User login
Caring About Prognosis
Prognostication continues to be a challenge to the clinician despite over 100 prognostic indices that have been developed during the past few decades to inform clinical practice and medical decision making.[1] Physicians are not accurate in prognostication of patients' risk of death and tend to overestimate survival.[2, 3] In addition, many physicians do not feel comfortable offering a prognosis to patients, despite patients' wishes to be informed.[4, 5] Regardless of the prevalence in the literature and value in improving physicians' prognostic accuracy, prognostic indices of survival are not regularly utilized in the hospital setting. Prognostic tools available for providers are often complicated and may require data about patients that are not readily available.[6, 7, 8] Prognostic indices may be too specific to a patient population, too difficult to remember, or too time consuming to use. A simple, rapid, and practical prognostic index is important in the hospital setting to assist in identifying patients at high risk of death so that primary palliative interventions can be incorporated into the plan of care early in the hospital stay. Patient and family education, advance care planning, formulating the plan of care based on patientfamily goals, and improved resource utilization could be better executed by more accurate risk of death prediction on hospital admission.
The CARING criteria are the only prognostic index to our knowledge that evaluates a patient's risk of death in the next year, with information readily available at the time of hospital admission (Table 1).[9] The CARING criteria are a unique prognostic tool: (1) CARING is a mnemonic acronym, making it more user friendly to the clinician. (2) The 5 prognostic indicators are readily available from the patient's chart on admission; gathering further data by patient or caretaker interviews or by obtaining laboratory data is not needed. (3) The timing for application of the tool on admission to the hospital is an ideal opportunity to intervene and introduce palliative interventions early on the hospital stay. The CARING criteria were developed and validated in a Veteran's Administration hospital setting by Fischer et al.[9] We sought to validate the CARING criteria in a broader patient populationmedical and surgical patients from a tertiary referral university hospital setting and a safety‐net hospital setting.
METHODS
Study Design
This study was a retrospective observational cohort study. The study was approved by the Colorado Multiple Institutional Review Board and the University of Colorado Hospital Research Review Committee.
Study Purpose
To validate the CARING criteria in a tertiary referral university hospital (University of Colorado Hospital [UCH]) and safety‐net hospital (Denver Health and Hospitals [DHH]) setting using similar methodology to that employed by the original CARING criteria study.[9]
Study Setting/Population
All adults (18 years of age) admitted as inpatients to the medical and surgical services of internal medicine, hospitalist, pulmonary, cardiology, hematology/oncology, hepatology, surgery, intensive care unit, and intermediary care unit at UCH and DHH during the study period of July 2005 through August 2005. The only exclusion criteria were those patients who were prisoners or pregnant. Administrative admission data from July 2005 to August 2005 were used to identify names of all persons admitted to the medicine and surgical services of the study hospitals during the specified time period.
The 2 study hospitals, UCH and DHH, provide a range of patients who vary in ethnicity, socioeconomic status, and medical illness. This variability allows for greater generalizability of the results. Both hospitals are affiliated with the University of Colorado School of Medicine internal medicine residency training program and are located in Denver, Colorado.
At the time of the study, UCH was a licensed 550‐bed tertiary referral, academic hospital serving the Denver metropolitan area and the Rocky Mountain region as a specialty care and referral center. DHH was a 398‐bed, academic, safety‐net hospital serving primarily the Denver metropolitan area. DHH provides 42% of the care for the uninsured in Denver and 26% of the uninsured care for the state of Colorado.
Measures
The CARING criteria were developed and validated in a Veteran's Administration (VA) hospital setting by Fischer et al.[9] The purpose of the CARING criteria is to identify patients, at the time of hospital admission, who are at higher risk of death in the following year. The prognostic index uses 5 predictors that can be abstracted from the chart at time of admission. The CARING criteria were developed a priori, and patients were evaluated using only the medical data available at the time of admission. The criteria include items that are already part of the routine physician admission notes and do not require additional data collection or assessments. The criteria include: C=primary diagnosis of cancer, A=2 admissions to the hospital for a chronic illness within the last year; R=resident in a nursing home; I=intensive care unit (ICU) admission with multiorgan failure (MOF), NG=noncancer hospice guidelines (meeting 2 of the National Hospice and Palliative Care Organization's [NHPCO] guidelines).
Patients were identified using name, date of birth, social security number, address, and phone number. This identifying information was then used for tracing death records 1 year after hospital admission.
Mortality at 1 year following the index hospitalization was the primary end point. To minimize missing data and the number of subjects lost to follow‐up, 3 determinants of mortality were used. First, electronic medical records of the 2 participating hospitals and their outpatient clinics were reviewed to determine if a follow‐up appointment had occurred past the study's end point of 1 year (August 2006). For those without a confirmed follow‐up visit, death records from the Colorado Department of Public Health and Vital Records were obtained. For those patients residing outside of Colorado or whose mortality status was still unclear, the National Death Index was accessed.
Medical Record Review
Medical records for all study participants were reviewed by J.Y. (UCH) and B.C. (DHH). Data collection was completed using direct data entry into a Microsoft Access (Microsoft Corp., Redmond, WA) database utilizing a data entry form linked with the database table. This form utilized skip patterns and input masks to ensure quality of data entry and minimize missing or invalid data. Inter‐rater reliability was assessed by an independent rereview (S.F.) of 5% of the total charts. Demographic variables were collected using hospital administrative data. These included personal identifiers of the participants for purposes of mortality follow‐up. Clinical data including the 5 CARING variables and additional descriptive variables were abstracted from the paper hospital chart and the electronic record of the chart (together these constitute the medical record).
Death Follow‐up
A search of Colorado death records was conducted in February 2011 for all subjects. Death records were used to determine mortality and time to death from the index hospitalization. The National Death Index was then searched for any subjects without or record of death in Colorado.
Analysis
All analyses were conducted using the statistical application software SAS for Windows version 9.3 (SAS Institute, Cary, NC). Simple frequencies and means ( standard deviation) were used to describe the baseline characteristics. Multiple logistic regression models were used to model 1‐year mortality. The models were fitted using all of the CARING variables and age. As the aim of the study was to validate the CARING criteria, the variables for the models were selected a priori based on the original index. Two hospital cohorts (DHH and UCH) were modeled separately and as a combined sample. Kaplan‐Meier survival analysis was conducted to compare those subjects who met 1 of the CARING criteria with those who did not through the entire period of mortality follow‐up (20052011). Finally, using the probabilities from the logistic regression models, we again developed a scoring rule appropriate for a non‐VA setting to allow clinicians to easily identify patient risk for 1‐year mortality at the time of hospital admission.
RESULTS
There were a total of 1064 patients admitted to the medical and surgical services during the study period568 patients at DHH and 496 patients at UCH. Sample characteristics of each individual hospital cohort and the entire combined study cohort are detailed in Table 2. Overall, slightly over half the population were male, with a mean age of 50 years, and the ethnic breakdown roughly reflects the population in Denver. A total of 36.5% (n=388) of the study population met 1 of the CARING criteria, and 12.6% (n=134 among 1063 excluding 1 without an admit date) died within 1 year of the index hospitalization. These were younger and healthier patients compared to the VA sample used in developing the CARING criteria.
| |
Renal | Dementia |
Stop/decline dialysis | Unable to ambulate independently |
Not candidate for transplant | Urinary or fecal incontinence |
Urine output < 40cc/24 hours | Unable to speak with more than single words |
Creatinine > 8.0 (>6.0 for diabetics) | Unable to bathe independently |
Creatinine clearance 10cc/min | Unable to dress independently |
Uremia | Co‐morbid conditions: |
Persistent serum K + > 7.0 | Aspiration pneumonia |
Co‐morbid conditions: | Pyelonephritis |
Cancer CHF | Decubitus ulcer |
Chronic lung disease AIDS/HIV | Difficulty swallowing or refusal to eat |
Sepsis Cirrhosis | |
Cardiac | Pulmonary |
Ejection fraction < 20% | Dyspnea at rest |
Symptomatic with diuretics and vasodilators | FEV1 < 30% |
Not candidate for transplant | Frequent ER or hospital admits for pulmonary infections or respiratory distress |
History of cardiac arrest | Cor pulmonale or right heart failure |
History of syncope | 02 sat < 88% on 02 |
Systolic BP < 120mmHG | PC02 > 50 |
CVA cardiac origin | Resting tachycardia > 100/min |
Co‐morbid conditions as listed in Renal | Co‐morbid conditions as listed in Renal |
Liver | Stroke/CVA |
End stage cirrhosis | Coma at onset |
Not candidate for transplant | Coma >3 days |
Protime > 5sec and albumin <2.5 | Limb paralysis |
Ascites unresponsive to treatment | Urinary/fecal incontinence |
Hepatorenal syndrome | Impaired sitting balance |
Hepatic encephalopathy | Karnofsky < 50% |
Spontaneous bacterial peritonitis | Recurrent aspiration |
Recurrent variceal bleed | Age > 70 |
Co‐morbid conditions as listed in Renal | Co‐morbid conditions as listed in Renal |
HIV/AIDS | Neuromuscular |
Persistent decline in function | Diminished respiratory function |
Chronic diarrhea 1 year | Chosen not to receive BiPAP/vent |
Decision to stop treatment | Difficulty swallowing |
CNS lymphoma | Diminished functional status |
MAC‐untreated | Incontinence |
Systemic lymphoma | Co‐morbid conditions as listed in Renal |
Dilated cardiomyopathy | |
CD4 < 25 with disease progression | |
Viral load > 100,000 |
Safety‐Net Hospital Cohort, N=568 | Academic Center Cohort, N=496 | Study Cohort,N=1064 | Original CARING Cohort, N=8739 | |
---|---|---|---|---|
| ||||
Mean age ( SD), y | 47.8 (16.5) | 54.4 (17.5) | 50.9 (17.3) | 63 (13) |
Male gender | 59.5% (338) | 50.1% (248) | 55.1% (586) | 98% (856) |
Ethnicity | ||||
African American | 14.1% (80) | 13.5% (65) | 13.8% (145) | 13% (114) |
Asian | 0.4% (2) | 1.5% (7) | 0.9% (9) | Not reported |
Caucasian | 41.7% (237) | 66.3% (318) | 53.0 % (555) | 69% (602) |
Latino | 41.9% (238) | 9.6% (46) | 27.1% (284) | 8% (70) |
Native American | 0.5% (3) | 0.4% (2) | 0.5% (5) | Not reported |
Other | 0.5% (3) | 0.6% (3) | 0.6% (6) | 10% (87) |
Unknown | 0.9% (5) | 8.1% (39) | 4.2% (44) | Not reported |
CARING criteria | ||||
Cancer | 6.2% (35) | 19.4% (96) | 12.3% (131) | 23% (201) |
Admissions to the hospital 2 in past year | 13.6% (77) | 42.7% (212) | 27.2% (289) | 36% (314) |
Resident in a nursing home | 1.8% (10) | 3.4% (17) | 2.5% (27) | 3% (26) |
ICU with MOF | 3.7% (21) | 1.2% (6) | 2.5% (27) | 2% (17) |
NHPCO (2) noncancer guidelines | 1.6% (9) | 5.9% (29) | 3.6% (38) | 8% (70) |
Reliability testing demonstrated excellent inter‐rater reliability. Kappa for each criterion is as follows: (1) primary diagnosis of cancer=1.0, (2) 2 admissions to the hospital in the past year=0.91, (3) resident in a nursing home=1.0, (4) ICU admission with MOF=1.0, and (5) 2 noncancer hospice guidelines=0.78.
This study aimed to validate the CARING criteria9; therefore, all original individual CARING criterion were included in the validation logistic regression models. The 1 exception to this was in the university hospital study cohort, where the ICU criterion was excluded from the model due to small sample size and quasiseparation in the model. The model results are presented in Table 3 for the individual hospitals and combined study cohort.
Safety Net Hospital Cohort, C Index=0.76 | Academic Center Cohort, C Index=0.76 | Combined Hospital Cohort, C Index=0.79 | ||||
---|---|---|---|---|---|---|
Estimate | Odds Ratio (95% CI) | Estimate | Odds Ratio (95% CI) | Estimate | Odds Ratio (95% CI) | |
| ||||||
Cancer | 1.92 | 6.85 (2.83‐16.59)a | 1.85 | 6.36 (3.54‐11.41)a | 1.98 | 7.23 (4.45‐11.75)a |
Admissions to the hospital 2 in past year | 0.55 | 1.74 (0.76‐3.97) | 0.14 | 0.87 (0.51‐1.49) | 0.20 | 1.22 (0.78‐1.91) |
Resident in a nursing home | 0.49 | 0.61 (0.06‐6.56) | 0.27 | 1.31 (0.37‐4.66) | 0.09 | 1.09 (0.36‐3.32) |
ICU with MOF | 1.85 | 6.34 (2.0219.90)a | 1.94 | 6.97 (2.75‐17.68)a | ||
NHPCO (2) noncancer guidelines | 3.04 | 20.86 (4.25102.32)a | 2.62 | 13.73 (5.86‐32.15)a | 2.74 | 15.55 (7.2833.23)a |
Ageb | 0.38 | 1.46 (1.05‐2.03)a | 0.45 | 1.56 (1.23‐1.98)a | 0.47 | 1.60 (1.32‐1.93)a |
In the safety‐net hospital, admission to the hospital with a primary diagnosis related to cancer, 2 noncancer hospice guidelines, ICU admission with MOF, and age by category all were significant predictors of 1‐year mortality. In the university hospital cohort, primary diagnosis of cancer, 2 noncancer hospice guidelines, and age by category were predictive of 1‐year mortality. Finally, in the entire study cohort, primary diagnosis of cancer, ICU with MOF, 2 noncancer hospice guidelines, and age were all predictive of 1‐year mortality. Parameter estimates were similar in 3 of the criteria compared to the VA setting. Differences in patient characteristics may have caused the differences in the estimates. Gender was additionally tested but not significant in any model. One‐year survival was significantly lower for those who met 1 of the CARING criteria versus those who did not (Figure 1).
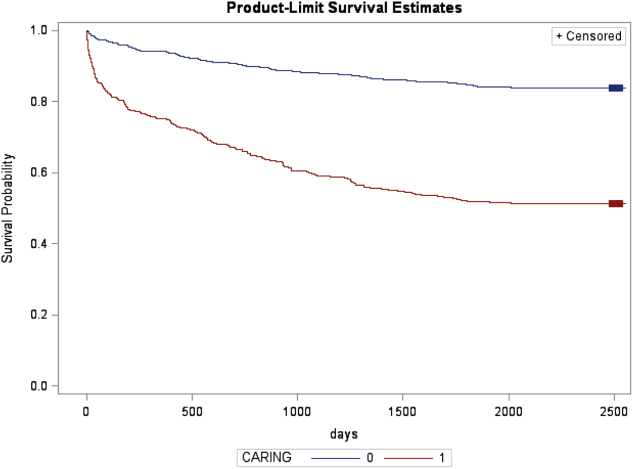
Based on the framework from the original CARING criteria analysis, a scoring rule was developed using the regression results of this validation cohort. To predict a high probability of 1‐year mortality, sensitivity was set to 58% and specificity was set at 86% (error rate=17%). Medium to high probability was set with a sensitivity of 73% and specificity of 72% (error rate=28%). The coefficients from the regression model of the entire study cohort were converted to scores for each of the CARING criteria. The scores are as follows: 0.5 points for admission from a nursing home, 1 point for 2 hospital admissions in the past year for a chronic illness, 10 points for primary diagnosis of cancer, 10 points for ICU admission with MOF, and 14 points for 2 noncancer hospice guidelines. For every age category increase, 2 points are assigned so that 0 points for age <55 years, 2 points for ages 56 to 65 years, 4 points for ages 66 to 75 years, and 6 points for >75 years. Points for individual risk factors were proportional to s (ie, log odds) in the logistic regression model for death at 1 year. Although no linear transformation exists between s and probabilities (of death at 1 year), the aggregated points for combinations of risk factors shown in Table 4 follow the probabilities in an approximately linear fashion, so that different degrees of risk of death can be represented contiguously (as highlighted by differently shaded regions in the scoring matrix) (Table 4). The scoring matrix allows for quick identification for patients at high risk for 1‐year mortality. In this non‐VA setting with healthier patients, low risk is defined at a lower probability threshold (0.1) compared to the VA setting (0.175).
CARING Criteria Components | |||||||
---|---|---|---|---|---|---|---|
None | Resident in a Nursing Home | Admitted to the Hospital 2 Times in the Past Year | Resident in a Nursing Home Admitted to the Hospital 2 Times in the Past Year | Primary Diagnosis of Cancer | ICU Admission With MOF | Noncancer Hospice Guidelines | |
| |||||||
Age | |||||||
55 years | 0 | 0.5 | 1 | 1.5 | 10 | ||
5565 years | 2 | 2.5 | 3 | 3.5 | 10 | ||
6675 years | 4 | 4.5 | 5 | 5.5 | 10 | ||
>75 years | 6 | 6.5 | 7 | 7.5 | 10 | ||
Risk | |||||||
Low | 3.5 | Probability<0.1 | |||||
Medium | 46.5 | 0.1probability <0.175 | |||||
High | 7 | Probability0.175 |
DISCUSSION
The CARING criteria are a practical prognostic tool that can be easily and rapidly applied to patients admitted to the hospital to estimate risk of death in 1 year, with the goal of identifying patients who may benefit most from incorporating palliative interventions into their plan of care. This study validated the CARING criteria in a tertiary referral university hospital and safety‐net hospital setting, demonstrating applicability in a much broader population than the VA hospital of the original CARING criteria study. The population studied represented a younger population by over 10 years, a more equitable proportion of males to females, a broader ethnic diversity, and lower 1‐year deaths rates than the original study. Despite the broader representation of the population, the significance of each of the individual CARING criterion was maintained except for 2 hospital admissions in the past year for a chronic illness (admission from a nursing home did not meet significance in either study as a sole criterion). As with the original study, meeting 2 of the NHPCO noncancer hospice guidelines demonstrated the highest risk of 1‐year mortality following index hospitalization, followed by primary diagnosis of cancer and ICU admission with MOF. Advancing age, also similar to the original study, conferred increased risk across the criterion.
Hospitalists could be an effective target for utilizing the CARING criteria because they are frequently the first‐line providers in the hospital setting. With the national shortage of palliative care specialists, hospitalists need to be able to identify when a patient has a limited life expectancy so they will be better equipped to make clinical decisions that are aligned with their patients' values, preferences, and goals of care. With the realization that not addressing advance care planning and patient goals of care may be considered medical errors, primary palliative care skills become alarmingly more important as priorities for hospitalists to obtain and feel comfortable using in daily practice.
The CARING criteria are directly applicable to patients who are seen by hospitalists. Other prognostic indices have focused on select patient populations, such as the elderly,[10, 11, 12] require collection of data that are not readily available on admission or would not otherwise be obtained,[10, 13] or apply to patients post‐hospital discharge, thereby missing the opportunity to make an impact earlier in the disease trajectory and incorporate palliative care into the hospital plan of care when key discussions about goals of care and preferences should be encouraged.
Additionally, the CARING criteria could easily be incorporated as a trigger for palliative care consults on hospital admission. Palliative care consults tend to happen late in a hospital stay, limiting the effectiveness of the palliative care team. A trigger system for hospitalists and other primary providers on hospital admission would lend to more effective timing of palliative measures being incorporated into the plan of care. Palliative care consults would not only be initiated earlier, but could be targeted for the more complex and sick patients with the highest risk of death in the next year.
