, 13] Although time constraints were cited as a significant barrier to BR, recent studies have suggested that BR do not necessarily take more time overall.[15] The notion that patient psychosocial complexities may limit BR has been reflected in the literature,[16, 17, 18, 19, 20] but these situations may also afford unique bedside teaching opportunities.[21] Finally, faculty, and in particular more junior attendings, may be uncomfortable teaching in the presence of the patient.[13] This barrier may be overcome through faculty development efforts.[15]
As internal medicine training transitions to the NAS and a milestone‐based assessment framework,[1] residency programs will need to consider how rounding can be structured to help trainees achieve the required milestones, and to help programs meaningfully assess trainee performance. Our survey indicates that HR may be effective across all of the competencies and the potential for this should be further explored. Yet, HR may allow for a limited ability to observe learners with patients, as there may only be cursory data gathering from the patient, a brief physical exam, and limited communication with patients and/or family members. Furthermore, the patient‐centeredness of HR may be called into question, given restricted emphasis on shared decision making. Finally, as efficiency remains crucial in the wake of duty‐hour reform, HR may also prove to be more time consuming than BR, given that it often requires information shared outside of the patient's room to be repeated in the patient's presence. Ultimately, there may not be a 1 size fits all solution, and institutions should ensure the organization and structure of their rounding models are optimally designed to enable the achievement and assessment of ACGME milestones.
Our study has several limitations. Due to our employment of snowball sampling, we could not calculate a response rate. We also recruited a self‐selected sample of internal medicine attending physicians, raising the possibility of selection bias. However, we captured a wide range of experience and opinion, and do not have reason to believe that any particular viewpoints are over‐ or under‐represented. Further, our study may have been influenced by sampling bias, reaching primarily attending physicians at university‐affiliated medical centers, calling into question the generalizability of our results and making any comparisons between academic and community health centers less meaningful. Nonetheless, we received responses from both large and small medical centers, as well as quaternary care and community‐based hospitals. The reported benefits and barriers were respondents' personal perceptions, rather than measured outcomes. Moreover, we focused primarily on the effectiveness of teaching of the ACGME competencies and did not explore other outcomes that could be impacted by rounding structure (eg, patient satisfaction, trainee satisfaction, length of stay, time of discharge). Our study also did not address the variety of complex factors that influence the location and methods of attending rounds. For example, the various institutions surveyed have a variety of team sizes and compositions, admitting schedules, geographic layouts, and time allotted for attending rounds, all of which can influence choices for rounding practices. Finally, we did not assess resident perceptions, an area of future study that would allow us to corroborate the findings of our survey.
In conclusion, in this cross‐national, multicenter survey study of the 3 most prevalent internal medicine rounding practices, respondents utilized HR most commonly and believed this model was effective in teaching across the 6 ACGME core competencies. Those surveyed identified the benefits and barriers to BR, and a substantial number continue to use CFR despite recognizing its educational limitations. Future studies should explore factors that promote various rounding models and assess the relationship between rounding structure and educational outcomes for trainees.
Disclosure: Nothing to report.
In 1999, the Accreditation Council for Graduate Medical Education (ACGME) established the requirement for residency programs to assess trainees' competencies in 6 core domains: patient care, medical knowledge, practice‐based learning, systems‐based practice, interpersonal and communication skills, and professionalism.[1] With the rollout of the Next Accreditation System (NAS), and a focus of graduate medical education turning to the assessment of milestones within the ACGME core competencies, it is essential for clinician educators to reflect on how current educational activities meet the needs of our learners and enable compliance with the new recommendations.[1]
On internal medicine services in the inpatient setting, clinician educators routinely supervise and teach trainees during attending rounds. The long‐standing practice of rounding innately offers a forum for making patient care decisions and for sharing medical knowledge.[2] However, the rounding process may also afford clinician educators opportunities to teach material relevant to the other 4 ACGME core competencies.[3] Despite the ubiquitous presence of rounds on internal medicine services, rounding practices vary markedly among and within institutions.[4, 5] Furthermore, there is no consensus with respect to best practices for rounds in general, or more specifically as they pertain to graduate medical education and teaching within the 6 core competencies.
We have conducted a multicenter survey study of internal medicine rounding practices at academic institutions from all US regions. As part of a larger investigation of rounding practices, we surveyed attending physicians regarding the frequency with which they participated in different rounding models (card‐flipping rounds [CFR], hallway rounds [HR], or bedside rounds [BR]), and the perceived capacity of each of these models to promote teaching of material relevant to the 6 ACGME core competencies.
METHODS
Sites and Subjects
We disseminated a survey using internal medicine educational leadership and hospital medicine clinical leadership electronic mailing lists (eg, the Society of General Internal Medicine [SGIM] and the Society of Hospital Medicine [SHM]). These listservs gave us access to leaders in the field at institutions affiliated with residency programs. Our initial survey distribution included attending physicians from 58 institutions. We asked these leaders for their assistance in distributing the survey within their respective institutions to physicians who attend on inpatient medicine teaching services.
Survey Development and Domains
The survey was composed of 24 multiple‐choice questions and 1 open‐ended question, and was adapted with permission from a survey created by Mittal and colleagues.[6] We initially piloted the survey with attending physicians in the Division of Hospital Medicine at the University of California, San Francisco. We defined the following 3 models for attending rounds based on our review of the literature, as well as interviews with inpatient clinician educators and internal medicine residency leadership at 3 different institutions: (1) BR, where the discussion of the patient and care plan occur in the presence of the patient with his or her active participation; (2) HR, where the discussion of the patient and care plan occurs partially outside the patient's room and partially at the patient's bedside in the presence of the patient; and (3) CFR, where the discussion of the patient and care plan occurs entirely outside of the patient's room and the team does not see the patient together. The survey asked respondents for their perceptions about how well each model promotes teaching content relevant to the 6 ACGME core competencies (options: very poorly, poorly, neutral, well, very well).
Survey Process
The survey was administered electronically using SurveyMonkey (SurveyMonkey, Menlo Park, CA). We sent an initial survey request to 58 institutional contacts. These contacts were designated clinical and educational leaders in the SHM and SGIM, and were an invited working group. Those leaders were asked to reach out to physicians within their institutions who attended on teaching services. We left the survey open for accrual for a total period of 80 days. Participants received 2 reminder emails asking for their assistance in distributing the survey. The study received approval by our institutional review board.
Data Analysis
We employed means and standard deviations to classify rounding model preference and prevalence. We used Pearson's [2] test to assess the association among the 3 rounding models and the perceptions of how well they worked for teaching material relevant to the ACGME competencies. We dichotomized measures with well and very well, forming the well category. All analysis was conducted using Stata 11.0 (StateCorp, College Station, TX).
RESULTS
Attending Characteristics
We received 153 completed surveys from attending physicians representing 34 unique institutions. All respondents were internal medicine physicians who attend on inpatient medicine teaching services. Institutions spanned all regions of the United States. The characteristics of the surveyed population are described in Table 1.
Variable | Category | Percent |
---|---|---|
| ||
Age, y | 40 | 62% |
4150 | 21% | |
5160 | 13% | |
>60 | 3% | |
Sex | Female | 46% |
Male | 54% | |
Job description | Hospitalist | 61% |
Outpatient internist | 10% | |
Mixed internist* | 14% | |
Specialist | 15% | |
Experience, y | 2 | 21% |
3+ | 79% | |
Months teaching/year | 3 | 50% |
3+ | 50% | |
Decisions requiring attending input | 30% | 40% |
>30% | 60% | |
Team cap | <20 | 47% |
20 | 53% | |
Average daily census | 10 | 51% |
>10 | 49% | |
Region | Midwest | 8% |
Northeast | 19% | |
South | 28% | |
West | 44% | |
Hospital type | University | 82% |
Community | 8% | |
Hospital size, beds | <300 | 23% |
300500 | 32% | |
>500 | 55% |
Rounding Characteristics
HR proved to be the model employed most frequently for both new and established patients (61% and 43%, respectively) (Table 2). The next most frequently utilized rounding models were CFR for established patients (36%) and BR for new patients (22%). Of attending physicians, 53% never used BR for established patients, and 46% never used them at all. When asked about barriers to bedside rounding, respondents cited time constraints, patient psychosocial complexities, and patient privacy as the most significant barriers to performing BR (64%, 39%, and 38%, respectively). Only 6% felt that patient preference was a barrier to bedside rounding.
New Patients | Old Patients | |
---|---|---|
Card‐flipping rounds | 17% (12%22%) | 36% (31%42%) |
Hallway rounds | 61% (55%68%) | 43% (37%48%) |
Bedside rounds | 22% (16%27%) | 21% (15%26%) |
Rounding Models and Core Competencies
Most attending physicians surveyed perceived CFR to perform well or very well for teaching medical knowledge (78%), practice‐based learning (59%), and systems‐based practice (53%). Conversely, a minority thought CFR performed well or very well with respect to teaching patient care (21%), professionalism (27%), or interpersonal skills and communication (16%).
The majority of respondents perceived HR to perform well or very well across all ACGME domains, including teaching patient care (91%), medical knowledge (87%), practice‐based learning (74%), systems‐based practice (69%), professionalism (87%), and interpersonal skills and communication (90%).
Most attending physicians surveyed felt BR performed well or very well with respect to teaching patient care (88%), medical knowledge (58%), professionalism (92%), and interpersonal skills and communication (95%). A minority of participants perceived BR to perform well or very well in teaching practice‐based learning (47%) or systems‐based practice (47%).
Compared with CFR, both HR and BR were perceived to be significantly more effective in teaching patient care, professionalism, and interpersonal skills and communication (Figure 1). Respondents rated BR as significantly inferior to both CFR and HR in teaching medical knowledge. In addition, BR was perceived to be inferior to HR with respect to teaching systems‐based practice and practice‐based learning.
