User login
Early Death or Hospital Readmission
Socioeconomic status (SES) classifies people according to occupation, prior education, or income.[1] Socioeconomic status has been associated with several population‐health outcomes, albeit with geographically inconsistent results.[2] If lower SES is associated with higher readmission rates, then further studies could be done to determine which specific socioeconomic factors are potentially modifiable and whether the provision of additional resources could allay the increased risk associated with those factors.
Nine studies have examined the association between SES and readmissions.[3, 4, 5, 6, 7, 8, 9, 10, 11] These studies varied extensively in methodologies, SES measures, and results. However, results from 1 of these studies[11] were particularly notable given the study's significant association between lower household income and increased risk of acute readmission in a publicly funded, open‐access healthcare system. Given the implications of these results, an accurate and explicit assessment of the association between SES measures and the risk of adverse postdischarge outcomes is important.
We recently developed a model that accurately predicts the risk of 30‐day death or urgent readmission using administrative data.[12] This model did not directly control for any SES factors. In this study, we determined if a commonly used SES measurehousehold‐income quintilewas associated with the risk of early death or urgent readmission after controlling for factors known to influence this outcome.
METHODS
Study Setting and Data Sources
This population‐based study took place in Ontario, Canada, between April 1, 2003 and March 31, 2009. All hospital and physician care in Ontario is publicly funded. The study used 2 databases, the Discharge Abstract Database and the Registered Persons Database. The Discharge Abstract Database records information about all nonpsychiatric hospitalizations, including dates of hospital admission and discharge, vital status at end of hospitalization, discharge destination (ie, community, nursing home, or chronic hospital), admission urgency, primary and other diagnoses, and postal code of patient's household. The Registered Persons Database captures basic demographic data about all Ontarians, including date of birth and date of death (if applicable), postal code of residence, and average household‐income quintile of postal code, determined by linking the postal code to Statistics Canada geographical units through the Postal Code Conversion File Plus.[13] The Registered Persons Database captures all deaths regardless of the death location (ie, community vs hospital).
Study Population
This study used patients from a previous analysis that internally validated an index to predict the risk of 30‐day death or urgent readmission.[12] This analysis included a simple random sample of 250,000 adult Ontarians (age >18 years) who were discharged from the hospital to the community between April 1, 2003 and March 31, 2009. These medical and surgical hospitalizations were sampled from the Discharge Abstract Database described above. Psychiatric admissions were excluded because their hospitalizations are captured in a distinct database; obstetrical admissions were also excluded because they have a very low risk of 30‐day death or readmission. We randomly chose 1 index admission per person to ensure that the patient was the unit of analysis.
For the present study, we selected all patients from the previous analysis who were discharged from the hospital in 2006. This year was chosen because the SES indicator we used in the study (average household‐income quintile) was measured during the 2006 Canadian Census and would be most accurate for patients discharged in that year. The present study also limited patients to those with a valid postal code, because this was required to link patients to their neighborhood and their household‐income quintile.
Study Outcome
The study outcome was all‐cause death or urgent readmission within 30 days of discharge from hospital. We combined death with urgent readmission to avoid potential biases that could occur when measuring associations between risk factors and urgent readmission; in analyses having readmission as the sole outcome, the categorization of early deaths that occur prior to readmission as nonevents could minimize the importance of factors (such as severe comorbidities or patient age) that are associated with both early death and readmission.
We linked to the Registered Patients Database to determine each person's 30‐day death status. We linked to the Discharge Abstract Database to determine if patients had been urgently readmitted to any hospital within 30 days of discharge. All deaths were considered regardless of cause. All urgent (ie, nonscheduled) readmissions were included regardless of the reason for admission. Urgent status was determined by the urgency field in the Discharge Abstract Database, for which data abstractors are instructed to classify all nonscheduled admissions as urgent; these admissions frequently include those admitted after presenting to the emergency department.