In the time‐pressured environment, the presence of any 1 of the CARING criteria can act as a trigger to begin incorporating primary palliative care measures into the plan of care. The admitting hospitalist provider (ie, physician, nurse practitioner, physician assistant) could access the CARING criteria through an electronic health record prompt when admitting patients. When a more detailed assessment of mortality risk is helpful, the hospitalist can use the scoring matrix, which combines age with the individual criterion to calculate patients at medium or high risk of death within 1 year. Limited resources can then be directed to the patients with the greatest need. Patients with a focused care need, such as advance care planning or hospice referral, can be directed to the social worker or case manager. More complicated patients may be referred to a specialty palliative care team.
Several limitations to this study are recognized, including the small sample size of patients meeting criterion for ICU with MOF in the academic center study cohort. The patient data were collected during a transition time when the university hospital moved to a new campus, resulting in an ICU at each campus that housed patients with differing levels of illness severity, which may have contributed to the lower acuity ICU patient observed. Although we advocate the simplicity of the CARING criteria, the NHPCO noncancer hospice guidelines are more complicated, as they incorporates 8 broad categories of chronic illness. The hospice guidelines may not be general knowledge to the hospitalist or other primary providers. ePrognosis (
CONCLUSION
The CARING criteria are a simple, practical prognostic tool predictive of death within 1 year that has been validated in a broad population of hospitalized patients. The criteria hold up in a younger, healthier population that is more diverse by age, gender, and ethnicity than the VA population. With ready access to critical prognostic information on hospital admission, clinicians will be better informed to make decisions that are aligned with their patients' values, preferences, and goals of care.
Disclosure
Nothing to report.
- Predicting death: an empirical evaluation of predictive tools for mortality. Arch Intern Med. 2011;171:1721–1726. , , .
- Extent and determinants of error in physicians' prognoses in terminally ill patients: prospective cohort study. West J Med. 2000;172:310–313. , .
- A systematic review of physicians' survival predictions in terminally ill cancer patients. BMJ. 2003;327:195–198. , , , et al.
- Attitude and self‐reported practice regarding prognostication in a national sample of internists. Arch Intern Med. 1998;158:2389–2395. , .
- Discussing prognosis: balancing hope and realism. Cancer J. 2010;16:461–466. , , , et al.
- Acute Physiology and Chronic Health Evaluation (APACHE) IV: hospital mortality assessment for today's critically ill patients. Crit Care Med. 2006;34:1297–1310. , , , .
- SAPS 3 admission score: an external validation in a general intensive care population. Intensive Care Med. 2008;34:1873–1877. , , , , .
- Prospective validation of the intensive care unit admission Mortality Probability Model (MPM0‐III). Crit Care Med. 2009;37:1619–1623. , , , , , .
- A practical tool to identify patients who may benefit from a palliative approach: the CARING criteria. J Pain Symptom Manage. 2006;31:285–292. , , , , , .
- Prediction of survival for older hospitalized patients: the HELP survival model. J Am Geriatr Soc. 2000;48:S16–S24. , , , et al.
- Development and validation of a multidimensional prognostic index for one‐year mortality from comprehensive geriatric assessment in hospitalized older patients. Rejuvenation Res. 2008;11:151–161. , , , et al.
- Burden of illness score for elderly persons: risk adjustment incorporating the cumulative impact of diseases, physiologic abnormalities, and functional impairments. Med Care. 2003;41:70–83. , , , et al.
- The SUPPORT prognostic model. Objective estimates of survival for seriously ill hospitalized adults. Study to understand prognoses and preferences for outcomes and risks of treatments. Ann Intern Med. 1995;122:191–203. , , , et al.
Prognostication continues to be a challenge to the clinician despite over 100 prognostic indices that have been developed during the past few decades to inform clinical practice and medical decision making.[1] Physicians are not accurate in prognostication of patients' risk of death and tend to overestimate survival.[2, 3] In addition, many physicians do not feel comfortable offering a prognosis to patients, despite patients' wishes to be informed.[4, 5] Regardless of the prevalence in the literature and value in improving physicians' prognostic accuracy, prognostic indices of survival are not regularly utilized in the hospital setting. Prognostic tools available for providers are often complicated and may require data about patients that are not readily available.[6, 7, 8] Prognostic indices may be too specific to a patient population, too difficult to remember, or too time consuming to use. A simple, rapid, and practical prognostic index is important in the hospital setting to assist in identifying patients at high risk of death so that primary palliative interventions can be incorporated into the plan of care early in the hospital stay. Patient and family education, advance care planning, formulating the plan of care based on patientfamily goals, and improved resource utilization could be better executed by more accurate risk of death prediction on hospital admission.
The CARING criteria are the only prognostic index to our knowledge that evaluates a patient's risk of death in the next year, with information readily available at the time of hospital admission (Table 1).[9] The CARING criteria are a unique prognostic tool: (1) CARING is a mnemonic acronym, making it more user friendly to the clinician. (2) The 5 prognostic indicators are readily available from the patient's chart on admission; gathering further data by patient or caretaker interviews or by obtaining laboratory data is not needed. (3) The timing for application of the tool on admission to the hospital is an ideal opportunity to intervene and introduce palliative interventions early on the hospital stay. The CARING criteria were developed and validated in a Veteran's Administration hospital setting by Fischer et al.[9] We sought to validate the CARING criteria in a broader patient populationmedical and surgical patients from a tertiary referral university hospital setting and a safety‐net hospital setting.
METHODS
Study Design
This study was a retrospective observational cohort study. The study was approved by the Colorado Multiple Institutional Review Board and the University of Colorado Hospital Research Review Committee.
Study Purpose
To validate the CARING criteria in a tertiary referral university hospital (University of Colorado Hospital [UCH]) and safety‐net hospital (Denver Health and Hospitals [DHH]) setting using similar methodology to that employed by the original CARING criteria study.[9]
Study Setting/Population
All adults (18 years of age) admitted as inpatients to the medical and surgical services of internal medicine, hospitalist, pulmonary, cardiology, hematology/oncology, hepatology, surgery, intensive care unit, and intermediary care unit at UCH and DHH during the study period of July 2005 through August 2005. The only exclusion criteria were those patients who were prisoners or pregnant. Administrative admission data from July 2005 to August 2005 were used to identify names of all persons admitted to the medicine and surgical services of the study hospitals during the specified time period.
The 2 study hospitals, UCH and DHH, provide a range of patients who vary in ethnicity, socioeconomic status, and medical illness. This variability allows for greater generalizability of the results. Both hospitals are affiliated with the University of Colorado School of Medicine internal medicine residency training program and are located in Denver, Colorado.
At the time of the study, UCH was a licensed 550‐bed tertiary referral, academic hospital serving the Denver metropolitan area and the Rocky Mountain region as a specialty care and referral center. DHH was a 398‐bed, academic, safety‐net hospital serving primarily the Denver metropolitan area. DHH provides 42% of the care for the uninsured in Denver and 26% of the uninsured care for the state of Colorado.
Measures
The CARING criteria were developed and validated in a Veteran's Administration (VA) hospital setting by Fischer et al.[9] The purpose of the CARING criteria is to identify patients, at the time of hospital admission, who are at higher risk of death in the following year. The prognostic index uses 5 predictors that can be abstracted from the chart at time of admission. The CARING criteria were developed a priori, and patients were evaluated using only the medical data available at the time of admission. The criteria include items that are already part of the routine physician admission notes and do not require additional data collection or assessments. The criteria include: C=primary diagnosis of cancer, A=2 admissions to the hospital for a chronic illness within the last year; R=resident in a nursing home; I=intensive care unit (ICU) admission with multiorgan failure (MOF), NG=noncancer hospice guidelines (meeting 2 of the National Hospice and Palliative Care Organization's [NHPCO] guidelines).
Patients were identified using name, date of birth, social security number, address, and phone number. This identifying information was then used for tracing death records 1 year after hospital admission.
Mortality at 1 year following the index hospitalization was the primary end point. To minimize missing data and the number of subjects lost to follow‐up, 3 determinants of mortality were used. First, electronic medical records of the 2 participating hospitals and their outpatient clinics were reviewed to determine if a follow‐up appointment had occurred past the study's end point of 1 year (August 2006). For those without a confirmed follow‐up visit, death records from the Colorado Department of Public Health and Vital Records were obtained. For those patients residing outside of Colorado or whose mortality status was still unclear, the National Death Index was accessed.
Medical Record Review
Medical records for all study participants were reviewed by J.Y. (UCH) and B.C. (DHH). Data collection was completed using direct data entry into a Microsoft Access (Microsoft Corp., Redmond, WA) database utilizing a data entry form linked with the database table. This form utilized skip patterns and input masks to ensure quality of data entry and minimize missing or invalid data. Inter‐rater reliability was assessed by an independent rereview (S.F.) of 5% of the total charts. Demographic variables were collected using hospital administrative data. These included personal identifiers of the participants for purposes of mortality follow‐up. Clinical data including the 5 CARING variables and additional descriptive variables were abstracted from the paper hospital chart and the electronic record of the chart (together these constitute the medical record).
Death Follow‐up
A search of Colorado death records was conducted in February 2011 for all subjects. Death records were used to determine mortality and time to death from the index hospitalization. The National Death Index was then searched for any subjects without or record of death in Colorado.
Analysis
All analyses were conducted using the statistical application software SAS for Windows version 9.3 (SAS Institute, Cary, NC). Simple frequencies and means ( standard deviation) were used to describe the baseline characteristics. Multiple logistic regression models were used to model 1‐year mortality. The models were fitted using all of the CARING variables and age. As the aim of the study was to validate the CARING criteria, the variables for the models were selected a priori based on the original index. Two hospital cohorts (DHH and UCH) were modeled separately and as a combined sample. Kaplan‐Meier survival analysis was conducted to compare those subjects who met 1 of the CARING criteria with those who did not through the entire period of mortality follow‐up (20052011). Finally, using the probabilities from the logistic regression models, we again developed a scoring rule appropriate for a non‐VA setting to allow clinicians to easily identify patient risk for 1‐year mortality at the time of hospital admission.
RESULTS
There were a total of 1064 patients admitted to the medical and surgical services during the study period568 patients at DHH and 496 patients at UCH. Sample characteristics of each individual hospital cohort and the entire combined study cohort are detailed in Table 2. Overall, slightly over half the population were male, with a mean age of 50 years, and the ethnic breakdown roughly reflects the population in Denver. A total of 36.5% (n=388) of the study population met 1 of the CARING criteria, and 12.6% (n=134 among 1063 excluding 1 without an admit date) died within 1 year of the index hospitalization. These were younger and healthier patients compared to the VA sample used in developing the CARING criteria.
| |
Renal | Dementia |
Stop/decline dialysis | Unable to ambulate independently |
Not candidate for transplant | Urinary or fecal incontinence |
Urine output < 40cc/24 hours | Unable to speak with more than single words |
Creatinine > 8.0 (>6.0 for diabetics) | Unable to bathe independently |
Creatinine clearance 10cc/min | Unable to dress independently |
Uremia | Co‐morbid conditions: |
Persistent serum K + > 7.0 | Aspiration pneumonia |
Co‐morbid conditions: | Pyelonephritis |
Cancer CHF | Decubitus ulcer |
Chronic lung disease AIDS/HIV | Difficulty swallowing or refusal to eat |
Sepsis Cirrhosis | |
Cardiac | Pulmonary |
Ejection fraction < 20% | Dyspnea at rest |
Symptomatic with diuretics and vasodilators | FEV1 < 30% |
Not candidate for transplant | Frequent ER or hospital admits for pulmonary infections or respiratory distress |
History of cardiac arrest | Cor pulmonale or right heart failure |
History of syncope | 02 sat < 88% on 02 |
Systolic BP < 120mmHG | PC02 > 50 |
CVA cardiac origin | Resting tachycardia > 100/min |
Co‐morbid conditions as listed in Renal | Co‐morbid conditions as listed in Renal |
Liver | Stroke/CVA |
End stage cirrhosis | Coma at onset |
Not candidate for transplant | Coma >3 days |
Protime > 5sec and albumin <2.5 | Limb paralysis |
Ascites unresponsive to treatment | Urinary/fecal incontinence |
Hepatorenal syndrome | Impaired sitting balance |
Hepatic encephalopathy | Karnofsky < 50% |
Spontaneous bacterial peritonitis | Recurrent aspiration |
Recurrent variceal bleed | Age > 70 |
Co‐morbid conditions as listed in Renal | Co‐morbid conditions as listed in Renal |
HIV/AIDS | Neuromuscular |
Persistent decline in function | Diminished respiratory function |
Chronic diarrhea 1 year | Chosen not to receive BiPAP/vent |
Decision to stop treatment | Difficulty swallowing |
CNS lymphoma | Diminished functional status |
MAC‐untreated | Incontinence |
Systemic lymphoma | Co‐morbid conditions as listed in Renal |
Dilated cardiomyopathy | |
CD4 < 25 with disease progression | |
Viral load > 100,000 |
Safety‐Net Hospital Cohort, N=568 | Academic Center Cohort, N=496 | Study Cohort,N=1064 | Original CARING Cohort, N=8739 | |
---|---|---|---|---|
| ||||
Mean age ( SD), y | 47.8 (16.5) | 54.4 (17.5) | 50.9 (17.3) | 63 (13) |
Male gender | 59.5% (338) | 50.1% (248) | 55.1% (586) | 98% (856) |
Ethnicity | ||||
African American | 14.1% (80) | 13.5% (65) | 13.8% (145) | 13% (114) |
Asian | 0.4% (2) | 1.5% (7) | 0.9% (9) | Not reported |
Caucasian | 41.7% (237) | 66.3% (318) | 53.0 % (555) | 69% (602) |
Latino | 41.9% (238) | 9.6% (46) | 27.1% (284) | 8% (70) |
Native American | 0.5% (3) | 0.4% (2) | 0.5% (5) | Not reported |
Other | 0.5% (3) | 0.6% (3) | 0.6% (6) | 10% (87) |
Unknown | 0.9% (5) | 8.1% (39) | 4.2% (44) | Not reported |
CARING criteria | ||||
Cancer | 6.2% (35) | 19.4% (96) | 12.3% (131) | 23% (201) |
Admissions to the hospital 2 in past year | 13.6% (77) | 42.7% (212) | 27.2% (289) | 36% (314) |
Resident in a nursing home | 1.8% (10) | 3.4% (17) | 2.5% (27) | 3% (26) |
ICU with MOF | 3.7% (21) | 1.2% (6) | 2.5% (27) | 2% (17) |
NHPCO (2) noncancer guidelines | 1.6% (9) | 5.9% (29) | 3.6% (38) | 8% (70) |
Reliability testing demonstrated excellent inter‐rater reliability. Kappa for each criterion is as follows: (1) primary diagnosis of cancer=1.0, (2) 2 admissions to the hospital in the past year=0.91, (3) resident in a nursing home=1.0, (4) ICU admission with MOF=1.0, and (5) 2 noncancer hospice guidelines=0.78.
This study aimed to validate the CARING criteria9; therefore, all original individual CARING criterion were included in the validation logistic regression models. The 1 exception to this was in the university hospital study cohort, where the ICU criterion was excluded from the model due to small sample size and quasiseparation in the model. The model results are presented in Table 3 for the individual hospitals and combined study cohort.
Safety Net Hospital Cohort, C Index=0.76 | Academic Center Cohort, C Index=0.76 | Combined Hospital Cohort, C Index=0.79 | ||||
---|---|---|---|---|---|---|
Estimate | Odds Ratio (95% CI) | Estimate | Odds Ratio (95% CI) | Estimate | Odds Ratio (95% CI) | |
| ||||||
Cancer | 1.92 | 6.85 (2.83‐16.59)a | 1.85 | 6.36 (3.54‐11.41)a | 1.98 | 7.23 (4.45‐11.75)a |
Admissions to the hospital 2 in past year | 0.55 | 1.74 (0.76‐3.97) | 0.14 | 0.87 (0.51‐1.49) | 0.20 | 1.22 (0.78‐1.91) |
Resident in a nursing home | 0.49 | 0.61 (0.06‐6.56) | 0.27 | 1.31 (0.37‐4.66) | 0.09 | 1.09 (0.36‐3.32) |
ICU with MOF | 1.85 | 6.34 (2.0219.90)a | 1.94 | 6.97 (2.75‐17.68)a | ||
NHPCO (2) noncancer guidelines | 3.04 | 20.86 (4.25102.32)a | 2.62 | 13.73 (5.86‐32.15)a | 2.74 | 15.55 (7.2833.23)a |
Ageb | 0.38 | 1.46 (1.05‐2.03)a | 0.45 | 1.56 (1.23‐1.98)a | 0.47 | 1.60 (1.32‐1.93)a |
In the safety‐net hospital, admission to the hospital with a primary diagnosis related to cancer, 2 noncancer hospice guidelines, ICU admission with MOF, and age by category all were significant predictors of 1‐year mortality. In the university hospital cohort, primary diagnosis of cancer, 2 noncancer hospice guidelines, and age by category were predictive of 1‐year mortality. Finally, in the entire study cohort, primary diagnosis of cancer, ICU with MOF, 2 noncancer hospice guidelines, and age were all predictive of 1‐year mortality. Parameter estimates were similar in 3 of the criteria compared to the VA setting. Differences in patient characteristics may have caused the differences in the estimates. Gender was additionally tested but not significant in any model. One‐year survival was significantly lower for those who met 1 of the CARING criteria versus those who did not (Figure 1).
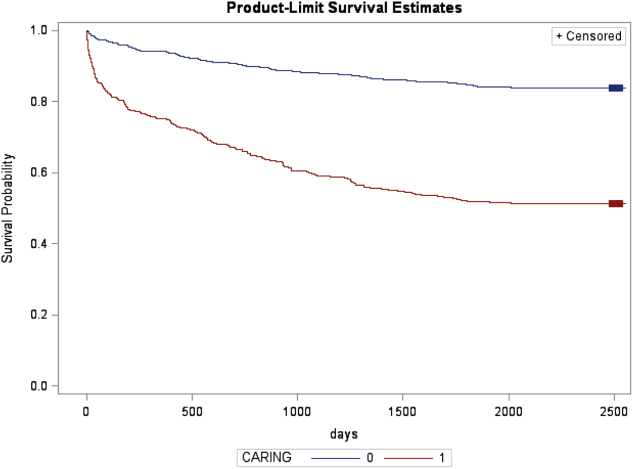
Based on the framework from the original CARING criteria analysis, a scoring rule was developed using the regression results of this validation cohort. To predict a high probability of 1‐year mortality, sensitivity was set to 58% and specificity was set at 86% (error rate=17%). Medium to high probability was set with a sensitivity of 73% and specificity of 72% (error rate=28%). The coefficients from the regression model of the entire study cohort were converted to scores for each of the CARING criteria. The scores are as follows: 0.5 points for admission from a nursing home, 1 point for 2 hospital admissions in the past year for a chronic illness, 10 points for primary diagnosis of cancer, 10 points for ICU admission with MOF, and 14 points for 2 noncancer hospice guidelines. For every age category increase, 2 points are assigned so that 0 points for age <55 years, 2 points for ages 56 to 65 years, 4 points for ages 66 to 75 years, and 6 points for >75 years. Points for individual risk factors were proportional to s (ie, log odds) in the logistic regression model for death at 1 year. Although no linear transformation exists between s and probabilities (of death at 1 year), the aggregated points for combinations of risk factors shown in Table 4 follow the probabilities in an approximately linear fashion, so that different degrees of risk of death can be represented contiguously (as highlighted by differently shaded regions in the scoring matrix) (Table 4). The scoring matrix allows for quick identification for patients at high risk for 1‐year mortality. In this non‐VA setting with healthier patients, low risk is defined at a lower probability threshold (0.1) compared to the VA setting (0.175).