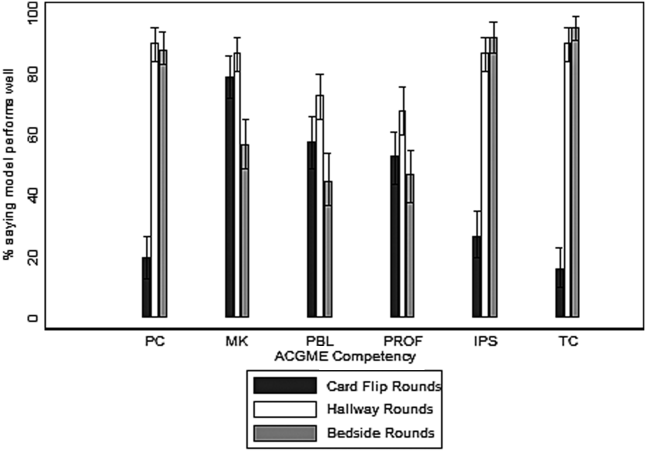
DISCUSSION
In the inpatient setting, attending rounds may offer a primary means for attending physician teaching of trainees. Although all trainees are assessed by their knowledge and skills within the 6 ACGME core competencies, little attention has been paid as to how various rounding models support resident education across these domains.[4] To our knowledge, this is the first cross‐national, multicenter survey study that examines how well the 3 most commonly employed internal medicine rounding practices promote teaching of material relevant to the 6 ACGME core competencies. We found that significant heterogeneity exists in current rounding practices, and different models are perceived to perform variably in their promotion of teaching content within the educational competencies.
In general, with respect to teaching across ACGME domains, CFR were perceived to be less effective compared with HR or BR, and significantly less so in the teaching of patient care, professionalism, and interpersonal skills and communication. Yet, CFR remain widely employed and are used by 17% of attending physicians for new patients and more than 36% for old patients. The reason for their ongoing use was not assessed by our survey; however, this practice does not appear to be driven by educational objectives. The prevalence of CFR may be related to a perception of improved efficiency and a frequent preference among trainees and attending physicians to do this model of rounding. There may be other perceived benefits, including physical comfort of providers or access to the electronic health record, but these qualities were not captured in this study. To our knowledge, there are no prior studies specifically examining CFR as a rounding model.
HR was the most commonly utilized rounding method for the majority of respondents. Attending physicians considered HR particularly effective in teaching patient care, medical knowledge, professionalism, and interpersonal skills and communication. The perceived value of HR may be related to the bimodal nature of the encounter. The discussion of the patient and the care plan outside of the room may include, but is not limited to, formulating the care plan through the formal oral case presentation, focusing on the patient management component of patient care, and sharing medical knowledge without fear of provoking patient anxiety or causing confusion. Subsequently, the time spent in the room may allow for observation and instruction of the physical examination, observation and modeling of professionalism in the patient interaction, and observation and modeling of effective communication with the patient and family members.
We found it interesting that attending physicians also considered HR superior to BR in their capacity to teach practice‐based learning and systems‐based practice. This requires further exploration, as it would seem that increased patient involvement in care plans could offer advantages for the teaching of both of these competencies. Despite the popularity of this rounding structure, there is little prior evidence examining the pros and cons of HR.
Conversely, there is significant literature exploring BR as a rounding model. Prior research has elucidated the multiple benefits of bedside rounding including, but not limited to, teaching history taking, physical examination skills, and clinical ethics; modeling humanism and professionalism; and promoting effective communication.[3, 7, 8, 9, 10, 11] Furthermore, the majority of patients may prefer bedside presentations.[11, 12, 13] Despite their apparent merits, roughly half of attendings surveyed never conduct BR. This may reflect the trend reported in the literature of diminishing bedside teaching, and more specifically, reports that in the United States, less than 5% of time is spent on observing learners' clinical skills and correcting faulty exam techniques.[2, 14] The perception that HR and CFR were superior to BR in teaching medical knowledge suggests that attending physicians value the teaching that occurs away from the patient's bedside. Prior studies suggest that, of the core clinical skills taught on the wards, trainees may find teaching of differential diagnosis to be most challenged by BR, and residents may not appreciate the educational benefits of BR in general.[11, 13] Although time constraints were cited as a significant barrier to BR, recent studies have suggested that BR do not necessarily take more time overall.[15] The notion that patient psychosocial complexities may limit BR has been reflected in the literature,[16, 17, 18, 19, 20] but these situations may also afford unique bedside teaching opportunities.[21] Finally, faculty, and in particular more junior attendings, may be uncomfortable teaching in the presence of the patient.[13] This barrier may be overcome through faculty development efforts.[15]
As internal medicine training transitions to the NAS and a milestone‐based assessment framework,[1] residency programs will need to consider how rounding can be structured to help trainees achieve the required milestones, and to help programs meaningfully assess trainee performance. Our survey indicates that HR may be effective across all of the competencies and the potential for this should be further explored. Yet, HR may allow for a limited ability to observe learners with patients, as there may only be cursory data gathering from the patient, a brief physical exam, and limited communication with patients and/or family members. Furthermore, the patient‐centeredness of HR may be called into question, given restricted emphasis on shared decision making. Finally, as efficiency remains crucial in the wake of duty‐hour reform, HR may also prove to be more time consuming than BR, given that it often requires information shared outside of the patient's room to be repeated in the patient's presence. Ultimately, there may not be a 1 size fits all solution, and institutions should ensure the organization and structure of their rounding models are optimally designed to enable the achievement and assessment of ACGME milestones.
Our study has several limitations. Due to our employment of snowball sampling, we could not calculate a response rate. We also recruited a self‐selected sample of internal medicine attending physicians, raising the possibility of selection bias. However, we captured a wide range of experience and opinion, and do not have reason to believe that any particular viewpoints are over‐ or under‐represented. Further, our study may have been influenced by sampling bias, reaching primarily attending physicians at university‐affiliated medical centers, calling into question the generalizability of our results and making any comparisons between academic and community health centers less meaningful. Nonetheless, we received responses from both large and small medical centers, as well as quaternary care and community‐based hospitals. The reported benefits and barriers were respondents' personal perceptions, rather than measured outcomes. Moreover, we focused primarily on the effectiveness of teaching of the ACGME competencies and did not explore other outcomes that could be impacted by rounding structure (eg, patient satisfaction, trainee satisfaction, length of stay, time of discharge). Our study also did not address the variety of complex factors that influence the location and methods of attending rounds. For example, the various institutions surveyed have a variety of team sizes and compositions, admitting schedules, geographic layouts, and time allotted for attending rounds, all of which can influence choices for rounding practices. Finally, we did not assess resident perceptions, an area of future study that would allow us to corroborate the findings of our survey.
In conclusion, in this cross‐national, multicenter survey study of the 3 most prevalent internal medicine rounding practices, respondents utilized HR most commonly and believed this model was effective in teaching across the 6 ACGME core competencies. Those surveyed identified the benefits and barriers to BR, and a substantial number continue to use CFR despite recognizing its educational limitations. Future studies should explore factors that promote various rounding models and assess the relationship between rounding structure and educational outcomes for trainees.
Disclosure: Nothing to report.
- The next GME accreditation system—rationale and benefits. N Engl J Med. 2012;366(11):1051–1056. , , , .
- Teaching the resident in internal medicine. Present practices and suggestions for the future. JAMA. 1986;256(6):725–729. , .
- Attending rounds and bedside case presentations: medical student and medicine resident experiences and attitudes. Teach Learn Med. 2009;21(2):105–110. , , , .
- Attending rounds in the current era: what is and is not happening. JAMA Intern Med. 2013;173(12):1084–1089. , , , et al.
- Relationships of the location and content of rounds to specialty, institution, patient‐census, and team size. PLoS One. 2010;5(6):e11246. , , , .
- Family‐centered rounds on pediatric wards: a PRIS network survey of US and Canadian hospitalists. Pediatrics. 2010;126(1):37–43. , , , et al.
- Educational strategies to promote clinical diagnostic reasoning. N Engl J Med. 2006;355(21):2217–2225. .
- Role modeling humanistic behavior: learning bedside manner from the experts. Acad Med. 2006;81(7):661–667. , , , , .
- Teaching approaches that reflect and promote professionalism. Acad Med. 2003;78(7):709–713. .
- Bedside rounds revisited. N Engl J Med. 1997;336(16):1174–1175. .
- Sounding boards. The case of bedside rounds. N Engl J Med. 1980;303(21):1230–1233. , .
- The effect of bedside case presentations on patients' perceptions of their medical care. N Engl J Med. 1997;336(16):1150–1155. , , , , .
- Student and patient perspectives on bedside teaching. Med Educ. 1997;31(5):341–346. , , .
- An observational study of attending rounds. J Gen Intern Med. 1992;7(6):646–648. , , , , .
- The return of bedside rounds: an educational intervention. J Gen Intern Med. 2010;25(8):792–798. , , , .
- The attending physician as teacher. N Engl J Med. 1983;308(19):1129–1132. , , .
- Bedside case presentations: why patients like them but learners don't. J Gen Intern Med. 1989;4(4):284–287. , , , , .
- On bedside teaching. Ann Intern Med. 1997;126(3):217–220. .
- Teaching at the bedside: a new model. Med Teach. 2003;25(2):127–130. , .
- Twelve tips to improve bedside teaching. Med Teach. 2003;25(2):112–115. .
- Wiese J, ed. Teaching in the Hospital. Philadelphia, PA: American College of Physicians; 2010.
- The next GME accreditation system—rationale and benefits. N Engl J Med. 2012;366(11):1051–1056. , , , .
- Teaching the resident in internal medicine. Present practices and suggestions for the future. JAMA. 1986;256(6):725–729. , .
- Attending rounds and bedside case presentations: medical student and medicine resident experiences and attitudes. Teach Learn Med. 2009;21(2):105–110. , , , .
- Attending rounds in the current era: what is and is not happening. JAMA Intern Med. 2013;173(12):1084–1089. , , , et al.
- Relationships of the location and content of rounds to specialty, institution, patient‐census, and team size. PLoS One. 2010;5(6):e11246. , , , .
- Family‐centered rounds on pediatric wards: a PRIS network survey of US and Canadian hospitalists. Pediatrics. 2010;126(1):37–43. , , , et al.
- Educational strategies to promote clinical diagnostic reasoning. N Engl J Med. 2006;355(21):2217–2225. .
- Role modeling humanistic behavior: learning bedside manner from the experts. Acad Med. 2006;81(7):661–667. , , , , .
- Teaching approaches that reflect and promote professionalism. Acad Med. 2003;78(7):709–713. .
- Bedside rounds revisited. N Engl J Med. 1997;336(16):1174–1175. .