Study Covariates: Readmission Risk and Neighborhood Household‐Income Quintile
In our primary analysis, we quantified the risk of 30‐day death or urgent readmission using an internally validated index, the LACE+ index: length of stay (L), acuity of the admission (A), comorbidity of the patient (measured with the Charlson Comorbidity Index score (C), and emergency‐department use (E).[12] The LACE+ index predicts the risk of 30‐day all‐cause death or urgent readmission for nonpsychiatric and nonobstetrical admissions. This index includes patient age, sex, comorbidities, and previous hospital and emergency‐department utilization; admission urgency; hospital type; total length of stay (LOS) and days in hospital awaiting placement; and hospitalization diagnostic risk.[14] The index quantified outcome risk as a score that ranged from 17 to 114. It was very discriminatory (C statistic, 77.1%) and was well calibrated (the observed and expected outcome risk was statistically distinct in only 2 of 14 risk groups that contained <2% of the population). The LACE+ quintiles were defined using score distribution from the entire 20032009 cohort.[12]
We used neighborhood income quintile as 1 measure of patient SES. Neighborhood income quintile was calculated by Statistics Canada using the Income Per Person Equivalent (IPPE) determined from the 2006 Canadian census.[13] The IPPE was calculated as total household income divided by the Single Persons Equivalent, which reflects decreased costs per person (and therefore increased available income per household occupant) in households having greater numbers of people. Within each dissemination area (each contains 400700 people), the average IPPE was calculated. Then, within each region (delineated by the Census Metropolitan Area, the Census Agglomeration, or provincial residual areas), dissemination areas were ranked by their average IPPE and then categorized into quintiles. These household‐income quintiles, therefore, are community‐specific and ensure that neighborhood household incomes are categorized based on comparisons within the same community. As such, the income thresholds for quintile categorization will vary between regions. We linked each patient's postal code to their dissemination area using the Postal Code Conversion File Plus[13] to determine their neighborhood income quintile.
Analysis
We described the patient cohort by readmission status. We categorized the expected risk of 30‐day death or urgent readmission to hospital (as determined by the LACE+ score) into quintiles. We used the 2 test and the test for trend to determine the association of these risk quintiles and SES quintiles with observed rates of 30‐day death or urgent readmission. The Cochran‐Mantel‐Haenszel test was used to determine the association of household‐income quintile and outcome risk after adjusting for LACE+ quintile.
To determine how the association between income quintile and outcome changes with increase adjustment, we constructed a series of logistic‐regression models that contained household‐income quintile and the sequential addition of components of the LACE+ score. For each model, we measured the influence of these added covariates on the association between household‐income quintile and early death or urgent readmission. We used orthogonal parameterization (which facilitates the comparison of parameter estimates in a regression model) to measure linear trends in the association of the income quintiles with outcomes.
RESULTS
The original cohort contained 250,000 people, of which 40,827 people (16.3%) were included in the present study (208,995 were excluded because patients were discharged in years other than 2006; 178 were excluded because of invalid postal codes).
Patients are described in Table 1. Patients were middle‐aged and had few documented chronic comorbidities. Of the patients, 37% had been to the emergency department and 12% had been admitted urgently. Most admissions were to large, nonteaching hospitals with a median LOS of 3 days.
Variable | Value | No Death/Readmission, n=38,189 | Death/Readmission, n=2,638 | Overall, N=40,827 | |||
---|---|---|---|---|---|---|---|
| |||||||
Mean age (SD), y | 57.39 (18.3) | 67.17 (17.2) | 58.02 (18.4) | ||||