CARING Criteria Components | |||||||
---|---|---|---|---|---|---|---|
None | Resident in a Nursing Home | Admitted to the Hospital 2 Times in the Past Year | Resident in a Nursing Home Admitted to the Hospital 2 Times in the Past Year | Primary Diagnosis of Cancer | ICU Admission With MOF | Noncancer Hospice Guidelines | |
| |||||||
Age | |||||||
55 years | 0 | 0.5 | 1 | 1.5 | 10 | ||
5565 years | 2 | 2.5 | 3 | 3.5 | 10 | ||
6675 years | 4 | 4.5 | 5 | 5.5 | 10 | ||
>75 years | 6 | 6.5 | 7 | 7.5 | 10 | ||
Risk | |||||||
Low | 3.5 | Probability<0.1 | |||||
Medium | 46.5 | 0.1probability <0.175 | |||||
High | 7 | Probability0.175 |
DISCUSSION
The CARING criteria are a practical prognostic tool that can be easily and rapidly applied to patients admitted to the hospital to estimate risk of death in 1 year, with the goal of identifying patients who may benefit most from incorporating palliative interventions into their plan of care. This study validated the CARING criteria in a tertiary referral university hospital and safety‐net hospital setting, demonstrating applicability in a much broader population than the VA hospital of the original CARING criteria study. The population studied represented a younger population by over 10 years, a more equitable proportion of males to females, a broader ethnic diversity, and lower 1‐year deaths rates than the original study. Despite the broader representation of the population, the significance of each of the individual CARING criterion was maintained except for 2 hospital admissions in the past year for a chronic illness (admission from a nursing home did not meet significance in either study as a sole criterion). As with the original study, meeting 2 of the NHPCO noncancer hospice guidelines demonstrated the highest risk of 1‐year mortality following index hospitalization, followed by primary diagnosis of cancer and ICU admission with MOF. Advancing age, also similar to the original study, conferred increased risk across the criterion.
Hospitalists could be an effective target for utilizing the CARING criteria because they are frequently the first‐line providers in the hospital setting. With the national shortage of palliative care specialists, hospitalists need to be able to identify when a patient has a limited life expectancy so they will be better equipped to make clinical decisions that are aligned with their patients' values, preferences, and goals of care. With the realization that not addressing advance care planning and patient goals of care may be considered medical errors, primary palliative care skills become alarmingly more important as priorities for hospitalists to obtain and feel comfortable using in daily practice.
The CARING criteria are directly applicable to patients who are seen by hospitalists. Other prognostic indices have focused on select patient populations, such as the elderly,[10, 11, 12] require collection of data that are not readily available on admission or would not otherwise be obtained,[10, 13] or apply to patients post‐hospital discharge, thereby missing the opportunity to make an impact earlier in the disease trajectory and incorporate palliative care into the hospital plan of care when key discussions about goals of care and preferences should be encouraged.
Additionally, the CARING criteria could easily be incorporated as a trigger for palliative care consults on hospital admission. Palliative care consults tend to happen late in a hospital stay, limiting the effectiveness of the palliative care team. A trigger system for hospitalists and other primary providers on hospital admission would lend to more effective timing of palliative measures being incorporated into the plan of care. Palliative care consults would not only be initiated earlier, but could be targeted for the more complex and sick patients with the highest risk of death in the next year.
In the time‐pressured environment, the presence of any 1 of the CARING criteria can act as a trigger to begin incorporating primary palliative care measures into the plan of care. The admitting hospitalist provider (ie, physician, nurse practitioner, physician assistant) could access the CARING criteria through an electronic health record prompt when admitting patients. When a more detailed assessment of mortality risk is helpful, the hospitalist can use the scoring matrix, which combines age with the individual criterion to calculate patients at medium or high risk of death within 1 year. Limited resources can then be directed to the patients with the greatest need. Patients with a focused care need, such as advance care planning or hospice referral, can be directed to the social worker or case manager. More complicated patients may be referred to a specialty palliative care team.
Several limitations to this study are recognized, including the small sample size of patients meeting criterion for ICU with MOF in the academic center study cohort. The patient data were collected during a transition time when the university hospital moved to a new campus, resulting in an ICU at each campus that housed patients with differing levels of illness severity, which may have contributed to the lower acuity ICU patient observed. Although we advocate the simplicity of the CARING criteria, the NHPCO noncancer hospice guidelines are more complicated, as they incorporates 8 broad categories of chronic illness. The hospice guidelines may not be general knowledge to the hospitalist or other primary providers. ePrognosis (
CONCLUSION
The CARING criteria are a simple, practical prognostic tool predictive of death within 1 year that has been validated in a broad population of hospitalized patients. The criteria hold up in a younger, healthier population that is more diverse by age, gender, and ethnicity than the VA population. With ready access to critical prognostic information on hospital admission, clinicians will be better informed to make decisions that are aligned with their patients' values, preferences, and goals of care.
Disclosure
Nothing to report.
Prognostication continues to be a challenge to the clinician despite over 100 prognostic indices that have been developed during the past few decades to inform clinical practice and medical decision making.[1] Physicians are not accurate in prognostication of patients' risk of death and tend to overestimate survival.[2, 3] In addition, many physicians do not feel comfortable offering a prognosis to patients, despite patients' wishes to be informed.[4, 5] Regardless of the prevalence in the literature and value in improving physicians' prognostic accuracy, prognostic indices of survival are not regularly utilized in the hospital setting. Prognostic tools available for providers are often complicated and may require data about patients that are not readily available.[6, 7, 8] Prognostic indices may be too specific to a patient population, too difficult to remember, or too time consuming to use. A simple, rapid, and practical prognostic index is important in the hospital setting to assist in identifying patients at high risk of death so that primary palliative interventions can be incorporated into the plan of care early in the hospital stay. Patient and family education, advance care planning, formulating the plan of care based on patientfamily goals, and improved resource utilization could be better executed by more accurate risk of death prediction on hospital admission.
The CARING criteria are the only prognostic index to our knowledge that evaluates a patient's risk of death in the next year, with information readily available at the time of hospital admission (Table 1).[9] The CARING criteria are a unique prognostic tool: (1) CARING is a mnemonic acronym, making it more user friendly to the clinician. (2) The 5 prognostic indicators are readily available from the patient's chart on admission; gathering further data by patient or caretaker interviews or by obtaining laboratory data is not needed. (3) The timing for application of the tool on admission to the hospital is an ideal opportunity to intervene and introduce palliative interventions early on the hospital stay. The CARING criteria were developed and validated in a Veteran's Administration hospital setting by Fischer et al.[9] We sought to validate the CARING criteria in a broader patient populationmedical and surgical patients from a tertiary referral university hospital setting and a safety‐net hospital setting.
METHODS
Study Design
This study was a retrospective observational cohort study. The study was approved by the Colorado Multiple Institutional Review Board and the University of Colorado Hospital Research Review Committee.
Study Purpose
To validate the CARING criteria in a tertiary referral university hospital (University of Colorado Hospital [UCH]) and safety‐net hospital (Denver Health and Hospitals [DHH]) setting using similar methodology to that employed by the original CARING criteria study.[9]
Study Setting/Population
All adults (18 years of age) admitted as inpatients to the medical and surgical services of internal medicine, hospitalist, pulmonary, cardiology, hematology/oncology, hepatology, surgery, intensive care unit, and intermediary care unit at UCH and DHH during the study period of July 2005 through August 2005. The only exclusion criteria were those patients who were prisoners or pregnant. Administrative admission data from July 2005 to August 2005 were used to identify names of all persons admitted to the medicine and surgical services of the study hospitals during the specified time period.
The 2 study hospitals, UCH and DHH, provide a range of patients who vary in ethnicity, socioeconomic status, and medical illness. This variability allows for greater generalizability of the results. Both hospitals are affiliated with the University of Colorado School of Medicine internal medicine residency training program and are located in Denver, Colorado.
At the time of the study, UCH was a licensed 550‐bed tertiary referral, academic hospital serving the Denver metropolitan area and the Rocky Mountain region as a specialty care and referral center. DHH was a 398‐bed, academic, safety‐net hospital serving primarily the Denver metropolitan area. DHH provides 42% of the care for the uninsured in Denver and 26% of the uninsured care for the state of Colorado.
Measures
The CARING criteria were developed and validated in a Veteran's Administration (VA) hospital setting by Fischer et al.[9] The purpose of the CARING criteria is to identify patients, at the time of hospital admission, who are at higher risk of death in the following year. The prognostic index uses 5 predictors that can be abstracted from the chart at time of admission. The CARING criteria were developed a priori, and patients were evaluated using only the medical data available at the time of admission. The criteria include items that are already part of the routine physician admission notes and do not require additional data collection or assessments. The criteria include: C=primary diagnosis of cancer, A=2 admissions to the hospital for a chronic illness within the last year; R=resident in a nursing home; I=intensive care unit (ICU) admission with multiorgan failure (MOF), NG=noncancer hospice guidelines (meeting 2 of the National Hospice and Palliative Care Organization's [NHPCO] guidelines).
Patients were identified using name, date of birth, social security number, address, and phone number. This identifying information was then used for tracing death records 1 year after hospital admission.
Mortality at 1 year following the index hospitalization was the primary end point. To minimize missing data and the number of subjects lost to follow‐up, 3 determinants of mortality were used. First, electronic medical records of the 2 participating hospitals and their outpatient clinics were reviewed to determine if a follow‐up appointment had occurred past the study's end point of 1 year (August 2006). For those without a confirmed follow‐up visit, death records from the Colorado Department of Public Health and Vital Records were obtained. For those patients residing outside of Colorado or whose mortality status was still unclear, the National Death Index was accessed.
Medical Record Review
Medical records for all study participants were reviewed by J.Y. (UCH) and B.C. (DHH). Data collection was completed using direct data entry into a Microsoft Access (Microsoft Corp., Redmond, WA) database utilizing a data entry form linked with the database table. This form utilized skip patterns and input masks to ensure quality of data entry and minimize missing or invalid data. Inter‐rater reliability was assessed by an independent rereview (S.F.) of 5% of the total charts. Demographic variables were collected using hospital administrative data. These included personal identifiers of the participants for purposes of mortality follow‐up. Clinical data including the 5 CARING variables and additional descriptive variables were abstracted from the paper hospital chart and the electronic record of the chart (together these constitute the medical record).
Death Follow‐up
A search of Colorado death records was conducted in February 2011 for all subjects. Death records were used to determine mortality and time to death from the index hospitalization. The National Death Index was then searched for any subjects without or record of death in Colorado.
Analysis
All analyses were conducted using the statistical application software SAS for Windows version 9.3 (SAS Institute, Cary, NC). Simple frequencies and means ( standard deviation) were used to describe the baseline characteristics. Multiple logistic regression models were used to model 1‐year mortality. The models were fitted using all of the CARING variables and age. As the aim of the study was to validate the CARING criteria, the variables for the models were selected a priori based on the original index. Two hospital cohorts (DHH and UCH) were modeled separately and as a combined sample. Kaplan‐Meier survival analysis was conducted to compare those subjects who met 1 of the CARING criteria with those who did not through the entire period of mortality follow‐up (20052011). Finally, using the probabilities from the logistic regression models, we again developed a scoring rule appropriate for a non‐VA setting to allow clinicians to easily identify patient risk for 1‐year mortality at the time of hospital admission.
RESULTS
There were a total of 1064 patients admitted to the medical and surgical services during the study period568 patients at DHH and 496 patients at UCH. Sample characteristics of each individual hospital cohort and the entire combined study cohort are detailed in Table 2. Overall, slightly over half the population were male, with a mean age of 50 years, and the ethnic breakdown roughly reflects the population in Denver. A total of 36.5% (n=388) of the study population met 1 of the CARING criteria, and 12.6% (n=134 among 1063 excluding 1 without an admit date) died within 1 year of the index hospitalization. These were younger and healthier patients compared to the VA sample used in developing the CARING criteria.
| |
Renal | Dementia |
Stop/decline dialysis | Unable to ambulate independently |
Not candidate for transplant | Urinary or fecal incontinence |
Urine output < 40cc/24 hours | Unable to speak with more than single words |
Creatinine > 8.0 (>6.0 for diabetics) | Unable to bathe independently |
Creatinine clearance 10cc/min | Unable to dress independently |
Uremia | Co‐morbid conditions: |
Persistent serum K + > 7.0 | Aspiration pneumonia |
Co‐morbid conditions: | Pyelonephritis |
Cancer CHF | Decubitus ulcer |
Chronic lung disease AIDS/HIV | Difficulty swallowing or refusal to eat |
Sepsis Cirrhosis | |
Cardiac | Pulmonary |
Ejection fraction < 20% | Dyspnea at rest |
Symptomatic with diuretics and vasodilators | FEV1 < 30% |
Not candidate for transplant | Frequent ER or hospital admits for pulmonary infections or respiratory distress |
History of cardiac arrest | Cor pulmonale or right heart failure |
History of syncope | 02 sat < 88% on 02 |
Systolic BP < 120mmHG | PC02 > 50 |
CVA cardiac origin | Resting tachycardia > 100/min |
Co‐morbid conditions as listed in Renal | Co‐morbid conditions as listed in Renal |
Liver | Stroke/CVA |
End stage cirrhosis | Coma at onset |
Not candidate for transplant | Coma >3 days |
Protime > 5sec and albumin <2.5 | Limb paralysis |
Ascites unresponsive to treatment | Urinary/fecal incontinence |
Hepatorenal syndrome | Impaired sitting balance |
Hepatic encephalopathy | Karnofsky < 50% |
Spontaneous bacterial peritonitis | Recurrent aspiration |
Recurrent variceal bleed | Age > 70 |
Co‐morbid conditions as listed in Renal | Co‐morbid conditions as listed in Renal |
HIV/AIDS | Neuromuscular |
Persistent decline in function | Diminished respiratory function |
Chronic diarrhea 1 year | Chosen not to receive BiPAP/vent |
Decision to stop treatment | Difficulty swallowing |
CNS lymphoma | Diminished functional status |
MAC‐untreated | Incontinence |
Systemic lymphoma | Co‐morbid conditions as listed in Renal |
Dilated cardiomyopathy | |
CD4 < 25 with disease progression | |
Viral load > 100,000 |
Safety‐Net Hospital Cohort, N=568 | Academic Center Cohort, N=496 | Study Cohort,N=1064 | Original CARING Cohort, N=8739 | |
---|---|---|---|---|
| ||||
Mean age ( SD), y | 47.8 (16.5) | 54.4 (17.5) | 50.9 (17.3) | 63 (13) |
Male gender | 59.5% (338) | 50.1% (248) | 55.1% (586) | 98% (856) |
Ethnicity | ||||
African American | 14.1% (80) | 13.5% (65) | 13.8% (145) | 13% (114) |
Asian | 0.4% (2) | 1.5% (7) | 0.9% (9) | Not reported |
Caucasian | 41.7% (237) | 66.3% (318) | 53.0 % (555) | 69% (602) |
Latino | 41.9% (238) | 9.6% (46) | 27.1% (284) | 8% (70) |
Native American | 0.5% (3) | 0.4% (2) | 0.5% (5) | Not reported |
Other | 0.5% (3) | 0.6% (3) | 0.6% (6) | 10% (87) |
Unknown | 0.9% (5) | 8.1% (39) | 4.2% (44) | Not reported |
CARING criteria | ||||
Cancer | 6.2% (35) | 19.4% (96) | 12.3% (131) | 23% (201) |
Admissions to the hospital 2 in past year | 13.6% (77) | 42.7% (212) | 27.2% (289) | 36% (314) |
Resident in a nursing home | 1.8% (10) | 3.4% (17) | 2.5% (27) | 3% (26) |
ICU with MOF | 3.7% (21) | 1.2% (6) | 2.5% (27) | 2% (17) |
NHPCO (2) noncancer guidelines | 1.6% (9) | 5.9% (29) | 3.6% (38) | 8% (70) |
Reliability testing demonstrated excellent inter‐rater reliability. Kappa for each criterion is as follows: (1) primary diagnosis of cancer=1.0, (2) 2 admissions to the hospital in the past year=0.91, (3) resident in a nursing home=1.0, (4) ICU admission with MOF=1.0, and (5) 2 noncancer hospice guidelines=0.78.
This study aimed to validate the CARING criteria9; therefore, all original individual CARING criterion were included in the validation logistic regression models. The 1 exception to this was in the university hospital study cohort, where the ICU criterion was excluded from the model due to small sample size and quasiseparation in the model. The model results are presented in Table 3 for the individual hospitals and combined study cohort.
Safety Net Hospital Cohort, C Index=0.76 | Academic Center Cohort, C Index=0.76 | Combined Hospital Cohort, C Index=0.79 | ||||
---|---|---|---|---|---|---|
Estimate | Odds Ratio (95% CI) | Estimate | Odds Ratio (95% CI) | Estimate | Odds Ratio (95% CI) | |
| ||||||
Cancer | 1.92 | 6.85 (2.83‐16.59)a | 1.85 | 6.36 (3.54‐11.41)a | 1.98 | 7.23 (4.45‐11.75)a |
Admissions to the hospital 2 in past year | 0.55 | 1.74 (0.76‐3.97) | 0.14 | 0.87 (0.51‐1.49) | 0.20 | 1.22 (0.78‐1.91) |
Resident in a nursing home | 0.49 | 0.61 (0.06‐6.56) | 0.27 | 1.31 (0.37‐4.66) | 0.09 | 1.09 (0.36‐3.32) |
ICU with MOF | 1.85 | 6.34 (2.0219.90)a | 1.94 | 6.97 (2.75‐17.68)a | ||
NHPCO (2) noncancer guidelines | 3.04 | 20.86 (4.25102.32)a | 2.62 | 13.73 (5.86‐32.15)a | 2.74 | 15.55 (7.2833.23)a |
Ageb | 0.38 | 1.46 (1.05‐2.03)a | 0.45 | 1.56 (1.23‐1.98)a | 0.47 | 1.60 (1.32‐1.93)a |
In the safety‐net hospital, admission to the hospital with a primary diagnosis related to cancer, 2 noncancer hospice guidelines, ICU admission with MOF, and age by category all were significant predictors of 1‐year mortality. In the university hospital cohort, primary diagnosis of cancer, 2 noncancer hospice guidelines, and age by category were predictive of 1‐year mortality. Finally, in the entire study cohort, primary diagnosis of cancer, ICU with MOF, 2 noncancer hospice guidelines, and age were all predictive of 1‐year mortality. Parameter estimates were similar in 3 of the criteria compared to the VA setting. Differences in patient characteristics may have caused the differences in the estimates. Gender was additionally tested but not significant in any model. One‐year survival was significantly lower for those who met 1 of the CARING criteria versus those who did not (Figure 1).