- Sounding boards. The case of bedside rounds. N Engl J Med. 1980;303(21):1230–1233. , .
- The effect of bedside case presentations on patients' perceptions of their medical care. N Engl J Med. 1997;336(16):1150–1155. , , , , .
- Student and patient perspectives on bedside teaching. Med Educ. 1997;31(5):341–346. , , .
- An observational study of attending rounds. J Gen Intern Med. 1992;7(6):646–648. , , , , .
- The return of bedside rounds: an educational intervention. J Gen Intern Med. 2010;25(8):792–798. , , , .
- The attending physician as teacher. N Engl J Med. 1983;308(19):1129–1132. , , .
- Bedside case presentations: why patients like them but learners don't. J Gen Intern Med. 1989;4(4):284–287. , , , , .
- On bedside teaching. Ann Intern Med. 1997;126(3):217–220. .
- Teaching at the bedside: a new model. Med Teach. 2003;25(2):127–130. , .
- Twelve tips to improve bedside teaching. Med Teach. 2003;25(2):112–115. .
- Wiese J, ed. Teaching in the Hospital. Philadelphia, PA: American College of Physicians; 2010.
© 2014 Society of Hospital Medicine
Night or Weekend Admission and Outcomes
The hospitalist movement and increasingly stringent resident work hour restrictions have led to the utilization of shift work in many hospitals.1 Use of nocturnist and night float systems, while often necessary, results in increased patient hand‐offs. Research suggests that hand‐offs in the inpatient setting can adversely affect patient outcomes as lack of continuity may increase the possibility of medical error.2, 3 In 2001, Bell et al.4 found that mortality was higher among patients admitted on weekends as compared to weekdays. Uneven staffing, lack of supervision, and fragmented care were cited as potential contributing factors.4 Similarly, Peberdy et al.5 in 2008 revealed that patients were less likely to survive a cardiac arrest if it occurred at night or on weekends, again attributed in part to fragmented patient care and understaffing.
The results of these studies raise concerns as to whether increased reliance on shift work and resulting handoffs compromises patient care.6, 7 The aim of this study was to evaluate the potential association between night admission and hospitalization‐relevant outcomes (length of stay [LOS], hospital charges, intensive care unit [ICU] transfer during hospitalization, repeat emergency department [ED] visit within 30 days of discharge, readmission within 30 days of discharge, and poor outcome [transfer to the ICU, cardiac arrest, or death] within the first 24 hours of admission) at an institution that exclusively uses nocturnists (night‐shift based hospitalists) and a resident night float system for patients admitted at night to the general medicine service. A secondary aim was to determine the potential association between weekend admission and hospitalization‐relevant outcomes.
Methods
Study Sample and Selection
We conducted a retrospective medical record review at a large urban academic hospital. Using an administrative hospital data set, we assembled a list of approximately 9000 admissions to the general medicine service from the ED between January 2008 and October 2008. We sampled consecutive admissions from 3 distinct periods beginning in January, April, and July to capture outcomes at various points in the academic year. We attempted to review approximately 10% of all charts equally distributed among the 3 sampling periods (ie, 900 charts total with one‐third from each period) based on time available to the reviewers. We excluded patients not admitted to the general medicine service and patients without complete demographic or outcome information. We also excluded patients not admitted from the ED given that the vast majority of admissions to our hospital during the night (96%) or weekend (93%) are from the ED. Patients admitted to the general medicine service are cared for either by a hospitalist or by a teaching team comprised of 1 attending (about 40% of whom are hospitalists), 1 resident, 1 to 2 interns, and 1 to 3 medical students. From 7 am to 6:59 pm patients are admitted to the care of 1 of the primary daytime admitting teams. From 7 pm to 6:59 am patients are admitted by nocturnists (hospitalist service) or night float residents (teaching service). These patients are handed off to day teams at 7 am. Hospitalist teams change service on a weekly to biweekly basis and resident teams switch on a monthly basis; there is no difference in physician staffing between the weekend and weekdays. The Northwestern University Institutional Review Board approved this study.
Data Acquisition and Medical Records Reviews
We obtained demographic data including gender, age, race and ethnicity, patient insurance, admission day (weekday vs. weekend), admission time (defined as the time that a patient receives a hospital bed, which at our institution is also the time that admitting teams receive report and assume care for the patient), and the International Classification of Disease codes required to determine the Major Diagnostic Category (MDC) and calculate the Charlson Comorbidity Index8, 9 as part of an administrative data set. We divided the admission time into night admission (defined as 7 pm to 6:59 am) and day admission (defined as 7:00 am to 6:59 pm). We created a chart abstraction tool to allow manual recording of the additional fields of admitting team (hospitalist vs. resident), 30 day repeat ED visit, 30 day readmission, and poor outcomes within the first 24 hours of admission, directly from the electronic record.
Study Outcomes
We evaluated each admission for the following 6 primary outcomes which were specified a priori: LOS (defined as discharge date and time minus admission date and time), hospital charges (defined as charges billed as recorded in the administrative data set), ICU transfer during hospitalization (defined as 1 ICU day in the administrative data set), 30 day repeat ED visit (defined as a visit to our ED within 30 days of discharge as assessed by chart abstraction), 30 day readmission (defined as any planned or unplanned admission to any inpatient service at our institution within 30 days of discharge as assessed by chart abstraction), and poor outcome within 24 hours of admission (defined as transfer to the ICU, cardiac arrest, or death as assessed by chart abstraction). Each of these outcomes has been used in prior work to assess the quality of inpatient care.10, 11
Statistical Analysis
Interrater reliability between the 3 physician reviewers was assessed for 20 randomly selected admissions across the 4 separate review measures using interclass correlation coefficients. Comparisons between night admissions and day admissions, and between weekend and weekday admissions, for the continuous primary outcomes (LOS, hospital charges) were assessed using 2‐tailed t‐tests as well as Wilcoxon rank sum test. In the multivariable modeling, these outcomes were assessed by linear regression controlling for age, gender, race and ethnicity, Medicaid or self‐pay insurance, admission to the hospitalist or teaching service, most common MDC categories, and Charlson Comorbidity Index. Because both outcomes were right‐skewed, we separately assessed each after log‐transformation controlling for the same variables.
All comparisons of the dichotomous primary outcomes (ICU transfer during hospitalization, 30 day repeat ED visit, 30 day readmission, and poor outcome within the first 24 hours after admission) were assessed at the univariate level by chi‐squared test, and in the multivariable models using logistic regression, controlling for the same variables as the linear models above. All adjustments were specified a priori. All data analyses were conducted using Stata (College Station, TX; Version 11).
Results
We reviewed 857 records. After excluding 33 records lacking administrative data regarding gender, race and ethnicity, and other demographic variables, there were 824 medical records available for analysis. We reviewed a similar number of records from each time period: 274 from January 2008, 265 from April 2008, and 285 from July 2008. A total of 345 (42%) patients were admitted during the day, and 479 (58%) at night; 641 (78%) were admitted on weekdays, and 183 (22%) on weekends. The 33 excluded charts were similar to the included charts for both time of admission and outcomes. Results for parametric testing and nonparametric testing, as well as for log‐transformation and non‐log‐transformation of the continuous outcomes were similar in both magnitude and statistical significance, so we present the parametric and nonlog‐transformed results below for ease of interpretation.
Interrater reliability among the 3 reviewers was very high. There were no disagreements among the 20 multiple reviews for either poor outcomes within 24 hours of admission or admitting service; the interclass correlation coefficients for 30 day repeat ED visit and 30 day readmission were 0.97 and 0.87, respectively.
Patients admitted at night or on the weekend were similar to patients admitted during the day and week across age, gender, insurance class, MDC, and Charlson Comorbidity Index (Table 1). For unadjusted outcomes, patients admitted at night has a similar LOS, hospital charges, 30 day repeat ED visits, 30 day readmissions, and poor outcome within 24 hours of admission as those patients admitted during the day. They had a potentially lower chance of any ICU transfer during hospitalization though this did not reach statistical significance at P < 0.05 (night admission 6%, day admission 3%, P = 0.06) (Table 2).
Characteristics | Time of Day | Day of the Week | ||
---|---|---|---|---|
Day Admission (n = 345) | Night Admission (n = 479) | Weekday Admission (n = 641) | Weekend Admission (n = 183) | |
| ||||
Age (years) | 60.8 | 59.7 | 60.6 | 58.7 |
Gender (% male) | 47 | 43 | 45 | 46 |
Race/Ethnicity (%) | ||||
White, Asian, other | 61 | 54 | 57 | 55 |
Black | 34 | 38 | 37 | 34 |
Hispanic | 5 | 8 | 6 | 10 |
Medicaid or self pay (%) | 9 | 10 | 10 | 11 |
Major diagnostic category (%) | ||||
Respiratory disease | 14 | 13 | 14 | 13 |
Circulatory disease | 28 | 23 | 26 | 24 |
Digestive disease | 12 | 12 | 12 | 12 |
Other | 45 | 52 | 48 | 51 |
Charlson Comorbidity Index | 3.71 | 3.60 | 3.66 | 3.60 |
Outcomes | Time of Day | Day of the Week | ||
---|---|---|---|---|
Day Admission (n = 345) | Night Admission (n = 479) | Weekday Admission (n = 641) | Weekend Admission (n = 183) | |
| ||||
Length of stay | 4.3 | 4.1 | 4.3 | 3.8 |
Hospital charges | $27,500 | $25,200 | $27,200* | $22,700* |
ICU transfer during hospitalization (%) | 6 | 3 | 5* | 1* |
Repeat ED visit at 30 days (%) | 20 | 22 | 22 | 21 |
Readmission at 30 days (%) | 17 | 20 | 20 | 17 |
Poor outcome at 24 hours (ICU transfer, cardiac arrest, or death)(%) | 2 | 1 | 2 | 1 |
Patients admitted to the hospital during the weekend were similar to patients admitted during the week for unadjusted LOS, 30 day repeat ED visit or readmission rate, and poor outcomes within 24 hours of admission as those admitted during the week; however, they had lower hospital charges (weekend admission $22,700, weekday admission $27,200; P = 0.02), and a lower chance of ICU transfer during hospitalization (weekend admission 1%, weekday admission 5%; P = 0.02) (Table 2).