Female sex | 20,044 | 52.5% | 1,291 | 48.9% | 21,335 | 52.3% | |
Charlson index | 0 | 28,908 | 75.7% | 1,238 | 46.9% | 30,146 | 73.8% |
1 | 450 | 11.7% | 362 | 13.7% | 4,812 | 11.8% | |
2 | 2,668 | 7.0% | 427 | 16.2% | 3,095 | 7.6% | |
3+ | 2,163 | 5.7% | 611 | 23.2% | 2,774 | 6.8% | |
ED visits in previous 6 moths | 0 | 24,599 | 64.4% | 1,210 | 45.9% | 25,809 | 63.2% |
12 | 11,262 | 29.5% | 1,008 | 38.2% | 12,270 | 30.1% | |
3+ | 2,328 | 6.1% | 420 | 15.9% | 2,748 | 6.7% | |
Urgent hospitalizations, previous year | 0 | 33,729 | 88.3% | 1,796 | 68.1% | 35,525 | 87.0% |
1 | 3,425 | 9.0% | 525 | 19.9% | 3,950 | 9.7% | |
1+ | 1,035 | 2.7% | 317 | 12.0% | 1,352 | 3.3% | |
Elective hospitalizations, previous year | 0 | 35,988 | 94.2% | 2,389 | 90.6% | 38,377 | 94.0% |
1 | 1,998 | 5.2% | 213 | 8.1% | 2,211 | 5.4% | |
2+ | 203 | 0.5% | 36 | 1.4% | 239 | 0.6% | |
Hospital type | Nonteaching, large | 20,554 | 53.8% | 1,334 | 50.6% | 21,888 | 53.6% |
Nonteaching, small | 5,239 | 13.7% | 487 | 18.5% | 5726 | 14.0% | |
Teaching | 12,396 | 32.5% | 817 | 31.0% | 13,213 | 32.4% | |
Urgent admit | 23,769 | 62.2% | 2,223 | 84.3% | 25,992 | 63.7% | |
LOS rounded to nearest day, median (IQR) | 3 (26) | 5 (311) | 3 (26) | ||||
Any hospital days on ALC | 0 | 646 | 1.7% | 127 | 4.8% | 773 | 1.9% |
CMG score of index admission | 0 | 27,257 | 71.4% | 1,594 | 60.4% | 28,851 | 70.7% |
1+ | 5,218 | 13.7% | 948 | 35.9% | 6,166 | 15.1% | |
<0 | 5,714 | 15.0% | 96 | 3.6% | 5,810 | 14.2% | |
LACE+ score of index admission, median (IQR) | 31 (1848) | 61 (4175) | 32 (1951) | ||||
Household‐income quintile | 1 (poorest) | 7,798 | 20.4% | 621 | 23.5% | 8,419 | 20.6% |
2 | 7,812 | 20.5% | 586 | 22.2% | 8,398 | 20.6% | |
3 | 7,557 | 19.8% | 484 | 18.3% | 8,041 | 19.7% | |
4 | 7,561 | 19.8% | 500 | 19.0% | 8,061 | 19.7% | |
5 (richest) | 7,461 | 19.5% | 447 | 16.9% | 7,908 | 19.4% |
Death or urgent readmission within 30 days occurred in 2638 people (6.5%) (Table 1). Outcome risk increased with age; in males; as comorbidities increased; with greater numbers of emergency‐department visits, urgent admissions, and previous elective admissions; when index admissions were emergent; with longer hospital LOS and increased number of alternate level of care days; and as the diagnostic risk (measured as the Case Mix Group [CMG] score)[14] increased. Outcome risk increased as income quintile became poorer.
Household Income and Risk of 30‐Day Death or Urgent Readmission
People were evenly divided among the income quintiles (Table 2). By itself, household‐income quintile was significantly associated with the risk of early death or urgent hospital readmission (Table 2, column C, 2=27.4, P<0.0001; Mantel‐Haenszel trend 2=24.3, P<0.0001). In the poorest quintile, 7.4% of people had an outcome, compared with 5.6% in the richest quintile (2=19.8, df=1, P<0.0001).
Risk Quintile of 30‐Day Death or Readmission (LACE+ Points Range) | ||||||
---|---|---|---|---|---|---|
1 (1416) [A] | 2 (1727) | 3 (2839) | 4 (4056) | 5 (57114) [B] | Income Quintile Overall [C] | |
| ||||||
Income quintile | ||||||
1 (poorest) | 18/1,485 (1.2%) | 42/1,667 (2.5%) | 65/1,635 (4.0%) | 117/1,722 (6.8%) | 379/1,910 (19.8%) | 621/8,419 (7.4%) |
2 | 21/1,627 (1.3%) | 39/1,665 (2.3%) | 65/1,598 (4.1%) | 130/1,808 (5.2%) | 331/1,700 (19.5%) | 586/8,398 (7.0%) |
3 | 18/1,761 (1.0%) | 33/1,665 (2.0%) | 63/1,568 (4.0%) | 96/1,499 (6.4%) | 274/1,548 (17.7%) | 484/8,041 (6.0%) |
4 | 27/1,851 (1.5%) | 42/1,698 (2.4%) | 57/1,585 (3.6%) | 110/1,548 (6.1%) | 264/1,379 (19.1%) | 500/8,061 (6.2%) |
5 (richest) | 20/1,864 (1.1%) | 32/1,736 (1.8%) | 60/1,468 (4.1%) | 107/1,525 (7.0%) | 228/1,315 (17.3%) | 447/7,908 (5.6%) |
Risk quintile overall [D] | 104/8,588 (1.2%) | 188/8,431 (2.2%) | 310/7,854 (4.0%) | 560/8,102 (6.9%) | 1476/7,852 (18.8%) | 2,638/40,827 (6.5%) |
However, household income was also strongly associated with LACE+ scores (2=240, P<0.0001; Mantel‐Haenszel trend 2=209, P<0.0001). The number of people in the lowest‐risk quintile increased with income, from 1485 in the poorest quintile to 1864 in the richest quintile (Table 2, column A). In contrast, the number of high‐risk people progressively decreased with income, from 1910 in the poorest quintile to 1315 in the richest quintile (Table 2, column B).