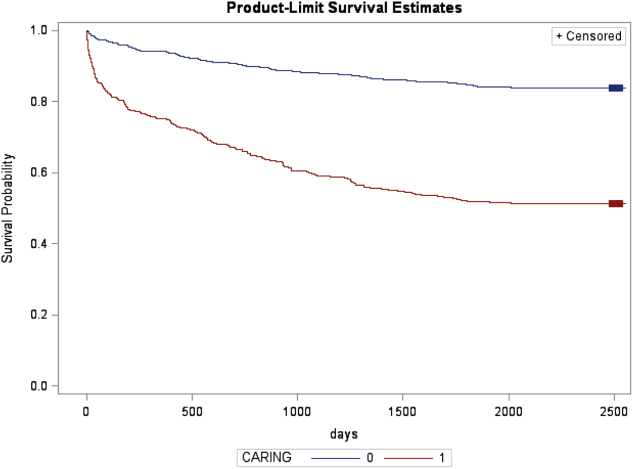
Based on the framework from the original CARING criteria analysis, a scoring rule was developed using the regression results of this validation cohort. To predict a high probability of 1‐year mortality, sensitivity was set to 58% and specificity was set at 86% (error rate=17%). Medium to high probability was set with a sensitivity of 73% and specificity of 72% (error rate=28%). The coefficients from the regression model of the entire study cohort were converted to scores for each of the CARING criteria. The scores are as follows: 0.5 points for admission from a nursing home, 1 point for 2 hospital admissions in the past year for a chronic illness, 10 points for primary diagnosis of cancer, 10 points for ICU admission with MOF, and 14 points for 2 noncancer hospice guidelines. For every age category increase, 2 points are assigned so that 0 points for age <55 years, 2 points for ages 56 to 65 years, 4 points for ages 66 to 75 years, and 6 points for >75 years. Points for individual risk factors were proportional to s (ie, log odds) in the logistic regression model for death at 1 year. Although no linear transformation exists between s and probabilities (of death at 1 year), the aggregated points for combinations of risk factors shown in Table 4 follow the probabilities in an approximately linear fashion, so that different degrees of risk of death can be represented contiguously (as highlighted by differently shaded regions in the scoring matrix) (Table 4). The scoring matrix allows for quick identification for patients at high risk for 1‐year mortality. In this non‐VA setting with healthier patients, low risk is defined at a lower probability threshold (0.1) compared to the VA setting (0.175).
CARING Criteria Components | |||||||
---|---|---|---|---|---|---|---|
None | Resident in a Nursing Home | Admitted to the Hospital 2 Times in the Past Year | Resident in a Nursing Home Admitted to the Hospital 2 Times in the Past Year | Primary Diagnosis of Cancer | ICU Admission With MOF | Noncancer Hospice Guidelines | |
| |||||||
Age | |||||||
55 years | 0 | 0.5 | 1 | 1.5 | 10 | ||
5565 years | 2 | 2.5 | 3 | 3.5 | 10 | ||
6675 years | 4 | 4.5 | 5 | 5.5 | 10 | ||
>75 years | 6 | 6.5 | 7 | 7.5 | 10 | ||
Risk | |||||||
Low | 3.5 | Probability<0.1 | |||||
Medium | 46.5 | 0.1probability <0.175 | |||||
High | 7 | Probability0.175 |
DISCUSSION
The CARING criteria are a practical prognostic tool that can be easily and rapidly applied to patients admitted to the hospital to estimate risk of death in 1 year, with the goal of identifying patients who may benefit most from incorporating palliative interventions into their plan of care. This study validated the CARING criteria in a tertiary referral university hospital and safety‐net hospital setting, demonstrating applicability in a much broader population than the VA hospital of the original CARING criteria study. The population studied represented a younger population by over 10 years, a more equitable proportion of males to females, a broader ethnic diversity, and lower 1‐year deaths rates than the original study. Despite the broader representation of the population, the significance of each of the individual CARING criterion was maintained except for 2 hospital admissions in the past year for a chronic illness (admission from a nursing home did not meet significance in either study as a sole criterion). As with the original study, meeting 2 of the NHPCO noncancer hospice guidelines demonstrated the highest risk of 1‐year mortality following index hospitalization, followed by primary diagnosis of cancer and ICU admission with MOF. Advancing age, also similar to the original study, conferred increased risk across the criterion.
Hospitalists could be an effective target for utilizing the CARING criteria because they are frequently the first‐line providers in the hospital setting. With the national shortage of palliative care specialists, hospitalists need to be able to identify when a patient has a limited life expectancy so they will be better equipped to make clinical decisions that are aligned with their patients' values, preferences, and goals of care. With the realization that not addressing advance care planning and patient goals of care may be considered medical errors, primary palliative care skills become alarmingly more important as priorities for hospitalists to obtain and feel comfortable using in daily practice.
The CARING criteria are directly applicable to patients who are seen by hospitalists. Other prognostic indices have focused on select patient populations, such as the elderly,[10, 11, 12] require collection of data that are not readily available on admission or would not otherwise be obtained,[10, 13] or apply to patients post‐hospital discharge, thereby missing the opportunity to make an impact earlier in the disease trajectory and incorporate palliative care into the hospital plan of care when key discussions about goals of care and preferences should be encouraged.
Additionally, the CARING criteria could easily be incorporated as a trigger for palliative care consults on hospital admission. Palliative care consults tend to happen late in a hospital stay, limiting the effectiveness of the palliative care team. A trigger system for hospitalists and other primary providers on hospital admission would lend to more effective timing of palliative measures being incorporated into the plan of care. Palliative care consults would not only be initiated earlier, but could be targeted for the more complex and sick patients with the highest risk of death in the next year.
In the time‐pressured environment, the presence of any 1 of the CARING criteria can act as a trigger to begin incorporating primary palliative care measures into the plan of care. The admitting hospitalist provider (ie, physician, nurse practitioner, physician assistant) could access the CARING criteria through an electronic health record prompt when admitting patients. When a more detailed assessment of mortality risk is helpful, the hospitalist can use the scoring matrix, which combines age with the individual criterion to calculate patients at medium or high risk of death within 1 year. Limited resources can then be directed to the patients with the greatest need. Patients with a focused care need, such as advance care planning or hospice referral, can be directed to the social worker or case manager. More complicated patients may be referred to a specialty palliative care team.
Several limitations to this study are recognized, including the small sample size of patients meeting criterion for ICU with MOF in the academic center study cohort. The patient data were collected during a transition time when the university hospital moved to a new campus, resulting in an ICU at each campus that housed patients with differing levels of illness severity, which may have contributed to the lower acuity ICU patient observed. Although we advocate the simplicity of the CARING criteria, the NHPCO noncancer hospice guidelines are more complicated, as they incorporates 8 broad categories of chronic illness. The hospice guidelines may not be general knowledge to the hospitalist or other primary providers. ePrognosis (
CONCLUSION
The CARING criteria are a simple, practical prognostic tool predictive of death within 1 year that has been validated in a broad population of hospitalized patients. The criteria hold up in a younger, healthier population that is more diverse by age, gender, and ethnicity than the VA population. With ready access to critical prognostic information on hospital admission, clinicians will be better informed to make decisions that are aligned with their patients' values, preferences, and goals of care.
Disclosure
Nothing to report.
- Predicting death: an empirical evaluation of predictive tools for mortality. Arch Intern Med. 2011;171:1721–1726. , , .
- Extent and determinants of error in physicians' prognoses in terminally ill patients: prospective cohort study. West J Med. 2000;172:310–313. , .
- A systematic review of physicians' survival predictions in terminally ill cancer patients. BMJ. 2003;327:195–198. , , , et al.
- Attitude and self‐reported practice regarding prognostication in a national sample of internists. Arch Intern Med. 1998;158:2389–2395. , .
- Discussing prognosis: balancing hope and realism. Cancer J. 2010;16:461–466. , , , et al.
- Acute Physiology and Chronic Health Evaluation (APACHE) IV: hospital mortality assessment for today's critically ill patients. Crit Care Med. 2006;34:1297–1310. , , , .
- SAPS 3 admission score: an external validation in a general intensive care population. Intensive Care Med. 2008;34:1873–1877. , , , , .
- Prospective validation of the intensive care unit admission Mortality Probability Model (MPM0‐III). Crit Care Med. 2009;37:1619–1623. , , , , , .
- A practical tool to identify patients who may benefit from a palliative approach: the CARING criteria. J Pain Symptom Manage. 2006;31:285–292. , , , , , .
- Prediction of survival for older hospitalized patients: the HELP survival model. J Am Geriatr Soc. 2000;48:S16–S24. , , , et al.
- Development and validation of a multidimensional prognostic index for one‐year mortality from comprehensive geriatric assessment in hospitalized older patients. Rejuvenation Res. 2008;11:151–161. , , , et al.
- Burden of illness score for elderly persons: risk adjustment incorporating the cumulative impact of diseases, physiologic abnormalities, and functional impairments. Med Care. 2003;41:70–83. , , , et al.
- The SUPPORT prognostic model. Objective estimates of survival for seriously ill hospitalized adults. Study to understand prognoses and preferences for outcomes and risks of treatments. Ann Intern Med. 1995;122:191–203. , , , et al.
- Predicting death: an empirical evaluation of predictive tools for mortality. Arch Intern Med. 2011;171:1721–1726. , , .
- Extent and determinants of error in physicians' prognoses in terminally ill patients: prospective cohort study. West J Med. 2000;172:310–313. , .
- A systematic review of physicians' survival predictions in terminally ill cancer patients. BMJ. 2003;327:195–198. , , , et al.
- Attitude and self‐reported practice regarding prognostication in a national sample of internists. Arch Intern Med. 1998;158:2389–2395. , .
- Discussing prognosis: balancing hope and realism. Cancer J. 2010;16:461–466. , , , et al.
- Acute Physiology and Chronic Health Evaluation (APACHE) IV: hospital mortality assessment for today's critically ill patients. Crit Care Med. 2006;34:1297–1310. , , , .
- SAPS 3 admission score: an external validation in a general intensive care population. Intensive Care Med. 2008;34:1873–1877. , , , , .
- Prospective validation of the intensive care unit admission Mortality Probability Model (MPM0‐III). Crit Care Med. 2009;37:1619–1623. , , , , , .
- A practical tool to identify patients who may benefit from a palliative approach: the CARING criteria. J Pain Symptom Manage. 2006;31:285–292. , , , , , .
- Prediction of survival for older hospitalized patients: the HELP survival model. J Am Geriatr Soc. 2000;48:S16–S24. , , , et al.
- Development and validation of a multidimensional prognostic index for one‐year mortality from comprehensive geriatric assessment in hospitalized older patients. Rejuvenation Res. 2008;11:151–161. , , , et al.
- Burden of illness score for elderly persons: risk adjustment incorporating the cumulative impact of diseases, physiologic abnormalities, and functional impairments. Med Care. 2003;41:70–83. , , , et al.
- The SUPPORT prognostic model. Objective estimates of survival for seriously ill hospitalized adults. Study to understand prognoses and preferences for outcomes and risks of treatments. Ann Intern Med. 1995;122:191–203. , , , et al.
© 2013 Society of Hospital Medicine
What is the best method of treating acutely worsened chronic pain?
Case
A 69-year-old female with metastatic ovarian cancer and chronic pain syndrome presented to the hospital with seven days of progressively worsening abdominal pain. The pain had been similar to her chronic cancer pain but more severe. She has acute renal failure secondary to volume depletion from poor intake. A CT scan of the abdomen and pelvis reveal progression of her cancer with acute pathology. What is the best method of treating this patient’s pain?
Overview
Pain is pandemic. It is the most common reason patients seek healthcare.1 Almost one-third of Americans will experience severe chronic pain at some point in their lives. Every year, approximately 25 million Americans experience acute pain and 50 million experience chronic pain. Only one in four patients with pain receives appropriate therapy and control of their pain.
Pain is the most common symptom experienced by hospitalized adults.2 Acute or chronic pain can be particularly challenging to treat because these patients are frequently opioid dependent and have many psychosocial factors. No one method of pain control is superior to another. However, one method to gain rapid control of an acute pain crisis in a patient with chronic pain is to use patient-controlled analgesia (PCA).
Review of the Data
The first commercially available PCA pumps became available in 1976.3 They were created after studies in the 1960s demonstrated that small doses of opioids given intravenously provided more effective pain relief than conventional intramuscular injections.
The majority of studies on PCAs are in the postoperative patient, with cancer pain being next most commonly studied. PCAs utilize microprocessor-controlled infusion pumps that deliver a preprogrammed dose of opioid when the patient pushes the demand button. They allow programming of dose (demand dose), time between doses (lockout interval), background infusion rate (basal rate), and nurse-initiated dose (bolus dose).
The PCA paradigm is based on the opioid pharmacologic concept of minimum effective analgesic concentration (MEAC).4,5 The MEAC is the smallest serum opioid concentration at which pain is relieved. The dose-response curve to opioids is sigmoidal such that minimal analgesia is achieved until the MEAC is reached, after which minute increases in opioid concentrations produce analgesia, until further increases produce no significant increased analgesic effect.
PCAs allow individualized dosing and titration to achieve the MEAC, with small incremental doses administered whenever the serum concentration falls below the MEAC. A major goal of PCA technology is to regulate drug delivery to rapidly achieve and maintain the MEAC.
Advantages of PCAs
- More individual dosing and titration of pain medications to account for inter-individual and intra-individual variability in the response to opioids;
- Negative feedback control system, an added safety measure to avoid respiratory depression. As patients become too sedated from opioids, they are no longer able to push the button to receive further opioids;
- Higher patient satisfaction with pain control, a major determinant being personal control over the delivery of pain relief;6-8 and
- Greater analgesic efficacy vs. conventional analgesia.
Disadvantages of PCAs
Select patient populations: Not all patients are able to understand and retain the required instructions necessary to safely or effectively use self-administered opioids (e.g., cognitively impaired patients).
Potential for opioid dosing errors: These are related to equipment factors, medical personnel prescribing or programming errors.
Increased cost: PCAs have been shown to be more expensive in comparison with intramuscular (IM) injections, the prior standard of care.9-10
PCA Prescribing
The parameters programmed into the PCA machine include the basal rate, demand (or incremental) dose, lockout interval, nurse-initiated bolus dose, and choice of opioid.
Basal rate: The continuous infusion of opioid set at an hourly rate. Most studies that compare PCA use with and without basal rates (in postoperative patients) do not show improved pain relief or sleep with basal rates.11 Basal rates have been associated with increased risk of sedation and respiratory depression.12
The routine use of basal rates is not recommended initially, unless a patient is opioid-tolerant (i.e., on chronic opioid therapy). For patients on chronic opioids, their 24-hour total opioid requirement is converted by equianalgesic dosing to the basal rate. Steady state is not achieved for eight to 12 hours of continuous infusion; therefore, it is not recommended to change the basal rate more frequently than every eight hours.13
Demand dose: The dose patients provide themselves by pushing the button. Studies on opioid-naïve patients using morphine PCAs have shown that 1 mg IV morphine was the optimal starting dose, based on good pain relief without respiratory depression. Lower doses, such as 0.5 mg IV morphine, are generally used in the elderly as opioid requirements are known to decrease with patient age.14
For patients with a basal rate, the demand dose is often set at 50% to 100% of the basal rate. The demand dose is the parameter that should be titrated up for acute pain control. World Health Organization guidelines recommend increasing the dose by 25% to 50% for mild to moderate pain, and 50% to 100% for moderate to severe pain.15
Lockout interval: Minimal allowable time between demand doses. This time is based on the time to peak effect of IV opioids and can vary from five to 15 minutes. The effects of varying lockout intervals—seven to 11 minutes for morphine and five to eight minutes for fentanyl—had no effect on pain levels or side effects.16 Ten minutes is a standard lockout interval.
Bolus dose: The nurse-initiated dose that may be given initially to achieve pain control and later to counteract incidental pain (e.g., that caused by physical therapy, dressing changes, or radiology tests). A recommended dose is equivalent to the basal rate or twice the demand dose.
Choice of opioid: Morphine is the standard opioid because of its familiarity, cost, and years of study. Although inter-individual variability exists, there are no major differences in side effects among the different opioids. Renal and hepatic insufficiency can increase the effects of opioids. Morphine is especially troublesome in renal failure because it has an active metabolite—morphine-6-glucuronide—that can accumulate and increase the risk of sedation and respiratory depression.
Other Concerns
PCA complications: The most well-studied adverse effects of PCAs are nausea and respiratory depression. There is no difference between PCAs and conventional analgesia in rates of nausea or respiratory depression.17
Nausea is the most common side effect in postoperative patients on PCAs. Patients rapidly develop tolerance to nausea over a period of days. However, many clinicians are concerned about respiratory depression and the risk of death. The overall incidence of respiratory depression with PCAs is less than 1% (range from 0.1 to 0.8%), similar to conventional analgesia. However, the incidence is significantly higher when basal rates are used, rising to 1.1 to 3.9%. Other factors predisposing a patient to increased risk of respiratory depression are older age, obstructive sleep apnea, hypovolemia, renal failure, and the concurrent use of other sedating medications.18
Medication errors are also common. The overall incidence of medication mishaps with PCAs is 1.2%.19 More than 50% of these occur because of operator-related errors (e.g., improper loading, programming errors, and documentation errors). Equipment malfunction is the next most common error.
Opioid equianalgesic dosing conversions: The equianalgesic dose ratio is the ratio of the dose of two opioids required to produce the same analgesic effect. (See Table 1, right.) For example, IV morphine is three times as potent as oral morphine, with an equianalgesic dose ratio of 1:3. Equianalgesic dose tables vary somewhat in their values, which have been largely determined by single-dose administration studies.20 The generalizability of these tables to chronic opioid administration is not well studied.
Incomplete cross tolerance: When switching from one opioid to another, lower doses can be used to control pain.21, 22 Tolerance to one opioid does not completely transfer to the new opioid. Starting at half to two-thirds of the new opioid dose is generally recommended to avoid opioid-specific tolerance and inter-individual variability.23,24
Back to the Case
Opioids are the mainstay of pharmacological management of moderate-to-severe cancer pain. Evaluation of the patient reveals that her acute increase in pain is likely due to progression of her cancer. She had been taking morphine (sustained-release, 90 mg oral) twice daily for her pain and had been using approximately five doses per day of immediate-release oral morphine 20 mg for breakthrough pain. This is equivalent to a total 24-hour opioid requirement of 280 mg oral morphine.
She should be started on a PCA for rapid pain control and titration. Hydromorphone (Dilaudid) is a better PCA choice than morphine because she has acute renal failure. The equianalgesic dose ratio of oral morphine to IV hydromorphone is approximately 30:1.5. The total 24-hour opioid dose of 280 mg oral morphine is equivalent to 14 mg IV hydromorphone ([280mg morphine per day ÷ 30] x 1.5 = 14).
After adjusting for 60% incomplete cross tolerance, the total 24-hour opioid dose is reduced to 8.4 mg IV hydromorphone (14 mg x 0.6 = 8.4 mg). This is approximately equivalent to 0.4 mg IV hydromorphone/hour (8.4 mg ÷ 24 hours), which is her initial basal rate. The demand dose should be set at 0.2 mg (50% the basal rate) with a lockout interval of 10 minutes.