In the multivariable linear and logistic regression models (Tables 3 and 4), we assessed the independent association between night admission or weekend admission and each hospitalization‐relevant outcome except for poor outcome within 24 hours of admission (poor outcome within 24 hours of admission was not modeled to avoid the risk of overfitting because there were only 13 total events). After adjustment for age, gender, race and ethnicity, admitting service (hospitalist or teaching), Medicaid or self‐pay insurance, MDC, and Charlson Comorbidity Index, there was no statistically significant association between night admission and worse outcomes for LOS, hospital charges, 30 day repeat ED visit, or 30 day readmission. Night admission was associated with a decreased chance of ICU transfer during hospitalization, but the difference was not statistically significant (odds ratio, 0.54; 95% confidence interval [CI], 0.26‐1.11, P = 0.09). Weekend admission was not associated with worse outcomes for LOS or 30 day repeat ED visit or readmission; however, weekend admission was associated with a decrease in overall charges ($4400; 95% CI, $8300 to $600) and a decreased chance of ICU transfer during hospitalization (odds ratio, 0.20; 95% CI, 0.050.88).
Predictors | Length of Stay (days), Coefficient (95% CI) | Hospital Charges (dollars), Coefficient (95% CI) |
---|---|---|
| ||
Night admission | 0.23 (0.77 to 0.32) | 2100 (5400 to 1100) |
Weekend admission | 0.42 (1.07 to 0.23) | 4400 (8300 to 600)* |
Age | 0.01 (0.01 to 0.03) | 0 (100 to 100) |
Male gender | 0.15 (0.70 to 0.39) | 400 (3700 to 2800) |
Race, Black | 0.18 (0.41 to 0.78) | 200 (3700 to 3400) |
Ethnicity, Hispanic | 0.62 (1.73 to 0.49) | 2300 (8900 to 4300) |
Medicaid or self‐pay insurance | 1.87 (0.93 to 2.82)* | 8900 (3300 to 14600)* |
Hospitalist service | 0.26 (0.29 to 0.81) | 600 (3900 to 2700) |
MDC: respiratory | 0.36 (1.18 to 0.46) | 700 (4200 to 5600) |
MDC: circulatory | 1.36 (2.04 to 0.68)* | 600 (4600 to 3400) |
MDC: digestive | 1.22 (2.08 to 0.35)* | 6800 (12000 to 1700)* |
Charlson Comorbidity Index | 0.35 (0.22 to 0.49)* | 2200 (1400 to 3000)* |
Predictors | ICU Transfer during Hospitalization, Odds Ratio (95% CI) | Repeat ED Visit at 30 days, Odds Ratio (95% CI) | Readmission at 30 days, Odds Ratio (95% CI) |
---|---|---|---|
| |||
Night admission | 0.53 (0.26 to 1.11) | 1.13 (0.80 to 1.60) | 1.23 (0.86 to 1.78) |
Weekend admission | 0.20 (0.05 to 0.88)* | 0.95 (0.63 to 1.44) | 0.80 (0.51 to 1.25) |
Age | 1.00 (0.98 to 1.02) | 0.99 (0.98 to 1.002) | 1.00 (0.99 to 1.01) |
Male gender | 0.98 (0.47 to 2.02) | 1.09 (0.78 to 1.54) | 0.91 (0.64 to 1.31) |
Race, Black | 0.75 (0.33 to 1.70) | 1.48 (1.02 to 2.14)* | 1.12 (0.76 to 1.65) |
Ethnicity, Hispanic | 0.76 (0.16 to 3.73) | 1.09 (0.55 to 2.17) | 1.11 (0.55 to 2.22) |
Medicaid or self‐pay insurance | 0.75 (0.16 to 3.49) | 1.61 (0.95 to 2.72) | 2.14 (1.24 to 3.67)* |
Hospitalist service | 0.68 (0.33 to 1.44) | 1.15 (0.81 to 1.63) | 0.99 (0.69 to 1.43) |
MDC: respiratory | 1.18 (0.41 to 3.38) | 1.02 (0.61 to 1.69) | 1.16 (0.69 to 1.95) |
MDC: circulatory | 1.22 (0.52 to 2.87) | 0.79 (0.51 to 1.22) | 0.80 (0.51 to 1.27) |
MDC: digestive | 0.51 (0.11 to 2.32) | 0.83 (0.47 to 1.46) | 1.08 (0.62 to 1.91) |
Charlson Comobrbidity Index | 1.25 (1.09 to 1.45)* | 1.09 (1.01 to 1.19)* | 1.11 (1.02 to 1.21)* |
Our multivariate models explained very little of the variance in patient outcomes. For LOS and hospital charges, adjusted R2 values were 0.06 and 0.05, respectively. For ICU transfer during hospitalization, 30 day repeat ED visit, and 30 day readmission, the areas under the receiver operator curves were 0.75, 0.51, and 0.61 respectively.
To assess the robustness of our conclusions regarding night admission, we redefined night to include only patients admitted between the hours of 8 pm and 5:59 am. This did not change our conclusions. We also tested for interaction between night admission and weekend admission for all outcomes to assess whether night admissions on the weekend were in fact at increased risk of worse outcomes; we found no evidence of interaction (P > 0.3 for the interaction terms in each model).
Discussion
Among patients admitted to the medicine services at our academic medical center, night or weekend admission was not associated with worse hospitalization‐relevant outcomes. In some cases, night or weekend admission was associated with better outcomes, particularly in terms of ICU transfer during hospitalization and hospital charges. Prior research indicates worse outcomes during off‐hours,5 but we did not replicate this finding in our study.
The finding that admission at night was not associated with worse outcomes, particularly proximal outcomes such as LOS or ICU transfer during hospitalization, was surprising, though reassuring in view of the fact that more than half of our patients are admitted at night. We believe a few factors may be responsible. First, our general medicine service is staffed during the night (7 pm to 7 am) by in‐house nocturnists and night float residents. Second, our staffing ratio, while lower at night than during the day, remains the same on weekends and may be higher than in other settings. In continuously well‐staffed settings such as the ED12 and ICU,13 night and weekend admissions are only inconsistently associated with worse outcomes, which may be the same phenomena we observed in the current study. Third, the hospital used as the site of this study has received Nursing Magnet recognition and numerous quality awards such as the National Research Corporation's Consumer Choice Award and recognition as a Distinguished Hospital for Clinical Excellence by HealthGrades. Fourth, our integrated electronic medical record, computerized physician order entry system, and automatically generated sign out serve as complements to the morning hand off. Fifth, hospitalists and teaching teams rotate on a weekly, biweekly, or every 4 week basis, which may protect against discontinuity associated with the weekend. We believe that all of these factors may facilitate alert, comprehensive care during the night and weekend as well as safe and efficient transfer of patients from the night to the day providers.
We were also surprised by the association between weekend admission and lower charges and a lower chance of ICU transfer during hospitalization. We believe many of the same factors noted above may have played a role in these findings. In terms of hospital charges, it is possible that some workups were completed outside of the hospital rather than during the hospitalization, and that some tests were not ordered at all due to unavailability on weekends. The decreased chance of ICU transfer is unexplained. We hypothesize that there may have been a more conservative admission strategy within the ED, such that patients with high baseline severity were admitted directly to the ICU on the weekend rather than being admitted first to the general medicine floor. This hypothesis requires further study.
Our study had important limitations. It was a retrospective study from a single academic hospital. The sample size lacked sufficient power to detect differences in the low frequency of certain outcomes such as poor outcomes within 24 hours of admission (2% vs. 1%), and also for more frequent outcomes such as 30 day readmission; it is possible that with a larger sample there would have been statistically significant differences. Further, we recognize that the Charlson Comorbidity Index, which was developed to predict 1‐year mortality for medicine service patients, does not adjust for severity of illness at presentation, particularly for outcomes such as readmission. If patients admitted at night and during the weekend were less acutely ill despite having similar comorbidities and MDCs at admission, true associations between time of admission and worse outcomes could have been masked. Furthermore, the multivariable modeling explained very little of the variance in patient outcomes such that significant unmeasured confounding may still be present, and consequently our results cannot be interpreted in a causal way. Data was collected from electronic records, so it is possible that some adverse events were not recorded. However, it seems unlikely that major events such as death and transfer to an ICU would have been missed.
Several aspects of the study strengthen our confidence in the findings, including a large sample size, relevance of the outcomes, the adjustment for confounders, and an assessment for robustness of the conclusions based on restricting the definition of night and also testing for interaction between night and weekend admission. Our patient demographics and insurance mix resemble that of other academic hospitals,10 and perhaps our results may be generalizable to these settings, if not to non‐urban or community hospitals. Furthermore, the Charlson Comorbidity Index was associated with all 5 of the modeled outcomes we chose for our study, reaffirming their utility in assessing the quality of hospital care. Future directions for investigation may include examining the association of night admission with hospitalization‐relevant outcomes in nonacademic, nonurban settings, and examining whether the lack of association between night and weekend admission and worse outcomes persists with adjustment for initial severity of illness.
In summary, at a large, well‐staffed urban academic hospital, day or time of admission were not associated with worse hospitalization‐relevant outcomes. The use of nocturnists and night float teams for night admissions and continuity across weekends appears to be a safe approach to handling the increased volume of patients admitted at night, and a viable alternative to overnight call in the era of work hour restrictions.
- Three‐year results of mandated work hour restrictions: attending and resident perspectives and effects in a community hospital.Am Surg.2008;74(6):542–546; discussion 546–547. , , , et al.
- Handoffs causing patient harm: a survey of medical and surgical house staff.Jt Comm J Qual Patient Saf.2008;34(10):563–570. , , , et al.
- Does housestaff discontinuity of care increase the risk for preventable adverse events?Ann Intern Med.1994;121(11):866–872. , , , , .
- Mortality among patients admitted to hospitals on weekends as compared with weekdays.N Engl J Med.2001;345(9):663–668. , .
- Survival from in‐hospital cardiac arrest during nights and weekends.JAMA.2008;299(7):785–792. , , , et al.
- Continuity of care and intensive care unit use at the end of life.Arch Intern Med.2009;169(1):81–86. , , , .
- Continuity of outpatient and inpatient care by primary care physicians for hospitalized older adults.JAMA.2009;301(16):1671–1680. , , , , , .