The LACE+ quintile was very strongly associated with outcome risk, as shown in Table 2, row D (2=2703, P<0.0001; Mantel‐Haenszel trend 2=2102, P<0.0001). Within each LACE+ stratum, the risk of death or urgent readmission did not appear to consistently change with income quintile. After adjusting for LACE+ scores, income quintile was no longer associated with 30‐day death or readmission (Cochran‐Mantel‐Haenszel 2=5.9, df=4, P=0.21).
We found no nonlinear associations between household‐income quintile and 30‐day death or readmission after adjusting for the LACE+ score. In addition, the association between LACE+ quintile and outcome did not vary significantly by household‐income quintile (P value for interaction term in logistic regression model=0.5582).
The association between income quintile and 30‐day death or urgent readmission decreased when incrementally controlling for other covariates in the LACE+ model (Figure 1). By itself, all income quintiles except 2 were significantly distinct from the poorest income quintile. The addition of patient age, sex, and hospital type had little effect on the association between income and outcomes. The addition of index admission urgency shifted all point estimates toward unity (Figure 1). Associations between income and death or readmission then remained relatively stable until the addition of number of urgent admissions in the previous year (Figure 1). The subsequent addition of number of emergency visits and comorbidities resulted in none of the income quintiles being statistically distinct from the poorest quintile, as well as a nonsignificant linear trend over the quintiles.
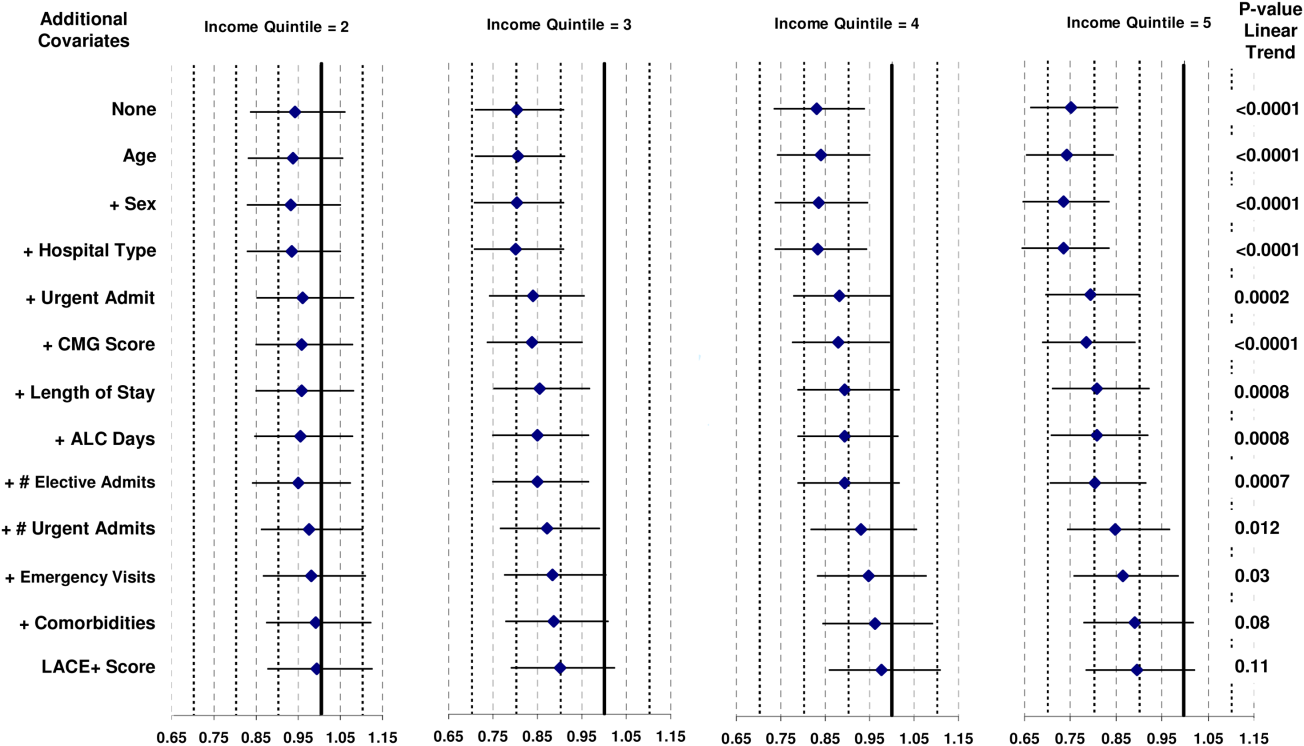
DISCUSSION
Our study shows that the risk of 30‐day death or urgent readmission was higher in people from lower‐income neighborhoods. However, this risk appears to be explained by patient‐level factors that are known to be associated with bad postdischarge outcomes. After accounting for these factors with the LACE+ index, we found no notable changes in the risk of early death or urgent readmission with SES as measured with average neighborhood household income.