Over a period of several days, the patient’s pain was controlled and her opioid requirements stabilized. She was on a basal rate of 1.4 mg/hour and a demand dose of 1 mg with a 10-minute lockout. Her total 24-hour opioid requirement was 44 mg of IV hydromorphone. As her renal function improved but did not completely normalize, oxycodone was chosen over morphine when converting her back to oral pain medications (less active renal metabolites). The equianalgesic dose ratio of oral oxycodone to IV hydromorphone is approximately 20:1.5. Her total 24-hour opioid dose of 44 mg IV hydromorphone is equivalent to 587 mg oral oxycodone (44 ÷ 1.5) x 20. After adjusting for 60% incomplete cross tolerance, the total 24-hour opioid dose is reduced to 352 mg oral oxycodone or 180 mg of sustained-release oxycodone twice daily (352 mg ÷ 2 ≈ 180 mg). For breakthrough pain she should receive 40 mg of immediate-release oxycodone every hour as needed (10% to 15% of the 24-hour opioid requirement). TH
Dr. Youngwerth is a hospitalist and instructor of medicine, University of Colorado at Denver, assistant director, Palliative Care Consult Service, associate director, Colorado Palliative Medicine Fellowship Program, and medical director, Hospice of Saint John.
References
- American Pain Society. Pain: Current understanding of assessment, management, and treatments. National Pharmaceutical Council 2006;1-79.
- Morrison RS, Meier DE, Fischberg D, et al. Improving the management of pain in hospitalized adults. Arch Intern Med. 2006;166:1033-1039.
- Grass JA. Patient-controlled analgesia. Anesth Analg. 2005;101:S44-S61.
- Etches RC. Patient-controlled analgesia. Surg Clinics N Amer. 1999;79:297-312.
- Nolan MF and Wilson M-C B. Patient-controlled analgesia: A method for the controlled self-administration of opioid pain medications. Phys Ther. 1995;75:374-379.
- Ballantyne JC, Carr DB, Chalmers TC, Dear KBG, Angelillo IF, Mosteller F. Postoperative patient-controlled analgesia: Meta-analyses of initial randomized control trials. J Clin Anesth. 1993;5:182-193.
- Hudcova J, McNicol E, Quah C, Lau J, Carr DB. Patient controlled opioid analgesia versus conventional opioid analgesia for postoperative pain. Cochrane Database of Systematic Reviews. 2006;4:1-10.
- Sidebotham D, Dijkhuizen MRJ, Schug SA. The safety and utilization of patient-controlled analgesia. J Pain Symptom Manage. 1997;14:202-209.
- Macintyre PE. Safety and efficacy of patient-controlled analgesia. Br J Anaesth. 2001;87:36-46.
- Manon C, Rittenhouse BE, Perreault S, et al. Efficacy and costs of patient-controlled analgesia versus regularly administered intramuscular opioid therapy. Amer Soc Anesth Inc. 1998;89:1377-1388.
- Krenn H, Oczenski W, Jellinek H, Krumpl-Ströher M, Schweitzer E, Fitzgerald RD. Nalbuphine by PCA-pump for analgesia following hysterectomy: Bolus application versus continuous infusion with bolus application. Eur J Pain. 2001;5:219-226.
- Lehmann KA. Recent developments in patient-controlled analgesia. J Pain Symptom Manage. 2005;29:S72-S89.
- American Pain Society. Principles of analgesic use in the treatment of acute pain and cancer pain. 5th ed. 2003:1-73.
- Macintyre PC, Jarvis DA. Age is the best predictor of postoperative morphine requirements. Pain. 1995;64:357-364.
- National Comprehensive Cancer Network. Clinical practice guidelines in oncology: Adult cancer pain. Version 2.2005:1-30.
- Ginsberg B, Gil KM, Muir M, Sullivan F, Williams DA, Glass PSA. The influence of lockout intervals and drug selection on patient-controlled analgesia following gynecological surgery. Pain. 1995;62:95-100.
- Walder B, Schafer M, Henzi I, Tramer MR. Efficacy and safety of patient-controlled opioid analgesia for acute postoperative pain. Acta Anaesthesiol Scand. 2001;45:795-804.
- Etches RC. Respiratory depression associated with patient-controlled analgesia: a review of eight cases. Can J Anaesth. 1994;41:125-132.
- Oswalt KE, Shrewsbury P, Stanton-Hicks M. The incidence of medication mishaps in 3,299 PCA patients. Pain. 1990;S5;S152.
- Pereira J, Lawlor P, Vigano A, Dorgan M, Bruera E. Equianalgesic dose rations for opioids: A critical review and proposals for long-term dosing. J Pain Symptom Manage. 2001;22:672-687.
- Ballantyne JC, Mao J. Opioid therapy for chronic pain. N Engl J Med. 2003;349:1943-1953.
- Mercandante S. Opioid rotation for cancer pain. Cancer. 1999;86:1856-1866.
- Mehta V, Langford RM. Acute pain management for opioid dependent patients. Anaesthesia. 2006;61:269-276.
- Pasternak GW. Incomplete cross tolerance and multiple mu opioid peptide receptors. Trends Pharm Sciences. 2001;22:67-70.
Case
A 69-year-old female with metastatic ovarian cancer and chronic pain syndrome presented to the hospital with seven days of progressively worsening abdominal pain. The pain had been similar to her chronic cancer pain but more severe. She has acute renal failure secondary to volume depletion from poor intake. A CT scan of the abdomen and pelvis reveal progression of her cancer with acute pathology. What is the best method of treating this patient’s pain?
Overview
Pain is pandemic. It is the most common reason patients seek healthcare.1 Almost one-third of Americans will experience severe chronic pain at some point in their lives. Every year, approximately 25 million Americans experience acute pain and 50 million experience chronic pain. Only one in four patients with pain receives appropriate therapy and control of their pain.
Pain is the most common symptom experienced by hospitalized adults.2 Acute or chronic pain can be particularly challenging to treat because these patients are frequently opioid dependent and have many psychosocial factors. No one method of pain control is superior to another. However, one method to gain rapid control of an acute pain crisis in a patient with chronic pain is to use patient-controlled analgesia (PCA).
Review of the Data
The first commercially available PCA pumps became available in 1976.3 They were created after studies in the 1960s demonstrated that small doses of opioids given intravenously provided more effective pain relief than conventional intramuscular injections.
The majority of studies on PCAs are in the postoperative patient, with cancer pain being next most commonly studied. PCAs utilize microprocessor-controlled infusion pumps that deliver a preprogrammed dose of opioid when the patient pushes the demand button. They allow programming of dose (demand dose), time between doses (lockout interval), background infusion rate (basal rate), and nurse-initiated dose (bolus dose).
The PCA paradigm is based on the opioid pharmacologic concept of minimum effective analgesic concentration (MEAC).4,5 The MEAC is the smallest serum opioid concentration at which pain is relieved. The dose-response curve to opioids is sigmoidal such that minimal analgesia is achieved until the MEAC is reached, after which minute increases in opioid concentrations produce analgesia, until further increases produce no significant increased analgesic effect.
PCAs allow individualized dosing and titration to achieve the MEAC, with small incremental doses administered whenever the serum concentration falls below the MEAC. A major goal of PCA technology is to regulate drug delivery to rapidly achieve and maintain the MEAC.
Advantages of PCAs
- More individual dosing and titration of pain medications to account for inter-individual and intra-individual variability in the response to opioids;
- Negative feedback control system, an added safety measure to avoid respiratory depression. As patients become too sedated from opioids, they are no longer able to push the button to receive further opioids;
- Higher patient satisfaction with pain control, a major determinant being personal control over the delivery of pain relief;6-8 and
- Greater analgesic efficacy vs. conventional analgesia.
Disadvantages of PCAs
Select patient populations: Not all patients are able to understand and retain the required instructions necessary to safely or effectively use self-administered opioids (e.g., cognitively impaired patients).
Potential for opioid dosing errors: These are related to equipment factors, medical personnel prescribing or programming errors.
Increased cost: PCAs have been shown to be more expensive in comparison with intramuscular (IM) injections, the prior standard of care.9-10
PCA Prescribing
The parameters programmed into the PCA machine include the basal rate, demand (or incremental) dose, lockout interval, nurse-initiated bolus dose, and choice of opioid.
Basal rate: The continuous infusion of opioid set at an hourly rate. Most studies that compare PCA use with and without basal rates (in postoperative patients) do not show improved pain relief or sleep with basal rates.11 Basal rates have been associated with increased risk of sedation and respiratory depression.12
The routine use of basal rates is not recommended initially, unless a patient is opioid-tolerant (i.e., on chronic opioid therapy). For patients on chronic opioids, their 24-hour total opioid requirement is converted by equianalgesic dosing to the basal rate. Steady state is not achieved for eight to 12 hours of continuous infusion; therefore, it is not recommended to change the basal rate more frequently than every eight hours.13
Demand dose: The dose patients provide themselves by pushing the button. Studies on opioid-naïve patients using morphine PCAs have shown that 1 mg IV morphine was the optimal starting dose, based on good pain relief without respiratory depression. Lower doses, such as 0.5 mg IV morphine, are generally used in the elderly as opioid requirements are known to decrease with patient age.14
For patients with a basal rate, the demand dose is often set at 50% to 100% of the basal rate. The demand dose is the parameter that should be titrated up for acute pain control. World Health Organization guidelines recommend increasing the dose by 25% to 50% for mild to moderate pain, and 50% to 100% for moderate to severe pain.15
Lockout interval: Minimal allowable time between demand doses. This time is based on the time to peak effect of IV opioids and can vary from five to 15 minutes. The effects of varying lockout intervals—seven to 11 minutes for morphine and five to eight minutes for fentanyl—had no effect on pain levels or side effects.16 Ten minutes is a standard lockout interval.
Bolus dose: The nurse-initiated dose that may be given initially to achieve pain control and later to counteract incidental pain (e.g., that caused by physical therapy, dressing changes, or radiology tests). A recommended dose is equivalent to the basal rate or twice the demand dose.
Choice of opioid: Morphine is the standard opioid because of its familiarity, cost, and years of study. Although inter-individual variability exists, there are no major differences in side effects among the different opioids. Renal and hepatic insufficiency can increase the effects of opioids. Morphine is especially troublesome in renal failure because it has an active metabolite—morphine-6-glucuronide—that can accumulate and increase the risk of sedation and respiratory depression.
Other Concerns
PCA complications: The most well-studied adverse effects of PCAs are nausea and respiratory depression. There is no difference between PCAs and conventional analgesia in rates of nausea or respiratory depression.17
Nausea is the most common side effect in postoperative patients on PCAs. Patients rapidly develop tolerance to nausea over a period of days. However, many clinicians are concerned about respiratory depression and the risk of death. The overall incidence of respiratory depression with PCAs is less than 1% (range from 0.1 to 0.8%), similar to conventional analgesia. However, the incidence is significantly higher when basal rates are used, rising to 1.1 to 3.9%. Other factors predisposing a patient to increased risk of respiratory depression are older age, obstructive sleep apnea, hypovolemia, renal failure, and the concurrent use of other sedating medications.18
Medication errors are also common. The overall incidence of medication mishaps with PCAs is 1.2%.19 More than 50% of these occur because of operator-related errors (e.g., improper loading, programming errors, and documentation errors). Equipment malfunction is the next most common error.
Opioid equianalgesic dosing conversions: The equianalgesic dose ratio is the ratio of the dose of two opioids required to produce the same analgesic effect. (See Table 1, right.) For example, IV morphine is three times as potent as oral morphine, with an equianalgesic dose ratio of 1:3. Equianalgesic dose tables vary somewhat in their values, which have been largely determined by single-dose administration studies.20 The generalizability of these tables to chronic opioid administration is not well studied.
Incomplete cross tolerance: When switching from one opioid to another, lower doses can be used to control pain.21, 22 Tolerance to one opioid does not completely transfer to the new opioid. Starting at half to two-thirds of the new opioid dose is generally recommended to avoid opioid-specific tolerance and inter-individual variability.23,24
Back to the Case
Opioids are the mainstay of pharmacological management of moderate-to-severe cancer pain. Evaluation of the patient reveals that her acute increase in pain is likely due to progression of her cancer. She had been taking morphine (sustained-release, 90 mg oral) twice daily for her pain and had been using approximately five doses per day of immediate-release oral morphine 20 mg for breakthrough pain. This is equivalent to a total 24-hour opioid requirement of 280 mg oral morphine.
She should be started on a PCA for rapid pain control and titration. Hydromorphone (Dilaudid) is a better PCA choice than morphine because she has acute renal failure. The equianalgesic dose ratio of oral morphine to IV hydromorphone is approximately 30:1.5. The total 24-hour opioid dose of 280 mg oral morphine is equivalent to 14 mg IV hydromorphone ([280mg morphine per day ÷ 30] x 1.5 = 14).
After adjusting for 60% incomplete cross tolerance, the total 24-hour opioid dose is reduced to 8.4 mg IV hydromorphone (14 mg x 0.6 = 8.4 mg). This is approximately equivalent to 0.4 mg IV hydromorphone/hour (8.4 mg ÷ 24 hours), which is her initial basal rate. The demand dose should be set at 0.2 mg (50% the basal rate) with a lockout interval of 10 minutes.
Over a period of several days, the patient’s pain was controlled and her opioid requirements stabilized. She was on a basal rate of 1.4 mg/hour and a demand dose of 1 mg with a 10-minute lockout. Her total 24-hour opioid requirement was 44 mg of IV hydromorphone. As her renal function improved but did not completely normalize, oxycodone was chosen over morphine when converting her back to oral pain medications (less active renal metabolites). The equianalgesic dose ratio of oral oxycodone to IV hydromorphone is approximately 20:1.5. Her total 24-hour opioid dose of 44 mg IV hydromorphone is equivalent to 587 mg oral oxycodone (44 ÷ 1.5) x 20. After adjusting for 60% incomplete cross tolerance, the total 24-hour opioid dose is reduced to 352 mg oral oxycodone or 180 mg of sustained-release oxycodone twice daily (352 mg ÷ 2 ≈ 180 mg). For breakthrough pain she should receive 40 mg of immediate-release oxycodone every hour as needed (10% to 15% of the 24-hour opioid requirement). TH
Dr. Youngwerth is a hospitalist and instructor of medicine, University of Colorado at Denver, assistant director, Palliative Care Consult Service, associate director, Colorado Palliative Medicine Fellowship Program, and medical director, Hospice of Saint John.
References
- American Pain Society. Pain: Current understanding of assessment, management, and treatments. National Pharmaceutical Council 2006;1-79.
- Morrison RS, Meier DE, Fischberg D, et al. Improving the management of pain in hospitalized adults. Arch Intern Med. 2006;166:1033-1039.
- Grass JA. Patient-controlled analgesia. Anesth Analg. 2005;101:S44-S61.
- Etches RC. Patient-controlled analgesia. Surg Clinics N Amer. 1999;79:297-312.
- Nolan MF and Wilson M-C B. Patient-controlled analgesia: A method for the controlled self-administration of opioid pain medications. Phys Ther. 1995;75:374-379.
- Ballantyne JC, Carr DB, Chalmers TC, Dear KBG, Angelillo IF, Mosteller F. Postoperative patient-controlled analgesia: Meta-analyses of initial randomized control trials. J Clin Anesth. 1993;5:182-193.
- Hudcova J, McNicol E, Quah C, Lau J, Carr DB. Patient controlled opioid analgesia versus conventional opioid analgesia for postoperative pain. Cochrane Database of Systematic Reviews. 2006;4:1-10.
- Sidebotham D, Dijkhuizen MRJ, Schug SA. The safety and utilization of patient-controlled analgesia. J Pain Symptom Manage. 1997;14:202-209.
- Macintyre PE. Safety and efficacy of patient-controlled analgesia. Br J Anaesth. 2001;87:36-46.
- Manon C, Rittenhouse BE, Perreault S, et al. Efficacy and costs of patient-controlled analgesia versus regularly administered intramuscular opioid therapy. Amer Soc Anesth Inc. 1998;89:1377-1388.
- Krenn H, Oczenski W, Jellinek H, Krumpl-Ströher M, Schweitzer E, Fitzgerald RD. Nalbuphine by PCA-pump for analgesia following hysterectomy: Bolus application versus continuous infusion with bolus application. Eur J Pain. 2001;5:219-226.
- Lehmann KA. Recent developments in patient-controlled analgesia. J Pain Symptom Manage. 2005;29:S72-S89.
- American Pain Society. Principles of analgesic use in the treatment of acute pain and cancer pain. 5th ed. 2003:1-73.
- Macintyre PC, Jarvis DA. Age is the best predictor of postoperative morphine requirements. Pain. 1995;64:357-364.
- National Comprehensive Cancer Network. Clinical practice guidelines in oncology: Adult cancer pain. Version 2.2005:1-30.
- Ginsberg B, Gil KM, Muir M, Sullivan F, Williams DA, Glass PSA. The influence of lockout intervals and drug selection on patient-controlled analgesia following gynecological surgery. Pain. 1995;62:95-100.
- Walder B, Schafer M, Henzi I, Tramer MR. Efficacy and safety of patient-controlled opioid analgesia for acute postoperative pain. Acta Anaesthesiol Scand. 2001;45:795-804.
- Etches RC. Respiratory depression associated with patient-controlled analgesia: a review of eight cases. Can J Anaesth. 1994;41:125-132.
- Oswalt KE, Shrewsbury P, Stanton-Hicks M. The incidence of medication mishaps in 3,299 PCA patients. Pain. 1990;S5;S152.
- Pereira J, Lawlor P, Vigano A, Dorgan M, Bruera E. Equianalgesic dose rations for opioids: A critical review and proposals for long-term dosing. J Pain Symptom Manage. 2001;22:672-687.
- Ballantyne JC, Mao J. Opioid therapy for chronic pain. N Engl J Med. 2003;349:1943-1953.
- Mercandante S. Opioid rotation for cancer pain. Cancer. 1999;86:1856-1866.
- Mehta V, Langford RM. Acute pain management for opioid dependent patients. Anaesthesia. 2006;61:269-276.
- Pasternak GW. Incomplete cross tolerance and multiple mu opioid peptide receptors. Trends Pharm Sciences. 2001;22:67-70.
Case
A 69-year-old female with metastatic ovarian cancer and chronic pain syndrome presented to the hospital with seven days of progressively worsening abdominal pain. The pain had been similar to her chronic cancer pain but more severe. She has acute renal failure secondary to volume depletion from poor intake. A CT scan of the abdomen and pelvis reveal progression of her cancer with acute pathology. What is the best method of treating this patient’s pain?
Overview
Pain is pandemic. It is the most common reason patients seek healthcare.1 Almost one-third of Americans will experience severe chronic pain at some point in their lives. Every year, approximately 25 million Americans experience acute pain and 50 million experience chronic pain. Only one in four patients with pain receives appropriate therapy and control of their pain.
Pain is the most common symptom experienced by hospitalized adults.2 Acute or chronic pain can be particularly challenging to treat because these patients are frequently opioid dependent and have many psychosocial factors. No one method of pain control is superior to another. However, one method to gain rapid control of an acute pain crisis in a patient with chronic pain is to use patient-controlled analgesia (PCA).