- Why predictive indexes perform less well in validation studies: is it magic or methods?Arch Intern Med.1987;147:2155–2161. , , , .
- Adapting a clinical comorbidity index for use with ICD‐9‐CM administrative databases.J Clin Epidemiol.1992;45(6):613–619. , , .
- Implementation of a physician assistant/hospitalist service in an academic medical center: impact on efficiency and patient outcomes.J Hosp Med.2008;3(5):361–368. , , , et al.
- Use of an admission early warning score to predict patient morbidity and mortality and treatment success.Emerg Med J.2008;25(12):803–806. , , , et al.
- The impact of weekends on outcome for emergency patients.Clin Med.2005;5(6):621–625. , , .
- Off hour admission to an intensivist‐led ICU is not associated with increased mortality.Crit Care.2009;13(3):R84. , , , , , .
The hospitalist movement and increasingly stringent resident work hour restrictions have led to the utilization of shift work in many hospitals.1 Use of nocturnist and night float systems, while often necessary, results in increased patient hand‐offs. Research suggests that hand‐offs in the inpatient setting can adversely affect patient outcomes as lack of continuity may increase the possibility of medical error.2, 3 In 2001, Bell et al.4 found that mortality was higher among patients admitted on weekends as compared to weekdays. Uneven staffing, lack of supervision, and fragmented care were cited as potential contributing factors.4 Similarly, Peberdy et al.5 in 2008 revealed that patients were less likely to survive a cardiac arrest if it occurred at night or on weekends, again attributed in part to fragmented patient care and understaffing.
The results of these studies raise concerns as to whether increased reliance on shift work and resulting handoffs compromises patient care.6, 7 The aim of this study was to evaluate the potential association between night admission and hospitalization‐relevant outcomes (length of stay [LOS], hospital charges, intensive care unit [ICU] transfer during hospitalization, repeat emergency department [ED] visit within 30 days of discharge, readmission within 30 days of discharge, and poor outcome [transfer to the ICU, cardiac arrest, or death] within the first 24 hours of admission) at an institution that exclusively uses nocturnists (night‐shift based hospitalists) and a resident night float system for patients admitted at night to the general medicine service. A secondary aim was to determine the potential association between weekend admission and hospitalization‐relevant outcomes.
Methods
Study Sample and Selection
We conducted a retrospective medical record review at a large urban academic hospital. Using an administrative hospital data set, we assembled a list of approximately 9000 admissions to the general medicine service from the ED between January 2008 and October 2008. We sampled consecutive admissions from 3 distinct periods beginning in January, April, and July to capture outcomes at various points in the academic year. We attempted to review approximately 10% of all charts equally distributed among the 3 sampling periods (ie, 900 charts total with one‐third from each period) based on time available to the reviewers. We excluded patients not admitted to the general medicine service and patients without complete demographic or outcome information. We also excluded patients not admitted from the ED given that the vast majority of admissions to our hospital during the night (96%) or weekend (93%) are from the ED. Patients admitted to the general medicine service are cared for either by a hospitalist or by a teaching team comprised of 1 attending (about 40% of whom are hospitalists), 1 resident, 1 to 2 interns, and 1 to 3 medical students. From 7 am to 6:59 pm patients are admitted to the care of 1 of the primary daytime admitting teams. From 7 pm to 6:59 am patients are admitted by nocturnists (hospitalist service) or night float residents (teaching service). These patients are handed off to day teams at 7 am. Hospitalist teams change service on a weekly to biweekly basis and resident teams switch on a monthly basis; there is no difference in physician staffing between the weekend and weekdays. The Northwestern University Institutional Review Board approved this study.
Data Acquisition and Medical Records Reviews
We obtained demographic data including gender, age, race and ethnicity, patient insurance, admission day (weekday vs. weekend), admission time (defined as the time that a patient receives a hospital bed, which at our institution is also the time that admitting teams receive report and assume care for the patient), and the International Classification of Disease codes required to determine the Major Diagnostic Category (MDC) and calculate the Charlson Comorbidity Index8, 9 as part of an administrative data set. We divided the admission time into night admission (defined as 7 pm to 6:59 am) and day admission (defined as 7:00 am to 6:59 pm). We created a chart abstraction tool to allow manual recording of the additional fields of admitting team (hospitalist vs. resident), 30 day repeat ED visit, 30 day readmission, and poor outcomes within the first 24 hours of admission, directly from the electronic record.
Study Outcomes
We evaluated each admission for the following 6 primary outcomes which were specified a priori: LOS (defined as discharge date and time minus admission date and time), hospital charges (defined as charges billed as recorded in the administrative data set), ICU transfer during hospitalization (defined as 1 ICU day in the administrative data set), 30 day repeat ED visit (defined as a visit to our ED within 30 days of discharge as assessed by chart abstraction), 30 day readmission (defined as any planned or unplanned admission to any inpatient service at our institution within 30 days of discharge as assessed by chart abstraction), and poor outcome within 24 hours of admission (defined as transfer to the ICU, cardiac arrest, or death as assessed by chart abstraction). Each of these outcomes has been used in prior work to assess the quality of inpatient care.10, 11
Statistical Analysis
Interrater reliability between the 3 physician reviewers was assessed for 20 randomly selected admissions across the 4 separate review measures using interclass correlation coefficients. Comparisons between night admissions and day admissions, and between weekend and weekday admissions, for the continuous primary outcomes (LOS, hospital charges) were assessed using 2‐tailed t‐tests as well as Wilcoxon rank sum test. In the multivariable modeling, these outcomes were assessed by linear regression controlling for age, gender, race and ethnicity, Medicaid or self‐pay insurance, admission to the hospitalist or teaching service, most common MDC categories, and Charlson Comorbidity Index. Because both outcomes were right‐skewed, we separately assessed each after log‐transformation controlling for the same variables.
All comparisons of the dichotomous primary outcomes (ICU transfer during hospitalization, 30 day repeat ED visit, 30 day readmission, and poor outcome within the first 24 hours after admission) were assessed at the univariate level by chi‐squared test, and in the multivariable models using logistic regression, controlling for the same variables as the linear models above. All adjustments were specified a priori. All data analyses were conducted using Stata (College Station, TX; Version 11).
Results
We reviewed 857 records. After excluding 33 records lacking administrative data regarding gender, race and ethnicity, and other demographic variables, there were 824 medical records available for analysis. We reviewed a similar number of records from each time period: 274 from January 2008, 265 from April 2008, and 285 from July 2008. A total of 345 (42%) patients were admitted during the day, and 479 (58%) at night; 641 (78%) were admitted on weekdays, and 183 (22%) on weekends. The 33 excluded charts were similar to the included charts for both time of admission and outcomes. Results for parametric testing and nonparametric testing, as well as for log‐transformation and non‐log‐transformation of the continuous outcomes were similar in both magnitude and statistical significance, so we present the parametric and nonlog‐transformed results below for ease of interpretation.
Interrater reliability among the 3 reviewers was very high. There were no disagreements among the 20 multiple reviews for either poor outcomes within 24 hours of admission or admitting service; the interclass correlation coefficients for 30 day repeat ED visit and 30 day readmission were 0.97 and 0.87, respectively.
Patients admitted at night or on the weekend were similar to patients admitted during the day and week across age, gender, insurance class, MDC, and Charlson Comorbidity Index (Table 1). For unadjusted outcomes, patients admitted at night has a similar LOS, hospital charges, 30 day repeat ED visits, 30 day readmissions, and poor outcome within 24 hours of admission as those patients admitted during the day. They had a potentially lower chance of any ICU transfer during hospitalization though this did not reach statistical significance at P < 0.05 (night admission 6%, day admission 3%, P = 0.06) (Table 2).
Characteristics | Time of Day | Day of the Week | ||
---|---|---|---|---|
Day Admission (n = 345) | Night Admission (n = 479) | Weekday Admission (n = 641) | Weekend Admission (n = 183) | |
| ||||
Age (years) | 60.8 | 59.7 | 60.6 | 58.7 |
Gender (% male) | 47 | 43 | 45 | 46 |
Race/Ethnicity (%) | ||||
White, Asian, other | 61 | 54 | 57 | 55 |
Black | 34 | 38 | 37 | 34 |
Hispanic | 5 | 8 | 6 | 10 |
Medicaid or self pay (%) | 9 | 10 | 10 | 11 |
Major diagnostic category (%) | ||||
Respiratory disease | 14 | 13 | 14 | 13 |
Circulatory disease | 28 | 23 | 26 | 24 |
Digestive disease | 12 | 12 | 12 | 12 |
Other | 45 | 52 | 48 | 51 |
Charlson Comorbidity Index | 3.71 | 3.60 | 3.66 | 3.60 |
Outcomes | Time of Day | Day of the Week | ||
---|---|---|---|---|
Day Admission (n = 345) | Night Admission (n = 479) | Weekday Admission (n = 641) | Weekend Admission (n = 183) | |
| ||||
Length of stay | 4.3 | 4.1 | 4.3 | 3.8 |
Hospital charges | $27,500 | $25,200 | $27,200* | $22,700* |
ICU transfer during hospitalization (%) | 6 | 3 | 5* | 1* |
Repeat ED visit at 30 days (%) | 20 | 22 | 22 | 21 |
Readmission at 30 days (%) | 17 | 20 | 20 | 17 |
Poor outcome at 24 hours (ICU transfer, cardiac arrest, or death)(%) | 2 | 1 | 2 | 1 |
Patients admitted to the hospital during the weekend were similar to patients admitted during the week for unadjusted LOS, 30 day repeat ED visit or readmission rate, and poor outcomes within 24 hours of admission as those admitted during the week; however, they had lower hospital charges (weekend admission $22,700, weekday admission $27,200; P = 0.02), and a lower chance of ICU transfer during hospitalization (weekend admission 1%, weekday admission 5%; P = 0.02) (Table 2).