Nine previous studies have measured the association between various SES measures and hospital readmission in disparate populations.[3, 4, 5, 6, 7, 8, 9, 10, 11] These studies were done in the United States,[5, 6, 8, 9, 10] the United Kingdom,[3, 7] Australia,[4] and Canada.[11] They used a range of SES indicators (from area‐level measures of household income[5] or deprivation[3] to personal education and income)[8, 9, 10] in diverse patient populations (from a random sample of all hospitalizations[3] to people with disabilities living in New York City)[15] and very different time horizons (capturing hospital readmissions that occurred from within 30 days[5] to 4 years).[10] Of these 9 studies, 5 found no independent association between their SES measure and readmission,[5, 6, 8, 9, 10] and 2 included SES in their final regression model but did not present the modelmaking it impossible to determine if SES significantly influenced outcomes.[3, 15] One study found that the risk of hospital readmission independently increased as a composite measure of area‐level social and economic indicators decreased.[4] A Canadian study[11] measured neighborhood income quintile and showed, after adjusting for patient sex, comorbidities, LOS variance, and previous admissions, that the odds of acute, nonpsychiatric readmission within 30 days of discharge were approximately 10% higher in the lowest versus the highest SES quintile. The ability of this model to adjust for important confounders when associating SES and risk of readmission is uncertain because the model fit was not reported.
Several factors could explain the difference between our study and the previous Canadian analysis showing significantly higher adjusted risk of readmission in patients from the lowest versus the highest SES quintile.[11] First, our analysis had a slightly different outcome, combining early death with urgent readmission (rather than the latter alone). We believe that this combination is important to avoid biased results when associating patient factors with readmission risk.[14] Second, our unit of analysis was the patient, whereas in the previous analysis it was the hospitalization.[11] A recent analysis by our group found that this distinction can change the results on analyses in early postdischarge outcomes.[16] In the present analysis, different results could occur if patients with multiple readmissions were disproportionately prevalent in low‐income neighborhoods. Third, our analysis was limited to Ontario rather than the entire country. Finally, and we believe most importantly, we used a validated model to control for risk of poor outcomes soon after discharge from hospital. Our analysis shows that this risk was strongly associated with neighborhood income (Table 2). This suggests that the association between SES and bad postdischarge outcomes could be explained by factors that independently increase the risk of these outcomes. Adequately controlling for these covariates would then remove variation in readmission risk by SES. We believe that these results highlight the importance of adequately controlling for potential confounders.
We believe that our results are reassuring but not definitive. We found no indication that, in Ontario, people from poorer neighborhoods are systematically more likelyafter considering factors that are known to be associated with early death or urgent readmissionto have a worse outcome early after their discharge from hospital. However, patient income and other SES measures could be associated with early death or readmission for several reasons. First, our study used average neighborhood income quintiles to quantify SES. It is possible that other SES measures (such as education or social deprivation) or patient‐level SES indicators could be significantly associated with early death or readmission.[17, 18] Second, we previously found that approximately only 25% of hospital readmissions are potentially avoidable.[19] Further study is required to determine if patient SES independently influences potentially avoidable hospital readmissions. Third, we cannot be certain how our results might generalize to health populations outside of Ontario. Specifically, SES might play a more important role in regions without universal healthcare in which community‐based healthcare resources that could decrease readmission risk, such as medications or physician follow‐up, are unavailable to those without health insurance coverage. Finally, we found notable confounding between neighborhood income quintile and factors known to be independently associated with early death or urgent readmission (Figure 1). This was especially prominent with index admission urgency, number of previous urgent admissions and emergency visits, and patient comorbidities. These factors have a much stronger association with early death or readmission than neighborhood income quintile. If low neighborhood income actually results in urgent hospital admission, emergency‐department visits, and comorbidities, then the inclusion of these covariates in the model could obscure the influence of neighborhood income on early death or readmission.