Review of the Data
The first commercially available PCA pumps became available in 1976.3 They were created after studies in the 1960s demonstrated that small doses of opioids given intravenously provided more effective pain relief than conventional intramuscular injections.
The majority of studies on PCAs are in the postoperative patient, with cancer pain being next most commonly studied. PCAs utilize microprocessor-controlled infusion pumps that deliver a preprogrammed dose of opioid when the patient pushes the demand button. They allow programming of dose (demand dose), time between doses (lockout interval), background infusion rate (basal rate), and nurse-initiated dose (bolus dose).
The PCA paradigm is based on the opioid pharmacologic concept of minimum effective analgesic concentration (MEAC).4,5 The MEAC is the smallest serum opioid concentration at which pain is relieved. The dose-response curve to opioids is sigmoidal such that minimal analgesia is achieved until the MEAC is reached, after which minute increases in opioid concentrations produce analgesia, until further increases produce no significant increased analgesic effect.
PCAs allow individualized dosing and titration to achieve the MEAC, with small incremental doses administered whenever the serum concentration falls below the MEAC. A major goal of PCA technology is to regulate drug delivery to rapidly achieve and maintain the MEAC.
Advantages of PCAs
- More individual dosing and titration of pain medications to account for inter-individual and intra-individual variability in the response to opioids;
- Negative feedback control system, an added safety measure to avoid respiratory depression. As patients become too sedated from opioids, they are no longer able to push the button to receive further opioids;
- Higher patient satisfaction with pain control, a major determinant being personal control over the delivery of pain relief;6-8 and
- Greater analgesic efficacy vs. conventional analgesia.
Disadvantages of PCAs
Select patient populations: Not all patients are able to understand and retain the required instructions necessary to safely or effectively use self-administered opioids (e.g., cognitively impaired patients).
Potential for opioid dosing errors: These are related to equipment factors, medical personnel prescribing or programming errors.
Increased cost: PCAs have been shown to be more expensive in comparison with intramuscular (IM) injections, the prior standard of care.9-10
PCA Prescribing
The parameters programmed into the PCA machine include the basal rate, demand (or incremental) dose, lockout interval, nurse-initiated bolus dose, and choice of opioid.
Basal rate: The continuous infusion of opioid set at an hourly rate. Most studies that compare PCA use with and without basal rates (in postoperative patients) do not show improved pain relief or sleep with basal rates.11 Basal rates have been associated with increased risk of sedation and respiratory depression.12
The routine use of basal rates is not recommended initially, unless a patient is opioid-tolerant (i.e., on chronic opioid therapy). For patients on chronic opioids, their 24-hour total opioid requirement is converted by equianalgesic dosing to the basal rate. Steady state is not achieved for eight to 12 hours of continuous infusion; therefore, it is not recommended to change the basal rate more frequently than every eight hours.13
Demand dose: The dose patients provide themselves by pushing the button. Studies on opioid-naïve patients using morphine PCAs have shown that 1 mg IV morphine was the optimal starting dose, based on good pain relief without respiratory depression. Lower doses, such as 0.5 mg IV morphine, are generally used in the elderly as opioid requirements are known to decrease with patient age.14
For patients with a basal rate, the demand dose is often set at 50% to 100% of the basal rate. The demand dose is the parameter that should be titrated up for acute pain control. World Health Organization guidelines recommend increasing the dose by 25% to 50% for mild to moderate pain, and 50% to 100% for moderate to severe pain.15
Lockout interval: Minimal allowable time between demand doses. This time is based on the time to peak effect of IV opioids and can vary from five to 15 minutes. The effects of varying lockout intervals—seven to 11 minutes for morphine and five to eight minutes for fentanyl—had no effect on pain levels or side effects.16 Ten minutes is a standard lockout interval.
Bolus dose: The nurse-initiated dose that may be given initially to achieve pain control and later to counteract incidental pain (e.g., that caused by physical therapy, dressing changes, or radiology tests). A recommended dose is equivalent to the basal rate or twice the demand dose.
Choice of opioid: Morphine is the standard opioid because of its familiarity, cost, and years of study. Although inter-individual variability exists, there are no major differences in side effects among the different opioids. Renal and hepatic insufficiency can increase the effects of opioids. Morphine is especially troublesome in renal failure because it has an active metabolite—morphine-6-glucuronide—that can accumulate and increase the risk of sedation and respiratory depression.
Other Concerns
PCA complications: The most well-studied adverse effects of PCAs are nausea and respiratory depression. There is no difference between PCAs and conventional analgesia in rates of nausea or respiratory depression.17
Nausea is the most common side effect in postoperative patients on PCAs. Patients rapidly develop tolerance to nausea over a period of days. However, many clinicians are concerned about respiratory depression and the risk of death. The overall incidence of respiratory depression with PCAs is less than 1% (range from 0.1 to 0.8%), similar to conventional analgesia. However, the incidence is significantly higher when basal rates are used, rising to 1.1 to 3.9%. Other factors predisposing a patient to increased risk of respiratory depression are older age, obstructive sleep apnea, hypovolemia, renal failure, and the concurrent use of other sedating medications.18
Medication errors are also common. The overall incidence of medication mishaps with PCAs is 1.2%.19 More than 50% of these occur because of operator-related errors (e.g., improper loading, programming errors, and documentation errors). Equipment malfunction is the next most common error.
Opioid equianalgesic dosing conversions: The equianalgesic dose ratio is the ratio of the dose of two opioids required to produce the same analgesic effect. (See Table 1, right.) For example, IV morphine is three times as potent as oral morphine, with an equianalgesic dose ratio of 1:3. Equianalgesic dose tables vary somewhat in their values, which have been largely determined by single-dose administration studies.20 The generalizability of these tables to chronic opioid administration is not well studied.
Incomplete cross tolerance: When switching from one opioid to another, lower doses can be used to control pain.21, 22 Tolerance to one opioid does not completely transfer to the new opioid. Starting at half to two-thirds of the new opioid dose is generally recommended to avoid opioid-specific tolerance and inter-individual variability.23,24
Back to the Case
Opioids are the mainstay of pharmacological management of moderate-to-severe cancer pain. Evaluation of the patient reveals that her acute increase in pain is likely due to progression of her cancer. She had been taking morphine (sustained-release, 90 mg oral) twice daily for her pain and had been using approximately five doses per day of immediate-release oral morphine 20 mg for breakthrough pain. This is equivalent to a total 24-hour opioid requirement of 280 mg oral morphine.
She should be started on a PCA for rapid pain control and titration. Hydromorphone (Dilaudid) is a better PCA choice than morphine because she has acute renal failure. The equianalgesic dose ratio of oral morphine to IV hydromorphone is approximately 30:1.5. The total 24-hour opioid dose of 280 mg oral morphine is equivalent to 14 mg IV hydromorphone ([280mg morphine per day ÷ 30] x 1.5 = 14).
After adjusting for 60% incomplete cross tolerance, the total 24-hour opioid dose is reduced to 8.4 mg IV hydromorphone (14 mg x 0.6 = 8.4 mg). This is approximately equivalent to 0.4 mg IV hydromorphone/hour (8.4 mg ÷ 24 hours), which is her initial basal rate. The demand dose should be set at 0.2 mg (50% the basal rate) with a lockout interval of 10 minutes.
Over a period of several days, the patient’s pain was controlled and her opioid requirements stabilized. She was on a basal rate of 1.4 mg/hour and a demand dose of 1 mg with a 10-minute lockout. Her total 24-hour opioid requirement was 44 mg of IV hydromorphone. As her renal function improved but did not completely normalize, oxycodone was chosen over morphine when converting her back to oral pain medications (less active renal metabolites). The equianalgesic dose ratio of oral oxycodone to IV hydromorphone is approximately 20:1.5. Her total 24-hour opioid dose of 44 mg IV hydromorphone is equivalent to 587 mg oral oxycodone (44 ÷ 1.5) x 20. After adjusting for 60% incomplete cross tolerance, the total 24-hour opioid dose is reduced to 352 mg oral oxycodone or 180 mg of sustained-release oxycodone twice daily (352 mg ÷ 2 ≈ 180 mg). For breakthrough pain she should receive 40 mg of immediate-release oxycodone every hour as needed (10% to 15% of the 24-hour opioid requirement). TH
Dr. Youngwerth is a hospitalist and instructor of medicine, University of Colorado at Denver, assistant director, Palliative Care Consult Service, associate director, Colorado Palliative Medicine Fellowship Program, and medical director, Hospice of Saint John.
References
- American Pain Society. Pain: Current understanding of assessment, management, and treatments. National Pharmaceutical Council 2006;1-79.
- Morrison RS, Meier DE, Fischberg D, et al. Improving the management of pain in hospitalized adults. Arch Intern Med. 2006;166:1033-1039.
- Grass JA. Patient-controlled analgesia. Anesth Analg. 2005;101:S44-S61.
- Etches RC. Patient-controlled analgesia. Surg Clinics N Amer. 1999;79:297-312.
- Nolan MF and Wilson M-C B. Patient-controlled analgesia: A method for the controlled self-administration of opioid pain medications. Phys Ther. 1995;75:374-379.
- Ballantyne JC, Carr DB, Chalmers TC, Dear KBG, Angelillo IF, Mosteller F. Postoperative patient-controlled analgesia: Meta-analyses of initial randomized control trials. J Clin Anesth. 1993;5:182-193.
- Hudcova J, McNicol E, Quah C, Lau J, Carr DB. Patient controlled opioid analgesia versus conventional opioid analgesia for postoperative pain. Cochrane Database of Systematic Reviews. 2006;4:1-10.
- Sidebotham D, Dijkhuizen MRJ, Schug SA. The safety and utilization of patient-controlled analgesia. J Pain Symptom Manage. 1997;14:202-209.
- Macintyre PE. Safety and efficacy of patient-controlled analgesia. Br J Anaesth. 2001;87:36-46.
- Manon C, Rittenhouse BE, Perreault S, et al. Efficacy and costs of patient-controlled analgesia versus regularly administered intramuscular opioid therapy. Amer Soc Anesth Inc. 1998;89:1377-1388.
- Krenn H, Oczenski W, Jellinek H, Krumpl-Ströher M, Schweitzer E, Fitzgerald RD. Nalbuphine by PCA-pump for analgesia following hysterectomy: Bolus application versus continuous infusion with bolus application. Eur J Pain. 2001;5:219-226.
- Lehmann KA. Recent developments in patient-controlled analgesia. J Pain Symptom Manage. 2005;29:S72-S89.
- American Pain Society. Principles of analgesic use in the treatment of acute pain and cancer pain. 5th ed. 2003:1-73.
- Macintyre PC, Jarvis DA. Age is the best predictor of postoperative morphine requirements. Pain. 1995;64:357-364.
- National Comprehensive Cancer Network. Clinical practice guidelines in oncology: Adult cancer pain. Version 2.2005:1-30.
- Ginsberg B, Gil KM, Muir M, Sullivan F, Williams DA, Glass PSA. The influence of lockout intervals and drug selection on patient-controlled analgesia following gynecological surgery. Pain. 1995;62:95-100.
- Walder B, Schafer M, Henzi I, Tramer MR. Efficacy and safety of patient-controlled opioid analgesia for acute postoperative pain. Acta Anaesthesiol Scand. 2001;45:795-804.
- Etches RC. Respiratory depression associated with patient-controlled analgesia: a review of eight cases. Can J Anaesth. 1994;41:125-132.
- Oswalt KE, Shrewsbury P, Stanton-Hicks M. The incidence of medication mishaps in 3,299 PCA patients. Pain. 1990;S5;S152.
- Pereira J, Lawlor P, Vigano A, Dorgan M, Bruera E. Equianalgesic dose rations for opioids: A critical review and proposals for long-term dosing. J Pain Symptom Manage. 2001;22:672-687.
- Ballantyne JC, Mao J. Opioid therapy for chronic pain. N Engl J Med. 2003;349:1943-1953.
- Mercandante S. Opioid rotation for cancer pain. Cancer. 1999;86:1856-1866.
- Mehta V, Langford RM. Acute pain management for opioid dependent patients. Anaesthesia. 2006;61:269-276.
- Pasternak GW. Incomplete cross tolerance and multiple mu opioid peptide receptors. Trends Pharm Sciences. 2001;22:67-70.
In the Literature
Repeat Testing for C. Diff?
By Jeff Glasheen, MD
Mohan SS, McDermott BP, Parchuri S, et al. Lack of value of repeat stool testing for Clostridium difficile toxin. Am J Med. 2006 Apr;119(4):356.e7-356.e8
Clostridium difficile is a common complication of antibiotic and chemotherapeutic use, especially in hospitalized patients. Yet most nosocomial diarrhea is not caused by C. difficile. Most antibiotics can cause loose stools through changes in the gastrointestinal flora that result in inadequate digestion and absorption of carbohydrates and a resultant osmotic diarrhea. Further, antibiotics such as erythromycin and amoxicillin/clavulanate may result in diarrhea via increases in GI tract motility. While osmotic and motility causes of diarrhea tend to improve with antibiotic discontinuation, C. difficile-associated diarrhea is associated with significant morbidity that often continues until adequately treated.
Thus having a test that differentiates between C. difficile and non-clostridial diarrhea is essential. The most commonly used test is the enzyme immunoassay (EIA) that detects toxins A and B. The sensitivity and specificity of this test has been reported to range between 50%-90% and 70%-95%, respectively. The authors of this paper evaluated the utility of repeat EIA testing in patients with a one negative test in the setting of nosocomial diarrhea associated with antibiotic use.
The authors reviewed 474 sequential EIA tests for C. difficile in 396 patients over a 10-month period at a large university-affiliated community hospital with an EIA sensitivity and specificity of 80%-90% and 80%-95%, respectively. Tests were considered to be “repeat” if they occurred within seven days of the original negative test. Of the 78 repeat tests (16.5% of all tests), only one was positive, resulting in a 0.8% conversion rate. At an institutional cost of $128 per test the total cost of EIA testing over the 10-month period was $60,672. The cost of repeat testing alone was $9,984. The authors conclude that there is limited value—and high cost—in repeat EIA testing and that alternative sources of diarrhea should be sought or we should repeat EIA testing in patients with continued nosocomial diarrhea and a negative EIA test.
While prior studies have shown incremental benefit of retesting for C. difficile with the EIA assay, this study’s authors conclude that repeat “C. difficile testing is not clinically justified and is economically wasteful.” Unfortunately, the authors did not utilize a strong enough research design to defend this statement. From the data presented, all we can conclude is that repeat testing with an EIA assay did not add significant value to the diagnostic workup. The lack of a negative gold standard test, such as the cytotoxin assay or follow up outcomes, such as resolution of diarrhea, leaves the reader pondering if the repeat EIA assays were negative because the patients did not have C. difficile or because the test was not sensitive enough to detect the toxin. The reported sensitivity for the EIA assay used was 80-90%, meaning that 10%-20% of patients with C. difficile disease would have had a false negative test. While a second negative test would lower the likelihood of true disease, it would not lower it enough in a patient with a high pre-test probability of disease to sufficiently rule out the disease such that further testing is indicated.
Additionally, while we can extrapolate that the EIA test was of little utility to the patients studied here, no patient-specific data is presented. Thus it is difficult to determine if our patient population is represented in the study. More knowledge about the patients would allow the reader to use published prediction rules to better delineate how likely it was that this cohort was at high risk for having toxin-mediated diarrhea. Perhaps a more reasonable approach to this clinical conundrum would be to send the repeat stool test for a cytotoxin assay or to treat the patient empirically in situations where either the likelihood of disease or the disease burden is high.
Stroke after MI
By Ethan Cumbler, MD
Witt BJ, Ballman KV, Brown RD Jr, et al. The incidence of stroke after myocardial infarction: a meta-analysis. Am J Med. 2006 Apr;119(4):354.e1-9.
Stroke and myocardial infarction (MI) share many of the risk factors leading to atherosclerosis, including hypertension, hyperlipidemia, diabetes, tobacco abuse, and age. Logically, patients at risk for one event would also be at risk for the other, yet this interaction appears to be more complex. The study by Witt, et al., aims to elucidate the rate of in-hospital stroke in patients initially admitted with an MI.
The authors analyzed 22 observational studies of myocardial infarction that recorded the incidence of cerebrovascular accidents after acute MI. Clinical trials were excluded from the analysis in order to provide representation of an unselected population. Of the trials reviewed, 11 were included in the analysis of in-hospital strokes, three for the 30-day time point, and two for the one-year time point. The other trials used different time points and were not included in the analysis. The patients had a mean age ranging between 59 and 72.7 years, and all had a predominance of males. The rate of in-hospital stroke was 11.1 events per 1,000 hospitalizations. This incidence rose to 12.2/1,000 at 30 days and 21.4/1,000 at one year.
Plausible hypotheses for why the rate of cerebrovascular accident would be particularly high in the post-MI period include the potential for localized wall motion dysmotility or low flow leading to intracardiac thrombosis, event-related arrhythmia, or procedure-related embolic events. The studies from which this meta-analysis was derived were not designed in such a way for a causal relationship to be identified. However, age, diabetes, hypertension, prior stroke, anterior location of MI, atrial fibrillation, heart failure, and nonwhite race were all found to have an association with increased risk for stroke. Interestingly, angina on presentation was associated with an apparent decreased risk, theorized to potentially be due to ischemic preconditioning.
While this study shares the usual limitations of meta-analyses of observational studies, the authors have done an excellent summation of the data available including both English and non-English language articles in the analysis. Notably, the review included studies spanning more than 25 years and, thus, included data from studies done in the era prior to modern therapy for cardiac ischemia including potent antiplatelet and statin therapy. The three studies with in-hospital time points started in the 1990s had a lower average rate of stroke, which may reflect the effect of more potent anti-platelet agents used in today’s therapy for acute coronary syndromes.
The implication for the hospitalist is to recognize that patients admitted for MI are at high risk for stroke during the index hospitalization. A low threshold for suspicion of a cerebrovascular event needs to be maintained for post-MI patients with new neurologic symptoms. Future studies will be needed to address the risk/benefit of anticoagulation in high-risk patients for stroke following a myocardial infarction.
Predicting PE in the ED Using Revised Geneva Score
By Jeffrey Carter, MD
Le Gal G, Righini M, Roy PM, et al. Prediction of pulmonary embolism in the emergency department: the revised Geneva score. Ann Intern Med. 2006 Feb 7;144(3):165-171.
Introduction: Pulmonary embolism remains a common life-threatening disorder with imperfect diagnostic modalities and strategies. Much of the current literature focuses on the development and validation of clinical probability assessments that identify low-risk patients who can be safely managed without invasive testing or lung scanning.
Two common scoring systems include the Geneva and Wells criteria, which employ a combination of historical information, clinical and laboratory data to stratify patients into three risk groups.1,2 It has recently been shown that a single negative D-dimer test safely excludes patients with suspected VTE in a large cohort, with a three-month follow-up rate of VTE of 0%.3 Criticism of current clinical probability assessment strategies include the inclusion of subjective criteria or the need for blood gas values.1,2 In this paper, the authors sought to derive and validate a scoring system based on readily available objective clinical data.