In the multivariable linear and logistic regression models (Tables 3 and 4), we assessed the independent association between night admission or weekend admission and each hospitalization‐relevant outcome except for poor outcome within 24 hours of admission (poor outcome within 24 hours of admission was not modeled to avoid the risk of overfitting because there were only 13 total events). After adjustment for age, gender, race and ethnicity, admitting service (hospitalist or teaching), Medicaid or self‐pay insurance, MDC, and Charlson Comorbidity Index, there was no statistically significant association between night admission and worse outcomes for LOS, hospital charges, 30 day repeat ED visit, or 30 day readmission. Night admission was associated with a decreased chance of ICU transfer during hospitalization, but the difference was not statistically significant (odds ratio, 0.54; 95% confidence interval [CI], 0.26‐1.11, P = 0.09). Weekend admission was not associated with worse outcomes for LOS or 30 day repeat ED visit or readmission; however, weekend admission was associated with a decrease in overall charges ($4400; 95% CI, $8300 to $600) and a decreased chance of ICU transfer during hospitalization (odds ratio, 0.20; 95% CI, 0.050.88).
Predictors | Length of Stay (days), Coefficient (95% CI) | Hospital Charges (dollars), Coefficient (95% CI) |
---|---|---|
| ||
Night admission | 0.23 (0.77 to 0.32) | 2100 (5400 to 1100) |
Weekend admission | 0.42 (1.07 to 0.23) | 4400 (8300 to 600)* |
Age | 0.01 (0.01 to 0.03) | 0 (100 to 100) |
Male gender | 0.15 (0.70 to 0.39) | 400 (3700 to 2800) |
Race, Black | 0.18 (0.41 to 0.78) | 200 (3700 to 3400) |
Ethnicity, Hispanic | 0.62 (1.73 to 0.49) | 2300 (8900 to 4300) |
Medicaid or self‐pay insurance | 1.87 (0.93 to 2.82)* | 8900 (3300 to 14600)* |
Hospitalist service | 0.26 (0.29 to 0.81) | 600 (3900 to 2700) |
MDC: respiratory | 0.36 (1.18 to 0.46) | 700 (4200 to 5600) |
MDC: circulatory | 1.36 (2.04 to 0.68)* | 600 (4600 to 3400) |
MDC: digestive | 1.22 (2.08 to 0.35)* | 6800 (12000 to 1700)* |
Charlson Comorbidity Index | 0.35 (0.22 to 0.49)* | 2200 (1400 to 3000)* |
Predictors | ICU Transfer during Hospitalization, Odds Ratio (95% CI) | Repeat ED Visit at 30 days, Odds Ratio (95% CI) | Readmission at 30 days, Odds Ratio (95% CI) |
---|---|---|---|
| |||
Night admission | 0.53 (0.26 to 1.11) | 1.13 (0.80 to 1.60) | 1.23 (0.86 to 1.78) |
Weekend admission | 0.20 (0.05 to 0.88)* | 0.95 (0.63 to 1.44) | 0.80 (0.51 to 1.25) |
Age | 1.00 (0.98 to 1.02) | 0.99 (0.98 to 1.002) | 1.00 (0.99 to 1.01) |
Male gender | 0.98 (0.47 to 2.02) | 1.09 (0.78 to 1.54) | 0.91 (0.64 to 1.31) |
Race, Black | 0.75 (0.33 to 1.70) | 1.48 (1.02 to 2.14)* | 1.12 (0.76 to 1.65) |
Ethnicity, Hispanic | 0.76 (0.16 to 3.73) | 1.09 (0.55 to 2.17) | 1.11 (0.55 to 2.22) |
Medicaid or self‐pay insurance | 0.75 (0.16 to 3.49) | 1.61 (0.95 to 2.72) | 2.14 (1.24 to 3.67)* |
Hospitalist service | 0.68 (0.33 to 1.44) | 1.15 (0.81 to 1.63) | 0.99 (0.69 to 1.43) |
MDC: respiratory | 1.18 (0.41 to 3.38) | 1.02 (0.61 to 1.69) | 1.16 (0.69 to 1.95) |
MDC: circulatory | 1.22 (0.52 to 2.87) | 0.79 (0.51 to 1.22) | 0.80 (0.51 to 1.27) |
MDC: digestive | 0.51 (0.11 to 2.32) | 0.83 (0.47 to 1.46) | 1.08 (0.62 to 1.91) |
Charlson Comobrbidity Index | 1.25 (1.09 to 1.45)* | 1.09 (1.01 to 1.19)* | 1.11 (1.02 to 1.21)* |
Our multivariate models explained very little of the variance in patient outcomes. For LOS and hospital charges, adjusted R2 values were 0.06 and 0.05, respectively. For ICU transfer during hospitalization, 30 day repeat ED visit, and 30 day readmission, the areas under the receiver operator curves were 0.75, 0.51, and 0.61 respectively.
To assess the robustness of our conclusions regarding night admission, we redefined night to include only patients admitted between the hours of 8 pm and 5:59 am. This did not change our conclusions. We also tested for interaction between night admission and weekend admission for all outcomes to assess whether night admissions on the weekend were in fact at increased risk of worse outcomes; we found no evidence of interaction (P > 0.3 for the interaction terms in each model).
Discussion
Among patients admitted to the medicine services at our academic medical center, night or weekend admission was not associated with worse hospitalization‐relevant outcomes. In some cases, night or weekend admission was associated with better outcomes, particularly in terms of ICU transfer during hospitalization and hospital charges. Prior research indicates worse outcomes during off‐hours,5 but we did not replicate this finding in our study.
The finding that admission at night was not associated with worse outcomes, particularly proximal outcomes such as LOS or ICU transfer during hospitalization, was surprising, though reassuring in view of the fact that more than half of our patients are admitted at night. We believe a few factors may be responsible. First, our general medicine service is staffed during the night (7 pm to 7 am) by in‐house nocturnists and night float residents. Second, our staffing ratio, while lower at night than during the day, remains the same on weekends and may be higher than in other settings. In continuously well‐staffed settings such as the ED12 and ICU,13 night and weekend admissions are only inconsistently associated with worse outcomes, which may be the same phenomena we observed in the current study. Third, the hospital used as the site of this study has received Nursing Magnet recognition and numerous quality awards such as the National Research Corporation's Consumer Choice Award and recognition as a Distinguished Hospital for Clinical Excellence by HealthGrades. Fourth, our integrated electronic medical record, computerized physician order entry system, and automatically generated sign out serve as complements to the morning hand off. Fifth, hospitalists and teaching teams rotate on a weekly, biweekly, or every 4 week basis, which may protect against discontinuity associated with the weekend. We believe that all of these factors may facilitate alert, comprehensive care during the night and weekend as well as safe and efficient transfer of patients from the night to the day providers.
We were also surprised by the association between weekend admission and lower charges and a lower chance of ICU transfer during hospitalization. We believe many of the same factors noted above may have played a role in these findings. In terms of hospital charges, it is possible that some workups were completed outside of the hospital rather than during the hospitalization, and that some tests were not ordered at all due to unavailability on weekends. The decreased chance of ICU transfer is unexplained. We hypothesize that there may have been a more conservative admission strategy within the ED, such that patients with high baseline severity were admitted directly to the ICU on the weekend rather than being admitted first to the general medicine floor. This hypothesis requires further study.
Our study had important limitations. It was a retrospective study from a single academic hospital. The sample size lacked sufficient power to detect differences in the low frequency of certain outcomes such as poor outcomes within 24 hours of admission (2% vs. 1%), and also for more frequent outcomes such as 30 day readmission; it is possible that with a larger sample there would have been statistically significant differences. Further, we recognize that the Charlson Comorbidity Index, which was developed to predict 1‐year mortality for medicine service patients, does not adjust for severity of illness at presentation, particularly for outcomes such as readmission. If patients admitted at night and during the weekend were less acutely ill despite having similar comorbidities and MDCs at admission, true associations between time of admission and worse outcomes could have been masked. Furthermore, the multivariable modeling explained very little of the variance in patient outcomes such that significant unmeasured confounding may still be present, and consequently our results cannot be interpreted in a causal way. Data was collected from electronic records, so it is possible that some adverse events were not recorded. However, it seems unlikely that major events such as death and transfer to an ICU would have been missed.
Several aspects of the study strengthen our confidence in the findings, including a large sample size, relevance of the outcomes, the adjustment for confounders, and an assessment for robustness of the conclusions based on restricting the definition of night and also testing for interaction between night and weekend admission. Our patient demographics and insurance mix resemble that of other academic hospitals,10 and perhaps our results may be generalizable to these settings, if not to non‐urban or community hospitals. Furthermore, the Charlson Comorbidity Index was associated with all 5 of the modeled outcomes we chose for our study, reaffirming their utility in assessing the quality of hospital care. Future directions for investigation may include examining the association of night admission with hospitalization‐relevant outcomes in nonacademic, nonurban settings, and examining whether the lack of association between night and weekend admission and worse outcomes persists with adjustment for initial severity of illness.
In summary, at a large, well‐staffed urban academic hospital, day or time of admission were not associated with worse hospitalization‐relevant outcomes. The use of nocturnists and night float teams for night admissions and continuity across weekends appears to be a safe approach to handling the increased volume of patients admitted at night, and a viable alternative to overnight call in the era of work hour restrictions.
The hospitalist movement and increasingly stringent resident work hour restrictions have led to the utilization of shift work in many hospitals.1 Use of nocturnist and night float systems, while often necessary, results in increased patient hand‐offs. Research suggests that hand‐offs in the inpatient setting can adversely affect patient outcomes as lack of continuity may increase the possibility of medical error.2, 3 In 2001, Bell et al.4 found that mortality was higher among patients admitted on weekends as compared to weekdays. Uneven staffing, lack of supervision, and fragmented care were cited as potential contributing factors.4 Similarly, Peberdy et al.5 in 2008 revealed that patients were less likely to survive a cardiac arrest if it occurred at night or on weekends, again attributed in part to fragmented patient care and understaffing.
The results of these studies raise concerns as to whether increased reliance on shift work and resulting handoffs compromises patient care.6, 7 The aim of this study was to evaluate the potential association between night admission and hospitalization‐relevant outcomes (length of stay [LOS], hospital charges, intensive care unit [ICU] transfer during hospitalization, repeat emergency department [ED] visit within 30 days of discharge, readmission within 30 days of discharge, and poor outcome [transfer to the ICU, cardiac arrest, or death] within the first 24 hours of admission) at an institution that exclusively uses nocturnists (night‐shift based hospitalists) and a resident night float system for patients admitted at night to the general medicine service. A secondary aim was to determine the potential association between weekend admission and hospitalization‐relevant outcomes.