Methods: The cohort evaluated in this study consists of the same 965 patients used to prospectively evaluate an emergency department diagnostic strategy of VTE.3 Patients presenting to the emergency ward with complaints of chest pain or shortness of breath were evaluated with a standard diagnostic workup that included a checklist of demographic and historical information, signs and symptoms possibly consistent with VTE, ABG, chest X-ray, and EKG results, as well as the likelihood of another diagnosis. However, the goal was to create a scoring system based on readily available objective data, so ABG, chest X-ray and electrocardiogram results were not evaluated as possible components of the score. Clinical variables associated with pulmonary embolism were evaluated for statistical significance in both a univariate and multivariate model. These variables were then validated both internally and with an external cohort.
Results: The incidence of VTE was 23% (222/965). Ten clinical variables were found to be statistically significantly associated with VTE; these comprise the Revised Geneva Score.
Clinical probability is based on points: 0-3 is low probability; 4-10 is intermediate probability, and >10 is high probability. Low, intermediate, and high clinical probabilities had respective rates of VTE of 8%, 28%, and 74%. These percentages were found in the validation cohort and were similar to those in the derivation cohort.
Discussion: This study focuses on the derivation and validation of a clinical scoring system that can provide a numerical estimate of likelihood of VTE. The diagnostic strategy by which VTE is confirmed or excluded is from derivation cohort and is published elsewhere.3 In that study patients classified as no VTE and not treated had a 90-day risk of VTE of 1%. The nine-point revised Geneva score does indeed provide an accurate assessment of risk of VTE, and can thus help guide clinical decision-making. However it is not clear that the revised Geneva score will help decrease invasive diagnostic tests. In the low-risk group, 8% is too great a risk of a life-threatening illness to forego further diagnostic evaluation. Coupled with a negative ELISA D-dimer, exclusion of these patients is safe, but these patients cannot be safely excluded without risk stratification.3
References
- Wells PS, Anderson DR, Rodger M, et al. Derivation of a simple clinical model to categorize patients’ probability of pulmonary embolism: increasing the model’s utility with the SimpliRED D-dimer. Thromb Haemost. 2000 Mar;83:416-420.
- Wicki J, Perneger TV, Junof AF, et al. Assessing clinical probability of pulmonary embolism in the emergency ward: a simple score. Arch Intern Med. 2001 Jan 8;161(1):92-97.
- Perrier A, Roy PM, Aujesky D, et al. Diagnosing pulmonary embolism in outpatients with clinical assessment, D-dimer measurement, venous ultrasound and helical computed tomography: a multicenter management study. Am J Med. 2004 Mar 1;116(5):291-299.
LMWH for Inpatient Palliative Care
By Jeanie Youngwerth, MD
Noble SI, Nelson A, Turner C, et al. Acceptability of low molecular weight heparin thromboprophylaxis for patients receiving palliative care: qualitative study. BMJ. 2006 Mar 11:332(7541);577-580.ePub 2006 Feb 3.
Venous thromboembolism is a major risk factor for patients with malignancy. VTE may reduce survival time in patients receiving palliative care, with one in seven inpatients with cancer dying from pulmonary embolism. The American College of Chest Physicians recommend low molecular weight heparin (LMWH) in hospitalized patients with cancer as level 1A evidence in their 2004 consensus statement on VTE. There are no thromboprophylaxis guidelines in the United Kingdom. Many physicians view daily injections of LMWH as unnecessary distress for palliative care patients. This study focused on what inpatients with advanced cancer receiving palliative care thought about the effect of thromboprophylaxis on overall quality of life.
This was a qualitative study of 28 inpatients receiving palliative care who had advanced metastatic cancer and who were in a regional cancer center in Wales. The patients had received LMWH for at least five consecutive days. The patients were audiotaped and then had semi-structured interviews transcribed regarding cancer treatments they had received. These interviews covered the patients’ insight into prognosis, their understanding of thromboprophylaxis, their beliefs concerning the effects of thromboprophylaxis on overall quality of life, and the negative aspects of heparin treatment.
The main outcome measures were recurring themes of the effect of thromboprophylaxis on overall quality of life. Major themes identified were insight into prognosis, knowledge and understanding of thromboprophylaxis, acceptability of heparin thromboprophylaxis, reassurance, and optimism. Minor themes identified were bruising, negative impact of antiembolic stockings, and anger at paternalistic views toward terminally ill patients.
Most patients showed clear insight into the nature of their condition and understood heparin prophylaxis for VTE. Many patients identified immobility and surgery as VTE risk factors, with little understanding of cancer as a risk factor. All knew that VTE could cause death, but none were aware of the common symptoms. All patients found LMWH thromboprophylaxis acceptable. Patients believed taking measures to prevent symptoms might improve their quality of life and felt that the medical team had not given up on them.
The only negative experiences of LMWH thromboprophylaxis was bruising. All of the patients who wore antiembolic stockings during previous admissions found them uncomfortable. Patients expressed their need to be involved in decision-making, particularly with respect to withdrawal or non-administration of treatment.
This small, qualitative study showed that, contrary to many physicians’ beliefs, patients in palliative care units believe that LMWH injections are acceptable as thromboprophylaxis, but antiembolic stockings are not. The effect of daily injections had little or no effect on the quality of life, with many patients having positive feelings that things were being done to prevent new problems from occurring.
This study was limited by the small sample size, the qualitative nature that could introduce interpretation bias, and the fact that only patients using LMWH were included. Additionally, the United Kingdom has aggressively educated the public on the risks of VTE associated with long flights such that baseline knowledge may differ in other parts of the world. Resource utilization, including drug costs and length of stay, as well as effect on mortality were not studied.
The implications for hospitalists are that many inpatients with advanced cancer receiving palliative care may find LMWH thromboprophylaxis acceptable therapy, and that discussions with the patient regarding this option should be explored together.
Cost Analysis: Intensive Glycemic Control in Critically Ill Adults
By Whitney Woodmansee, MD
Krinsley JS, Jones, RL. Cost analysis of intensive glycemic control in critically ill adult patients. Chest. 2006;129:644-650.
Several studies have demonstrated improved outcomes and decreased mortality in ICU patients treated with intensive control of blood glucose levels. This study sought to identify the costs associated with intensive glycemic control in the ICU.
An ICU patient database was analyzed for cost data related to intensive glycemic control. A baseline group of 800 consecutive ICU patients admitted prior to initiation (baseline) of an intensive glucose management (blood glucose levels between 80-140 mg/dl protocol were compared with a treatment group of 800 consecutive patients admitted after initiation of the protocol). Previously reported outcomes of these patients demonstrated significant improvement in mortality with tight glycemic control. Costs related to ICU and non-ICU length of stay; duration of mechanical ventilation; and all laboratory, pharmacy, and radiology services were analyzed between groups. Resource utilization was determined by assessing charges from the database and adjusting for inflation and applying Medicare cost, charge ratios for each category, and fiscal year. Costs associated with the intensive glycemic control protocol were determined. Unfortunately, only cost estimates for insulin and associated disposable supplies for each group were available for analysis.
Baseline and treatment populations did not differ significantly regarding demographics such as age, gender, race, admitting diagnosis, diabetes prevalence, or APACHE II scores. There were fewer patients in the treatment group that required mechanical ventilation during their ICU stay (40.6% versus 33.6%). Intensive glucose management was associated with a 13.9% reduction in total ICU days and duration of mechanical ventilation (median of two days decreased to 1.7 days p=0.045). There was a $1,580 adjusted cost savings per patient in the intensive treatment group compared with the baseline group (p<0.001). This reduction in cost was primarily driven by a decrease in laboratory and radiology costs in the ventilated patients. There were nonsignificant cost reductions in the unventilated patients.
Intensive control of hyperglycemia in the hospitalized ICU patient appears to be associated with reduction of morbidity and mortality. This suggests that tight glycemic control also leads to reductions in overall patient care costs—particularly in the ventilated ICU patient. Although not a randomized control trial, database analysis of costs and resource utilization demonstrated an overall cost savings in the treatment group (after initiation of an intensive glycemic control protocol) compared with the baseline group (before protocol initiation). One caveat is that the authors used estimates when determining the costs associated with the implementation of the intensive glucose management protocol. Nevertheless, intensive glycemic control was associated with an overall reduction in patient costs related to decreased ICU days and mechanical ventilation as well as resource utilization in a patient population already shown to have improved mortality. These results, if confirmed, suggest that tight glycemic control in the ICU is cost effective and should become standard medical practice. TH
Repeat Testing for C. Diff?
By Jeff Glasheen, MD
Mohan SS, McDermott BP, Parchuri S, et al. Lack of value of repeat stool testing for Clostridium difficile toxin. Am J Med. 2006 Apr;119(4):356.e7-356.e8
Clostridium difficile is a common complication of antibiotic and chemotherapeutic use, especially in hospitalized patients. Yet most nosocomial diarrhea is not caused by C. difficile. Most antibiotics can cause loose stools through changes in the gastrointestinal flora that result in inadequate digestion and absorption of carbohydrates and a resultant osmotic diarrhea. Further, antibiotics such as erythromycin and amoxicillin/clavulanate may result in diarrhea via increases in GI tract motility. While osmotic and motility causes of diarrhea tend to improve with antibiotic discontinuation, C. difficile-associated diarrhea is associated with significant morbidity that often continues until adequately treated.
Thus having a test that differentiates between C. difficile and non-clostridial diarrhea is essential. The most commonly used test is the enzyme immunoassay (EIA) that detects toxins A and B. The sensitivity and specificity of this test has been reported to range between 50%-90% and 70%-95%, respectively. The authors of this paper evaluated the utility of repeat EIA testing in patients with a one negative test in the setting of nosocomial diarrhea associated with antibiotic use.
The authors reviewed 474 sequential EIA tests for C. difficile in 396 patients over a 10-month period at a large university-affiliated community hospital with an EIA sensitivity and specificity of 80%-90% and 80%-95%, respectively. Tests were considered to be “repeat” if they occurred within seven days of the original negative test. Of the 78 repeat tests (16.5% of all tests), only one was positive, resulting in a 0.8% conversion rate. At an institutional cost of $128 per test the total cost of EIA testing over the 10-month period was $60,672. The cost of repeat testing alone was $9,984. The authors conclude that there is limited value—and high cost—in repeat EIA testing and that alternative sources of diarrhea should be sought or we should repeat EIA testing in patients with continued nosocomial diarrhea and a negative EIA test.
While prior studies have shown incremental benefit of retesting for C. difficile with the EIA assay, this study’s authors conclude that repeat “C. difficile testing is not clinically justified and is economically wasteful.” Unfortunately, the authors did not utilize a strong enough research design to defend this statement. From the data presented, all we can conclude is that repeat testing with an EIA assay did not add significant value to the diagnostic workup. The lack of a negative gold standard test, such as the cytotoxin assay or follow up outcomes, such as resolution of diarrhea, leaves the reader pondering if the repeat EIA assays were negative because the patients did not have C. difficile or because the test was not sensitive enough to detect the toxin. The reported sensitivity for the EIA assay used was 80-90%, meaning that 10%-20% of patients with C. difficile disease would have had a false negative test. While a second negative test would lower the likelihood of true disease, it would not lower it enough in a patient with a high pre-test probability of disease to sufficiently rule out the disease such that further testing is indicated.
Additionally, while we can extrapolate that the EIA test was of little utility to the patients studied here, no patient-specific data is presented. Thus it is difficult to determine if our patient population is represented in the study. More knowledge about the patients would allow the reader to use published prediction rules to better delineate how likely it was that this cohort was at high risk for having toxin-mediated diarrhea. Perhaps a more reasonable approach to this clinical conundrum would be to send the repeat stool test for a cytotoxin assay or to treat the patient empirically in situations where either the likelihood of disease or the disease burden is high.
Stroke after MI
By Ethan Cumbler, MD
Witt BJ, Ballman KV, Brown RD Jr, et al. The incidence of stroke after myocardial infarction: a meta-analysis. Am J Med. 2006 Apr;119(4):354.e1-9.
Stroke and myocardial infarction (MI) share many of the risk factors leading to atherosclerosis, including hypertension, hyperlipidemia, diabetes, tobacco abuse, and age. Logically, patients at risk for one event would also be at risk for the other, yet this interaction appears to be more complex. The study by Witt, et al., aims to elucidate the rate of in-hospital stroke in patients initially admitted with an MI.
The authors analyzed 22 observational studies of myocardial infarction that recorded the incidence of cerebrovascular accidents after acute MI. Clinical trials were excluded from the analysis in order to provide representation of an unselected population. Of the trials reviewed, 11 were included in the analysis of in-hospital strokes, three for the 30-day time point, and two for the one-year time point. The other trials used different time points and were not included in the analysis. The patients had a mean age ranging between 59 and 72.7 years, and all had a predominance of males. The rate of in-hospital stroke was 11.1 events per 1,000 hospitalizations. This incidence rose to 12.2/1,000 at 30 days and 21.4/1,000 at one year.
Plausible hypotheses for why the rate of cerebrovascular accident would be particularly high in the post-MI period include the potential for localized wall motion dysmotility or low flow leading to intracardiac thrombosis, event-related arrhythmia, or procedure-related embolic events. The studies from which this meta-analysis was derived were not designed in such a way for a causal relationship to be identified. However, age, diabetes, hypertension, prior stroke, anterior location of MI, atrial fibrillation, heart failure, and nonwhite race were all found to have an association with increased risk for stroke. Interestingly, angina on presentation was associated with an apparent decreased risk, theorized to potentially be due to ischemic preconditioning.
While this study shares the usual limitations of meta-analyses of observational studies, the authors have done an excellent summation of the data available including both English and non-English language articles in the analysis. Notably, the review included studies spanning more than 25 years and, thus, included data from studies done in the era prior to modern therapy for cardiac ischemia including potent antiplatelet and statin therapy. The three studies with in-hospital time points started in the 1990s had a lower average rate of stroke, which may reflect the effect of more potent anti-platelet agents used in today’s therapy for acute coronary syndromes.
The implication for the hospitalist is to recognize that patients admitted for MI are at high risk for stroke during the index hospitalization. A low threshold for suspicion of a cerebrovascular event needs to be maintained for post-MI patients with new neurologic symptoms. Future studies will be needed to address the risk/benefit of anticoagulation in high-risk patients for stroke following a myocardial infarction.
Predicting PE in the ED Using Revised Geneva Score
By Jeffrey Carter, MD
Le Gal G, Righini M, Roy PM, et al. Prediction of pulmonary embolism in the emergency department: the revised Geneva score. Ann Intern Med. 2006 Feb 7;144(3):165-171.
Introduction: Pulmonary embolism remains a common life-threatening disorder with imperfect diagnostic modalities and strategies. Much of the current literature focuses on the development and validation of clinical probability assessments that identify low-risk patients who can be safely managed without invasive testing or lung scanning.
Two common scoring systems include the Geneva and Wells criteria, which employ a combination of historical information, clinical and laboratory data to stratify patients into three risk groups.1,2 It has recently been shown that a single negative D-dimer test safely excludes patients with suspected VTE in a large cohort, with a three-month follow-up rate of VTE of 0%.3 Criticism of current clinical probability assessment strategies include the inclusion of subjective criteria or the need for blood gas values.1,2 In this paper, the authors sought to derive and validate a scoring system based on readily available objective clinical data.
Methods: The cohort evaluated in this study consists of the same 965 patients used to prospectively evaluate an emergency department diagnostic strategy of VTE.3 Patients presenting to the emergency ward with complaints of chest pain or shortness of breath were evaluated with a standard diagnostic workup that included a checklist of demographic and historical information, signs and symptoms possibly consistent with VTE, ABG, chest X-ray, and EKG results, as well as the likelihood of another diagnosis. However, the goal was to create a scoring system based on readily available objective data, so ABG, chest X-ray and electrocardiogram results were not evaluated as possible components of the score. Clinical variables associated with pulmonary embolism were evaluated for statistical significance in both a univariate and multivariate model. These variables were then validated both internally and with an external cohort.
Results: The incidence of VTE was 23% (222/965). Ten clinical variables were found to be statistically significantly associated with VTE; these comprise the Revised Geneva Score.
Clinical probability is based on points: 0-3 is low probability; 4-10 is intermediate probability, and >10 is high probability. Low, intermediate, and high clinical probabilities had respective rates of VTE of 8%, 28%, and 74%. These percentages were found in the validation cohort and were similar to those in the derivation cohort.
Discussion: This study focuses on the derivation and validation of a clinical scoring system that can provide a numerical estimate of likelihood of VTE. The diagnostic strategy by which VTE is confirmed or excluded is from derivation cohort and is published elsewhere.3 In that study patients classified as no VTE and not treated had a 90-day risk of VTE of 1%. The nine-point revised Geneva score does indeed provide an accurate assessment of risk of VTE, and can thus help guide clinical decision-making. However it is not clear that the revised Geneva score will help decrease invasive diagnostic tests. In the low-risk group, 8% is too great a risk of a life-threatening illness to forego further diagnostic evaluation. Coupled with a negative ELISA D-dimer, exclusion of these patients is safe, but these patients cannot be safely excluded without risk stratification.3
References
- Wells PS, Anderson DR, Rodger M, et al. Derivation of a simple clinical model to categorize patients’ probability of pulmonary embolism: increasing the model’s utility with the SimpliRED D-dimer. Thromb Haemost. 2000 Mar;83:416-420.
- Wicki J, Perneger TV, Junof AF, et al. Assessing clinical probability of pulmonary embolism in the emergency ward: a simple score. Arch Intern Med. 2001 Jan 8;161(1):92-97.
- Perrier A, Roy PM, Aujesky D, et al. Diagnosing pulmonary embolism in outpatients with clinical assessment, D-dimer measurement, venous ultrasound and helical computed tomography: a multicenter management study. Am J Med. 2004 Mar 1;116(5):291-299.
LMWH for Inpatient Palliative Care
By Jeanie Youngwerth, MD
Noble SI, Nelson A, Turner C, et al. Acceptability of low molecular weight heparin thromboprophylaxis for patients receiving palliative care: qualitative study. BMJ. 2006 Mar 11:332(7541);577-580.ePub 2006 Feb 3.
Venous thromboembolism is a major risk factor for patients with malignancy. VTE may reduce survival time in patients receiving palliative care, with one in seven inpatients with cancer dying from pulmonary embolism. The American College of Chest Physicians recommend low molecular weight heparin (LMWH) in hospitalized patients with cancer as level 1A evidence in their 2004 consensus statement on VTE. There are no thromboprophylaxis guidelines in the United Kingdom. Many physicians view daily injections of LMWH as unnecessary distress for palliative care patients. This study focused on what inpatients with advanced cancer receiving palliative care thought about the effect of thromboprophylaxis on overall quality of life.
This was a qualitative study of 28 inpatients receiving palliative care who had advanced metastatic cancer and who were in a regional cancer center in Wales. The patients had received LMWH for at least five consecutive days. The patients were audiotaped and then had semi-structured interviews transcribed regarding cancer treatments they had received. These interviews covered the patients’ insight into prognosis, their understanding of thromboprophylaxis, their beliefs concerning the effects of thromboprophylaxis on overall quality of life, and the negative aspects of heparin treatment.
The main outcome measures were recurring themes of the effect of thromboprophylaxis on overall quality of life. Major themes identified were insight into prognosis, knowledge and understanding of thromboprophylaxis, acceptability of heparin thromboprophylaxis, reassurance, and optimism. Minor themes identified were bruising, negative impact of antiembolic stockings, and anger at paternalistic views toward terminally ill patients.