Methods
Study Sample and Selection
We conducted a retrospective medical record review at a large urban academic hospital. Using an administrative hospital data set, we assembled a list of approximately 9000 admissions to the general medicine service from the ED between January 2008 and October 2008. We sampled consecutive admissions from 3 distinct periods beginning in January, April, and July to capture outcomes at various points in the academic year. We attempted to review approximately 10% of all charts equally distributed among the 3 sampling periods (ie, 900 charts total with one‐third from each period) based on time available to the reviewers. We excluded patients not admitted to the general medicine service and patients without complete demographic or outcome information. We also excluded patients not admitted from the ED given that the vast majority of admissions to our hospital during the night (96%) or weekend (93%) are from the ED. Patients admitted to the general medicine service are cared for either by a hospitalist or by a teaching team comprised of 1 attending (about 40% of whom are hospitalists), 1 resident, 1 to 2 interns, and 1 to 3 medical students. From 7 am to 6:59 pm patients are admitted to the care of 1 of the primary daytime admitting teams. From 7 pm to 6:59 am patients are admitted by nocturnists (hospitalist service) or night float residents (teaching service). These patients are handed off to day teams at 7 am. Hospitalist teams change service on a weekly to biweekly basis and resident teams switch on a monthly basis; there is no difference in physician staffing between the weekend and weekdays. The Northwestern University Institutional Review Board approved this study.
Data Acquisition and Medical Records Reviews
We obtained demographic data including gender, age, race and ethnicity, patient insurance, admission day (weekday vs. weekend), admission time (defined as the time that a patient receives a hospital bed, which at our institution is also the time that admitting teams receive report and assume care for the patient), and the International Classification of Disease codes required to determine the Major Diagnostic Category (MDC) and calculate the Charlson Comorbidity Index8, 9 as part of an administrative data set. We divided the admission time into night admission (defined as 7 pm to 6:59 am) and day admission (defined as 7:00 am to 6:59 pm). We created a chart abstraction tool to allow manual recording of the additional fields of admitting team (hospitalist vs. resident), 30 day repeat ED visit, 30 day readmission, and poor outcomes within the first 24 hours of admission, directly from the electronic record.
Study Outcomes
We evaluated each admission for the following 6 primary outcomes which were specified a priori: LOS (defined as discharge date and time minus admission date and time), hospital charges (defined as charges billed as recorded in the administrative data set), ICU transfer during hospitalization (defined as 1 ICU day in the administrative data set), 30 day repeat ED visit (defined as a visit to our ED within 30 days of discharge as assessed by chart abstraction), 30 day readmission (defined as any planned or unplanned admission to any inpatient service at our institution within 30 days of discharge as assessed by chart abstraction), and poor outcome within 24 hours of admission (defined as transfer to the ICU, cardiac arrest, or death as assessed by chart abstraction). Each of these outcomes has been used in prior work to assess the quality of inpatient care.10, 11
Statistical Analysis
Interrater reliability between the 3 physician reviewers was assessed for 20 randomly selected admissions across the 4 separate review measures using interclass correlation coefficients. Comparisons between night admissions and day admissions, and between weekend and weekday admissions, for the continuous primary outcomes (LOS, hospital charges) were assessed using 2‐tailed t‐tests as well as Wilcoxon rank sum test. In the multivariable modeling, these outcomes were assessed by linear regression controlling for age, gender, race and ethnicity, Medicaid or self‐pay insurance, admission to the hospitalist or teaching service, most common MDC categories, and Charlson Comorbidity Index. Because both outcomes were right‐skewed, we separately assessed each after log‐transformation controlling for the same variables.
All comparisons of the dichotomous primary outcomes (ICU transfer during hospitalization, 30 day repeat ED visit, 30 day readmission, and poor outcome within the first 24 hours after admission) were assessed at the univariate level by chi‐squared test, and in the multivariable models using logistic regression, controlling for the same variables as the linear models above. All adjustments were specified a priori. All data analyses were conducted using Stata (College Station, TX; Version 11).
Results
We reviewed 857 records. After excluding 33 records lacking administrative data regarding gender, race and ethnicity, and other demographic variables, there were 824 medical records available for analysis. We reviewed a similar number of records from each time period: 274 from January 2008, 265 from April 2008, and 285 from July 2008. A total of 345 (42%) patients were admitted during the day, and 479 (58%) at night; 641 (78%) were admitted on weekdays, and 183 (22%) on weekends. The 33 excluded charts were similar to the included charts for both time of admission and outcomes. Results for parametric testing and nonparametric testing, as well as for log‐transformation and non‐log‐transformation of the continuous outcomes were similar in both magnitude and statistical significance, so we present the parametric and nonlog‐transformed results below for ease of interpretation.
Interrater reliability among the 3 reviewers was very high. There were no disagreements among the 20 multiple reviews for either poor outcomes within 24 hours of admission or admitting service; the interclass correlation coefficients for 30 day repeat ED visit and 30 day readmission were 0.97 and 0.87, respectively.
Patients admitted at night or on the weekend were similar to patients admitted during the day and week across age, gender, insurance class, MDC, and Charlson Comorbidity Index (Table 1). For unadjusted outcomes, patients admitted at night has a similar LOS, hospital charges, 30 day repeat ED visits, 30 day readmissions, and poor outcome within 24 hours of admission as those patients admitted during the day. They had a potentially lower chance of any ICU transfer during hospitalization though this did not reach statistical significance at P < 0.05 (night admission 6%, day admission 3%, P = 0.06) (Table 2).
Characteristics | Time of Day | Day of the Week | ||
---|---|---|---|---|
Day Admission (n = 345) | Night Admission (n = 479) | Weekday Admission (n = 641) | Weekend Admission (n = 183) | |
| ||||
Age (years) | 60.8 | 59.7 | 60.6 | 58.7 |
Gender (% male) | 47 | 43 | 45 | 46 |
Race/Ethnicity (%) | ||||
White, Asian, other | 61 | 54 | 57 | 55 |
Black | 34 | 38 | 37 | 34 |
Hispanic | 5 | 8 | 6 | 10 |
Medicaid or self pay (%) | 9 | 10 | 10 | 11 |
Major diagnostic category (%) | ||||
Respiratory disease | 14 | 13 | 14 | 13 |
Circulatory disease | 28 | 23 | 26 | 24 |
Digestive disease | 12 | 12 | 12 | 12 |
Other | 45 | 52 | 48 | 51 |
Charlson Comorbidity Index | 3.71 | 3.60 | 3.66 | 3.60 |
Outcomes | Time of Day | Day of the Week | ||
---|---|---|---|---|
Day Admission (n = 345) | Night Admission (n = 479) | Weekday Admission (n = 641) | Weekend Admission (n = 183) | |
| ||||
Length of stay | 4.3 | 4.1 | 4.3 | 3.8 |
Hospital charges | $27,500 | $25,200 | $27,200* | $22,700* |
ICU transfer during hospitalization (%) | 6 | 3 | 5* | 1* |
Repeat ED visit at 30 days (%) | 20 | 22 | 22 | 21 |
Readmission at 30 days (%) | 17 | 20 | 20 | 17 |
Poor outcome at 24 hours (ICU transfer, cardiac arrest, or death)(%) | 2 | 1 | 2 | 1 |
Patients admitted to the hospital during the weekend were similar to patients admitted during the week for unadjusted LOS, 30 day repeat ED visit or readmission rate, and poor outcomes within 24 hours of admission as those admitted during the week; however, they had lower hospital charges (weekend admission $22,700, weekday admission $27,200; P = 0.02), and a lower chance of ICU transfer during hospitalization (weekend admission 1%, weekday admission 5%; P = 0.02) (Table 2).
In the multivariable linear and logistic regression models (Tables 3 and 4), we assessed the independent association between night admission or weekend admission and each hospitalization‐relevant outcome except for poor outcome within 24 hours of admission (poor outcome within 24 hours of admission was not modeled to avoid the risk of overfitting because there were only 13 total events). After adjustment for age, gender, race and ethnicity, admitting service (hospitalist or teaching), Medicaid or self‐pay insurance, MDC, and Charlson Comorbidity Index, there was no statistically significant association between night admission and worse outcomes for LOS, hospital charges, 30 day repeat ED visit, or 30 day readmission. Night admission was associated with a decreased chance of ICU transfer during hospitalization, but the difference was not statistically significant (odds ratio, 0.54; 95% confidence interval [CI], 0.26‐1.11, P = 0.09). Weekend admission was not associated with worse outcomes for LOS or 30 day repeat ED visit or readmission; however, weekend admission was associated with a decrease in overall charges ($4400; 95% CI, $8300 to $600) and a decreased chance of ICU transfer during hospitalization (odds ratio, 0.20; 95% CI, 0.050.88).