Most patients showed clear insight into the nature of their condition and understood heparin prophylaxis for VTE. Many patients identified immobility and surgery as VTE risk factors, with little understanding of cancer as a risk factor. All knew that VTE could cause death, but none were aware of the common symptoms. All patients found LMWH thromboprophylaxis acceptable. Patients believed taking measures to prevent symptoms might improve their quality of life and felt that the medical team had not given up on them.
The only negative experiences of LMWH thromboprophylaxis was bruising. All of the patients who wore antiembolic stockings during previous admissions found them uncomfortable. Patients expressed their need to be involved in decision-making, particularly with respect to withdrawal or non-administration of treatment.
This small, qualitative study showed that, contrary to many physicians’ beliefs, patients in palliative care units believe that LMWH injections are acceptable as thromboprophylaxis, but antiembolic stockings are not. The effect of daily injections had little or no effect on the quality of life, with many patients having positive feelings that things were being done to prevent new problems from occurring.
This study was limited by the small sample size, the qualitative nature that could introduce interpretation bias, and the fact that only patients using LMWH were included. Additionally, the United Kingdom has aggressively educated the public on the risks of VTE associated with long flights such that baseline knowledge may differ in other parts of the world. Resource utilization, including drug costs and length of stay, as well as effect on mortality were not studied.
The implications for hospitalists are that many inpatients with advanced cancer receiving palliative care may find LMWH thromboprophylaxis acceptable therapy, and that discussions with the patient regarding this option should be explored together.
Cost Analysis: Intensive Glycemic Control in Critically Ill Adults
By Whitney Woodmansee, MD
Krinsley JS, Jones, RL. Cost analysis of intensive glycemic control in critically ill adult patients. Chest. 2006;129:644-650.
Several studies have demonstrated improved outcomes and decreased mortality in ICU patients treated with intensive control of blood glucose levels. This study sought to identify the costs associated with intensive glycemic control in the ICU.
An ICU patient database was analyzed for cost data related to intensive glycemic control. A baseline group of 800 consecutive ICU patients admitted prior to initiation (baseline) of an intensive glucose management (blood glucose levels between 80-140 mg/dl protocol were compared with a treatment group of 800 consecutive patients admitted after initiation of the protocol). Previously reported outcomes of these patients demonstrated significant improvement in mortality with tight glycemic control. Costs related to ICU and non-ICU length of stay; duration of mechanical ventilation; and all laboratory, pharmacy, and radiology services were analyzed between groups. Resource utilization was determined by assessing charges from the database and adjusting for inflation and applying Medicare cost, charge ratios for each category, and fiscal year. Costs associated with the intensive glycemic control protocol were determined. Unfortunately, only cost estimates for insulin and associated disposable supplies for each group were available for analysis.
Baseline and treatment populations did not differ significantly regarding demographics such as age, gender, race, admitting diagnosis, diabetes prevalence, or APACHE II scores. There were fewer patients in the treatment group that required mechanical ventilation during their ICU stay (40.6% versus 33.6%). Intensive glucose management was associated with a 13.9% reduction in total ICU days and duration of mechanical ventilation (median of two days decreased to 1.7 days p=0.045). There was a $1,580 adjusted cost savings per patient in the intensive treatment group compared with the baseline group (p<0.001). This reduction in cost was primarily driven by a decrease in laboratory and radiology costs in the ventilated patients. There were nonsignificant cost reductions in the unventilated patients.
Intensive control of hyperglycemia in the hospitalized ICU patient appears to be associated with reduction of morbidity and mortality. This suggests that tight glycemic control also leads to reductions in overall patient care costs—particularly in the ventilated ICU patient. Although not a randomized control trial, database analysis of costs and resource utilization demonstrated an overall cost savings in the treatment group (after initiation of an intensive glycemic control protocol) compared with the baseline group (before protocol initiation). One caveat is that the authors used estimates when determining the costs associated with the implementation of the intensive glucose management protocol. Nevertheless, intensive glycemic control was associated with an overall reduction in patient costs related to decreased ICU days and mechanical ventilation as well as resource utilization in a patient population already shown to have improved mortality. These results, if confirmed, suggest that tight glycemic control in the ICU is cost effective and should become standard medical practice. TH
Repeat Testing for C. Diff?
By Jeff Glasheen, MD
Mohan SS, McDermott BP, Parchuri S, et al. Lack of value of repeat stool testing for Clostridium difficile toxin. Am J Med. 2006 Apr;119(4):356.e7-356.e8
Clostridium difficile is a common complication of antibiotic and chemotherapeutic use, especially in hospitalized patients. Yet most nosocomial diarrhea is not caused by C. difficile. Most antibiotics can cause loose stools through changes in the gastrointestinal flora that result in inadequate digestion and absorption of carbohydrates and a resultant osmotic diarrhea. Further, antibiotics such as erythromycin and amoxicillin/clavulanate may result in diarrhea via increases in GI tract motility. While osmotic and motility causes of diarrhea tend to improve with antibiotic discontinuation, C. difficile-associated diarrhea is associated with significant morbidity that often continues until adequately treated.
Thus having a test that differentiates between C. difficile and non-clostridial diarrhea is essential. The most commonly used test is the enzyme immunoassay (EIA) that detects toxins A and B. The sensitivity and specificity of this test has been reported to range between 50%-90% and 70%-95%, respectively. The authors of this paper evaluated the utility of repeat EIA testing in patients with a one negative test in the setting of nosocomial diarrhea associated with antibiotic use.
The authors reviewed 474 sequential EIA tests for C. difficile in 396 patients over a 10-month period at a large university-affiliated community hospital with an EIA sensitivity and specificity of 80%-90% and 80%-95%, respectively. Tests were considered to be “repeat” if they occurred within seven days of the original negative test. Of the 78 repeat tests (16.5% of all tests), only one was positive, resulting in a 0.8% conversion rate. At an institutional cost of $128 per test the total cost of EIA testing over the 10-month period was $60,672. The cost of repeat testing alone was $9,984. The authors conclude that there is limited value—and high cost—in repeat EIA testing and that alternative sources of diarrhea should be sought or we should repeat EIA testing in patients with continued nosocomial diarrhea and a negative EIA test.
While prior studies have shown incremental benefit of retesting for C. difficile with the EIA assay, this study’s authors conclude that repeat “C. difficile testing is not clinically justified and is economically wasteful.” Unfortunately, the authors did not utilize a strong enough research design to defend this statement. From the data presented, all we can conclude is that repeat testing with an EIA assay did not add significant value to the diagnostic workup. The lack of a negative gold standard test, such as the cytotoxin assay or follow up outcomes, such as resolution of diarrhea, leaves the reader pondering if the repeat EIA assays were negative because the patients did not have C. difficile or because the test was not sensitive enough to detect the toxin. The reported sensitivity for the EIA assay used was 80-90%, meaning that 10%-20% of patients with C. difficile disease would have had a false negative test. While a second negative test would lower the likelihood of true disease, it would not lower it enough in a patient with a high pre-test probability of disease to sufficiently rule out the disease such that further testing is indicated.
Additionally, while we can extrapolate that the EIA test was of little utility to the patients studied here, no patient-specific data is presented. Thus it is difficult to determine if our patient population is represented in the study. More knowledge about the patients would allow the reader to use published prediction rules to better delineate how likely it was that this cohort was at high risk for having toxin-mediated diarrhea. Perhaps a more reasonable approach to this clinical conundrum would be to send the repeat stool test for a cytotoxin assay or to treat the patient empirically in situations where either the likelihood of disease or the disease burden is high.
Stroke after MI
By Ethan Cumbler, MD
Witt BJ, Ballman KV, Brown RD Jr, et al. The incidence of stroke after myocardial infarction: a meta-analysis. Am J Med. 2006 Apr;119(4):354.e1-9.
Stroke and myocardial infarction (MI) share many of the risk factors leading to atherosclerosis, including hypertension, hyperlipidemia, diabetes, tobacco abuse, and age. Logically, patients at risk for one event would also be at risk for the other, yet this interaction appears to be more complex. The study by Witt, et al., aims to elucidate the rate of in-hospital stroke in patients initially admitted with an MI.
The authors analyzed 22 observational studies of myocardial infarction that recorded the incidence of cerebrovascular accidents after acute MI. Clinical trials were excluded from the analysis in order to provide representation of an unselected population. Of the trials reviewed, 11 were included in the analysis of in-hospital strokes, three for the 30-day time point, and two for the one-year time point. The other trials used different time points and were not included in the analysis. The patients had a mean age ranging between 59 and 72.7 years, and all had a predominance of males. The rate of in-hospital stroke was 11.1 events per 1,000 hospitalizations. This incidence rose to 12.2/1,000 at 30 days and 21.4/1,000 at one year.
Plausible hypotheses for why the rate of cerebrovascular accident would be particularly high in the post-MI period include the potential for localized wall motion dysmotility or low flow leading to intracardiac thrombosis, event-related arrhythmia, or procedure-related embolic events. The studies from which this meta-analysis was derived were not designed in such a way for a causal relationship to be identified. However, age, diabetes, hypertension, prior stroke, anterior location of MI, atrial fibrillation, heart failure, and nonwhite race were all found to have an association with increased risk for stroke. Interestingly, angina on presentation was associated with an apparent decreased risk, theorized to potentially be due to ischemic preconditioning.
While this study shares the usual limitations of meta-analyses of observational studies, the authors have done an excellent summation of the data available including both English and non-English language articles in the analysis. Notably, the review included studies spanning more than 25 years and, thus, included data from studies done in the era prior to modern therapy for cardiac ischemia including potent antiplatelet and statin therapy. The three studies with in-hospital time points started in the 1990s had a lower average rate of stroke, which may reflect the effect of more potent anti-platelet agents used in today’s therapy for acute coronary syndromes.
The implication for the hospitalist is to recognize that patients admitted for MI are at high risk for stroke during the index hospitalization. A low threshold for suspicion of a cerebrovascular event needs to be maintained for post-MI patients with new neurologic symptoms. Future studies will be needed to address the risk/benefit of anticoagulation in high-risk patients for stroke following a myocardial infarction.
Predicting PE in the ED Using Revised Geneva Score
By Jeffrey Carter, MD
Le Gal G, Righini M, Roy PM, et al. Prediction of pulmonary embolism in the emergency department: the revised Geneva score. Ann Intern Med. 2006 Feb 7;144(3):165-171.
Introduction: Pulmonary embolism remains a common life-threatening disorder with imperfect diagnostic modalities and strategies. Much of the current literature focuses on the development and validation of clinical probability assessments that identify low-risk patients who can be safely managed without invasive testing or lung scanning.
Two common scoring systems include the Geneva and Wells criteria, which employ a combination of historical information, clinical and laboratory data to stratify patients into three risk groups.1,2 It has recently been shown that a single negative D-dimer test safely excludes patients with suspected VTE in a large cohort, with a three-month follow-up rate of VTE of 0%.3 Criticism of current clinical probability assessment strategies include the inclusion of subjective criteria or the need for blood gas values.1,2 In this paper, the authors sought to derive and validate a scoring system based on readily available objective clinical data.
Methods: The cohort evaluated in this study consists of the same 965 patients used to prospectively evaluate an emergency department diagnostic strategy of VTE.3 Patients presenting to the emergency ward with complaints of chest pain or shortness of breath were evaluated with a standard diagnostic workup that included a checklist of demographic and historical information, signs and symptoms possibly consistent with VTE, ABG, chest X-ray, and EKG results, as well as the likelihood of another diagnosis. However, the goal was to create a scoring system based on readily available objective data, so ABG, chest X-ray and electrocardiogram results were not evaluated as possible components of the score. Clinical variables associated with pulmonary embolism were evaluated for statistical significance in both a univariate and multivariate model. These variables were then validated both internally and with an external cohort.
Results: The incidence of VTE was 23% (222/965). Ten clinical variables were found to be statistically significantly associated with VTE; these comprise the Revised Geneva Score.
Clinical probability is based on points: 0-3 is low probability; 4-10 is intermediate probability, and >10 is high probability. Low, intermediate, and high clinical probabilities had respective rates of VTE of 8%, 28%, and 74%. These percentages were found in the validation cohort and were similar to those in the derivation cohort.
Discussion: This study focuses on the derivation and validation of a clinical scoring system that can provide a numerical estimate of likelihood of VTE. The diagnostic strategy by which VTE is confirmed or excluded is from derivation cohort and is published elsewhere.3 In that study patients classified as no VTE and not treated had a 90-day risk of VTE of 1%. The nine-point revised Geneva score does indeed provide an accurate assessment of risk of VTE, and can thus help guide clinical decision-making. However it is not clear that the revised Geneva score will help decrease invasive diagnostic tests. In the low-risk group, 8% is too great a risk of a life-threatening illness to forego further diagnostic evaluation. Coupled with a negative ELISA D-dimer, exclusion of these patients is safe, but these patients cannot be safely excluded without risk stratification.3
References
- Wells PS, Anderson DR, Rodger M, et al. Derivation of a simple clinical model to categorize patients’ probability of pulmonary embolism: increasing the model’s utility with the SimpliRED D-dimer. Thromb Haemost. 2000 Mar;83:416-420.
- Wicki J, Perneger TV, Junof AF, et al. Assessing clinical probability of pulmonary embolism in the emergency ward: a simple score. Arch Intern Med. 2001 Jan 8;161(1):92-97.
- Perrier A, Roy PM, Aujesky D, et al. Diagnosing pulmonary embolism in outpatients with clinical assessment, D-dimer measurement, venous ultrasound and helical computed tomography: a multicenter management study. Am J Med. 2004 Mar 1;116(5):291-299.
LMWH for Inpatient Palliative Care
By Jeanie Youngwerth, MD
Noble SI, Nelson A, Turner C, et al. Acceptability of low molecular weight heparin thromboprophylaxis for patients receiving palliative care: qualitative study. BMJ. 2006 Mar 11:332(7541);577-580.ePub 2006 Feb 3.
Venous thromboembolism is a major risk factor for patients with malignancy. VTE may reduce survival time in patients receiving palliative care, with one in seven inpatients with cancer dying from pulmonary embolism. The American College of Chest Physicians recommend low molecular weight heparin (LMWH) in hospitalized patients with cancer as level 1A evidence in their 2004 consensus statement on VTE. There are no thromboprophylaxis guidelines in the United Kingdom. Many physicians view daily injections of LMWH as unnecessary distress for palliative care patients. This study focused on what inpatients with advanced cancer receiving palliative care thought about the effect of thromboprophylaxis on overall quality of life.
This was a qualitative study of 28 inpatients receiving palliative care who had advanced metastatic cancer and who were in a regional cancer center in Wales. The patients had received LMWH for at least five consecutive days. The patients were audiotaped and then had semi-structured interviews transcribed regarding cancer treatments they had received. These interviews covered the patients’ insight into prognosis, their understanding of thromboprophylaxis, their beliefs concerning the effects of thromboprophylaxis on overall quality of life, and the negative aspects of heparin treatment.
The main outcome measures were recurring themes of the effect of thromboprophylaxis on overall quality of life. Major themes identified were insight into prognosis, knowledge and understanding of thromboprophylaxis, acceptability of heparin thromboprophylaxis, reassurance, and optimism. Minor themes identified were bruising, negative impact of antiembolic stockings, and anger at paternalistic views toward terminally ill patients.
Most patients showed clear insight into the nature of their condition and understood heparin prophylaxis for VTE. Many patients identified immobility and surgery as VTE risk factors, with little understanding of cancer as a risk factor. All knew that VTE could cause death, but none were aware of the common symptoms. All patients found LMWH thromboprophylaxis acceptable. Patients believed taking measures to prevent symptoms might improve their quality of life and felt that the medical team had not given up on them.
The only negative experiences of LMWH thromboprophylaxis was bruising. All of the patients who wore antiembolic stockings during previous admissions found them uncomfortable. Patients expressed their need to be involved in decision-making, particularly with respect to withdrawal or non-administration of treatment.
This small, qualitative study showed that, contrary to many physicians’ beliefs, patients in palliative care units believe that LMWH injections are acceptable as thromboprophylaxis, but antiembolic stockings are not. The effect of daily injections had little or no effect on the quality of life, with many patients having positive feelings that things were being done to prevent new problems from occurring.
This study was limited by the small sample size, the qualitative nature that could introduce interpretation bias, and the fact that only patients using LMWH were included. Additionally, the United Kingdom has aggressively educated the public on the risks of VTE associated with long flights such that baseline knowledge may differ in other parts of the world. Resource utilization, including drug costs and length of stay, as well as effect on mortality were not studied.
The implications for hospitalists are that many inpatients with advanced cancer receiving palliative care may find LMWH thromboprophylaxis acceptable therapy, and that discussions with the patient regarding this option should be explored together.
Cost Analysis: Intensive Glycemic Control in Critically Ill Adults
By Whitney Woodmansee, MD
Krinsley JS, Jones, RL. Cost analysis of intensive glycemic control in critically ill adult patients. Chest. 2006;129:644-650.
Several studies have demonstrated improved outcomes and decreased mortality in ICU patients treated with intensive control of blood glucose levels. This study sought to identify the costs associated with intensive glycemic control in the ICU.
An ICU patient database was analyzed for cost data related to intensive glycemic control. A baseline group of 800 consecutive ICU patients admitted prior to initiation (baseline) of an intensive glucose management (blood glucose levels between 80-140 mg/dl protocol were compared with a treatment group of 800 consecutive patients admitted after initiation of the protocol). Previously reported outcomes of these patients demonstrated significant improvement in mortality with tight glycemic control. Costs related to ICU and non-ICU length of stay; duration of mechanical ventilation; and all laboratory, pharmacy, and radiology services were analyzed between groups. Resource utilization was determined by assessing charges from the database and adjusting for inflation and applying Medicare cost, charge ratios for each category, and fiscal year. Costs associated with the intensive glycemic control protocol were determined. Unfortunately, only cost estimates for insulin and associated disposable supplies for each group were available for analysis.
Baseline and treatment populations did not differ significantly regarding demographics such as age, gender, race, admitting diagnosis, diabetes prevalence, or APACHE II scores. There were fewer patients in the treatment group that required mechanical ventilation during their ICU stay (40.6% versus 33.6%). Intensive glucose management was associated with a 13.9% reduction in total ICU days and duration of mechanical ventilation (median of two days decreased to 1.7 days p=0.045). There was a $1,580 adjusted cost savings per patient in the intensive treatment group compared with the baseline group (p<0.001). This reduction in cost was primarily driven by a decrease in laboratory and radiology costs in the ventilated patients. There were nonsignificant cost reductions in the unventilated patients.
Intensive control of hyperglycemia in the hospitalized ICU patient appears to be associated with reduction of morbidity and mortality. This suggests that tight glycemic control also leads to reductions in overall patient care costs—particularly in the ventilated ICU patient. Although not a randomized control trial, database analysis of costs and resource utilization demonstrated an overall cost savings in the treatment group (after initiation of an intensive glycemic control protocol) compared with the baseline group (before protocol initiation). One caveat is that the authors used estimates when determining the costs associated with the implementation of the intensive glucose management protocol. Nevertheless, intensive glycemic control was associated with an overall reduction in patient costs related to decreased ICU days and mechanical ventilation as well as resource utilization in a patient population already shown to have improved mortality. These results, if confirmed, suggest that tight glycemic control in the ICU is cost effective and should become standard medical practice. TH