Predictors | Length of Stay (days), Coefficient (95% CI) | Hospital Charges (dollars), Coefficient (95% CI) |
---|---|---|
| ||
Night admission | 0.23 (0.77 to 0.32) | 2100 (5400 to 1100) |
Weekend admission | 0.42 (1.07 to 0.23) | 4400 (8300 to 600)* |
Age | 0.01 (0.01 to 0.03) | 0 (100 to 100) |
Male gender | 0.15 (0.70 to 0.39) | 400 (3700 to 2800) |
Race, Black | 0.18 (0.41 to 0.78) | 200 (3700 to 3400) |
Ethnicity, Hispanic | 0.62 (1.73 to 0.49) | 2300 (8900 to 4300) |
Medicaid or self‐pay insurance | 1.87 (0.93 to 2.82)* | 8900 (3300 to 14600)* |
Hospitalist service | 0.26 (0.29 to 0.81) | 600 (3900 to 2700) |
MDC: respiratory | 0.36 (1.18 to 0.46) | 700 (4200 to 5600) |
MDC: circulatory | 1.36 (2.04 to 0.68)* | 600 (4600 to 3400) |
MDC: digestive | 1.22 (2.08 to 0.35)* | 6800 (12000 to 1700)* |
Charlson Comorbidity Index | 0.35 (0.22 to 0.49)* | 2200 (1400 to 3000)* |
Predictors | ICU Transfer during Hospitalization, Odds Ratio (95% CI) | Repeat ED Visit at 30 days, Odds Ratio (95% CI) | Readmission at 30 days, Odds Ratio (95% CI) |
---|---|---|---|
| |||
Night admission | 0.53 (0.26 to 1.11) | 1.13 (0.80 to 1.60) | 1.23 (0.86 to 1.78) |
Weekend admission | 0.20 (0.05 to 0.88)* | 0.95 (0.63 to 1.44) | 0.80 (0.51 to 1.25) |
Age | 1.00 (0.98 to 1.02) | 0.99 (0.98 to 1.002) | 1.00 (0.99 to 1.01) |
Male gender | 0.98 (0.47 to 2.02) | 1.09 (0.78 to 1.54) | 0.91 (0.64 to 1.31) |
Race, Black | 0.75 (0.33 to 1.70) | 1.48 (1.02 to 2.14)* | 1.12 (0.76 to 1.65) |
Ethnicity, Hispanic | 0.76 (0.16 to 3.73) | 1.09 (0.55 to 2.17) | 1.11 (0.55 to 2.22) |
Medicaid or self‐pay insurance | 0.75 (0.16 to 3.49) | 1.61 (0.95 to 2.72) | 2.14 (1.24 to 3.67)* |
Hospitalist service | 0.68 (0.33 to 1.44) | 1.15 (0.81 to 1.63) | 0.99 (0.69 to 1.43) |
MDC: respiratory | 1.18 (0.41 to 3.38) | 1.02 (0.61 to 1.69) | 1.16 (0.69 to 1.95) |
MDC: circulatory | 1.22 (0.52 to 2.87) | 0.79 (0.51 to 1.22) | 0.80 (0.51 to 1.27) |
MDC: digestive | 0.51 (0.11 to 2.32) | 0.83 (0.47 to 1.46) | 1.08 (0.62 to 1.91) |
Charlson Comobrbidity Index | 1.25 (1.09 to 1.45)* | 1.09 (1.01 to 1.19)* | 1.11 (1.02 to 1.21)* |
Our multivariate models explained very little of the variance in patient outcomes. For LOS and hospital charges, adjusted R2 values were 0.06 and 0.05, respectively. For ICU transfer during hospitalization, 30 day repeat ED visit, and 30 day readmission, the areas under the receiver operator curves were 0.75, 0.51, and 0.61 respectively.
To assess the robustness of our conclusions regarding night admission, we redefined night to include only patients admitted between the hours of 8 pm and 5:59 am. This did not change our conclusions. We also tested for interaction between night admission and weekend admission for all outcomes to assess whether night admissions on the weekend were in fact at increased risk of worse outcomes; we found no evidence of interaction (P > 0.3 for the interaction terms in each model).
Discussion
Among patients admitted to the medicine services at our academic medical center, night or weekend admission was not associated with worse hospitalization‐relevant outcomes. In some cases, night or weekend admission was associated with better outcomes, particularly in terms of ICU transfer during hospitalization and hospital charges. Prior research indicates worse outcomes during off‐hours,5 but we did not replicate this finding in our study.
The finding that admission at night was not associated with worse outcomes, particularly proximal outcomes such as LOS or ICU transfer during hospitalization, was surprising, though reassuring in view of the fact that more than half of our patients are admitted at night. We believe a few factors may be responsible. First, our general medicine service is staffed during the night (7 pm to 7 am) by in‐house nocturnists and night float residents. Second, our staffing ratio, while lower at night than during the day, remains the same on weekends and may be higher than in other settings. In continuously well‐staffed settings such as the ED12 and ICU,13 night and weekend admissions are only inconsistently associated with worse outcomes, which may be the same phenomena we observed in the current study. Third, the hospital used as the site of this study has received Nursing Magnet recognition and numerous quality awards such as the National Research Corporation's Consumer Choice Award and recognition as a Distinguished Hospital for Clinical Excellence by HealthGrades. Fourth, our integrated electronic medical record, computerized physician order entry system, and automatically generated sign out serve as complements to the morning hand off. Fifth, hospitalists and teaching teams rotate on a weekly, biweekly, or every 4 week basis, which may protect against discontinuity associated with the weekend. We believe that all of these factors may facilitate alert, comprehensive care during the night and weekend as well as safe and efficient transfer of patients from the night to the day providers.
We were also surprised by the association between weekend admission and lower charges and a lower chance of ICU transfer during hospitalization. We believe many of the same factors noted above may have played a role in these findings. In terms of hospital charges, it is possible that some workups were completed outside of the hospital rather than during the hospitalization, and that some tests were not ordered at all due to unavailability on weekends. The decreased chance of ICU transfer is unexplained. We hypothesize that there may have been a more conservative admission strategy within the ED, such that patients with high baseline severity were admitted directly to the ICU on the weekend rather than being admitted first to the general medicine floor. This hypothesis requires further study.
Our study had important limitations. It was a retrospective study from a single academic hospital. The sample size lacked sufficient power to detect differences in the low frequency of certain outcomes such as poor outcomes within 24 hours of admission (2% vs. 1%), and also for more frequent outcomes such as 30 day readmission; it is possible that with a larger sample there would have been statistically significant differences. Further, we recognize that the Charlson Comorbidity Index, which was developed to predict 1‐year mortality for medicine service patients, does not adjust for severity of illness at presentation, particularly for outcomes such as readmission. If patients admitted at night and during the weekend were less acutely ill despite having similar comorbidities and MDCs at admission, true associations between time of admission and worse outcomes could have been masked. Furthermore, the multivariable modeling explained very little of the variance in patient outcomes such that significant unmeasured confounding may still be present, and consequently our results cannot be interpreted in a causal way. Data was collected from electronic records, so it is possible that some adverse events were not recorded. However, it seems unlikely that major events such as death and transfer to an ICU would have been missed.
Several aspects of the study strengthen our confidence in the findings, including a large sample size, relevance of the outcomes, the adjustment for confounders, and an assessment for robustness of the conclusions based on restricting the definition of night and also testing for interaction between night and weekend admission. Our patient demographics and insurance mix resemble that of other academic hospitals,10 and perhaps our results may be generalizable to these settings, if not to non‐urban or community hospitals. Furthermore, the Charlson Comorbidity Index was associated with all 5 of the modeled outcomes we chose for our study, reaffirming their utility in assessing the quality of hospital care. Future directions for investigation may include examining the association of night admission with hospitalization‐relevant outcomes in nonacademic, nonurban settings, and examining whether the lack of association between night and weekend admission and worse outcomes persists with adjustment for initial severity of illness.
In summary, at a large, well‐staffed urban academic hospital, day or time of admission were not associated with worse hospitalization‐relevant outcomes. The use of nocturnists and night float teams for night admissions and continuity across weekends appears to be a safe approach to handling the increased volume of patients admitted at night, and a viable alternative to overnight call in the era of work hour restrictions.
- Three‐year results of mandated work hour restrictions: attending and resident perspectives and effects in a community hospital.Am Surg.2008;74(6):542–546; discussion 546–547. , , , et al.
- Handoffs causing patient harm: a survey of medical and surgical house staff.Jt Comm J Qual Patient Saf.2008;34(10):563–570. , , , et al.
- Does housestaff discontinuity of care increase the risk for preventable adverse events?Ann Intern Med.1994;121(11):866–872. , , , , .
- Mortality among patients admitted to hospitals on weekends as compared with weekdays.N Engl J Med.2001;345(9):663–668. , .
- Survival from in‐hospital cardiac arrest during nights and weekends.JAMA.2008;299(7):785–792. , , , et al.
- Continuity of care and intensive care unit use at the end of life.Arch Intern Med.2009;169(1):81–86. , , , .
- Continuity of outpatient and inpatient care by primary care physicians for hospitalized older adults.JAMA.2009;301(16):1671–1680. , , , , , .
- Why predictive indexes perform less well in validation studies: is it magic or methods?Arch Intern Med.1987;147:2155–2161. , , , .
- Adapting a clinical comorbidity index for use with ICD‐9‐CM administrative databases.J Clin Epidemiol.1992;45(6):613–619. , , .
- Implementation of a physician assistant/hospitalist service in an academic medical center: impact on efficiency and patient outcomes.J Hosp Med.2008;3(5):361–368. , , , et al.
- Use of an admission early warning score to predict patient morbidity and mortality and treatment success.Emerg Med J.2008;25(12):803–806. , , , et al.
- The impact of weekends on outcome for emergency patients.Clin Med.2005;5(6):621–625. , , .
- Off hour admission to an intensivist‐led ICU is not associated with increased mortality.Crit Care.2009;13(3):R84. , , , , , .
- Three‐year results of mandated work hour restrictions: attending and resident perspectives and effects in a community hospital.Am Surg.2008;74(6):542–546; discussion 546–547. , , , et al.
- Handoffs causing patient harm: a survey of medical and surgical house staff.Jt Comm J Qual Patient Saf.2008;34(10):563–570. , , , et al.
- Does housestaff discontinuity of care increase the risk for preventable adverse events?Ann Intern Med.1994;121(11):866–872. , , , , .
- Mortality among patients admitted to hospitals on weekends as compared with weekdays.N Engl J Med.2001;345(9):663–668. , .
- Survival from in‐hospital cardiac arrest during nights and weekends.JAMA.2008;299(7):785–792. , , , et al.
- Continuity of care and intensive care unit use at the end of life.Arch Intern Med.2009;169(1):81–86. , , , .
- Continuity of outpatient and inpatient care by primary care physicians for hospitalized older adults.JAMA.2009;301(16):1671–1680. , , , , , .
- Why predictive indexes perform less well in validation studies: is it magic or methods?Arch Intern Med.1987;147:2155–2161. , , , .
- Adapting a clinical comorbidity index for use with ICD‐9‐CM administrative databases.J Clin Epidemiol.1992;45(6):613–619. , , .
- Implementation of a physician assistant/hospitalist service in an academic medical center: impact on efficiency and patient outcomes.J Hosp Med.2008;3(5):361–368. , , , et al.
- Use of an admission early warning score to predict patient morbidity and mortality and treatment success.Emerg Med J.2008;25(12):803–806. , , , et al.
- The impact of weekends on outcome for emergency patients.Clin Med.2005;5(6):621–625. , , .
- Off hour admission to an intensivist‐led ICU is not associated with increased mortality.Crit Care.2009;13(3):R84. , , , , , .
Copyright © 2010 Society of Hospital Medicine