In summary, our study found that neighborhood income was not associated with early death or urgent readmission independent of known risk factors. Our analysis indicates that focusing resources on patients in lower‐income neighborhoods is unlikely to change the risk of early postdischarge adverse events. Further study is required to determine if SES is associated with adverse postdischarge outcomes in settings without publicly funded healthcare.
Acknowledgment
Disclosure: Nothing to report.
- Last JM, ed. A Dictionary of Epidemiology. 3rd ed. New York, NY: Oxford University Press; 1995.
- Is income inequality a determinant of population health? Part 1: A systematic review. Milbank Q. 2004;82(1):5–99. , , , et al.
- Identifying patients at high risk of emergency hospital admissions: a logistic regression analysis. J R Soc Med. 2006;99(8):406–414. , , .
- Using routine inpatient data to identify patients at risk of hospital readmission. BMC Health Serv Res. 2009;9:96. , , , .
- Risk factors for 30‐day hospital readmission in patients ≥65 years of age. Proc (Bayl Univ Med Cent). 2008;21(4):363–372. , , , , .
- An automated model to identify heart failure patients at risk for 30‐day readmission or death using electronic medical record data. Med Care. 2010;48(11):981–988. , , , et al.
- Improving the management of care for high‐cost Medicaid patients. Health Aff (Millwood). 2007;26(6):1643–1654. , .
- Factors predicting readmission of older general medicine patients. J Gen Intern Med. 1991;6(5):389–393. , .
- Hospital readmission in general medicine patients: a prediction model. J Gen Intern Med. 2010;25(3):211–219. , , , et al.
- Screening elders for risk of hospital admission. J Am Geriatr Soc. 1993;41(8):811–817. , , , , , .
- Canadian Institute for Health Information. All‐Cause Readmission to Acute Care and Return to the Emergency Department. Ottawa, ON: Canadian Institute for Health Information; 2012:1–64.
- LACE+ index: extension of a validated index to predict early death or unplanned readmission following hospital discharge using administrative data. Open Medicine. 2012;6(2):80–89. , , .
- PCCF Plus version 5E user's guide. Ottawa ON: Statistics Canada; 2009;82F0086‐XDB. .
- Derivation and validation of diagnostic score based on case‐mix groups to predict 30‐day death or urgent readmission. Open Medicine. 2012;6(3):e80–e89. , , .
- Executing high‐quality care transitions: a call to do it right. J Hosp Med. 2007;2(5):287–290. , .
- Predicting post‐discharge death or readmission: deterioration of model performance in a population having multiple admissions per patient [published online ahead of print November 19, 2012]. J Eval Clin Pract. doi: 10.1111/jep.12012. , , , .
- Patient self‐management of chronic disease in primary care. JAMA. 2002;288(19): 2469–2475. , , , .
- Multilevel analyses of neighbourhood socioeconomic context and health outcomes: a critical review. J Epidemiol Community Health. 2001;55(2):111–122. , .
- Incidence of potentially avoidable hospital readmissions and its relationship to all‐cause urgent readmissions. CMAJ. 2011;183(14):E1067–E1072. , , , et al.
- Validation of a combined comorbidity index. J Clin Epidemiol. 1994;47(11):1245–1251. , , , .
Socioeconomic status (SES) classifies people according to occupation, prior education, or income.[1] Socioeconomic status has been associated with several population‐health outcomes, albeit with geographically inconsistent results.[2] If lower SES is associated with higher readmission rates, then further studies could be done to determine which specific socioeconomic factors are potentially modifiable and whether the provision of additional resources could allay the increased risk associated with those factors.
Nine studies have examined the association between SES and readmissions.[3, 4, 5, 6, 7, 8, 9, 10, 11] These studies varied extensively in methodologies, SES measures, and results. However, results from 1 of these studies[11] were particularly notable given the study's significant association between lower household income and increased risk of acute readmission in a publicly funded, open‐access healthcare system. Given the implications of these results, an accurate and explicit assessment of the association between SES measures and the risk of adverse postdischarge outcomes is important.
We recently developed a model that accurately predicts the risk of 30‐day death or urgent readmission using administrative data.[12] This model did not directly control for any SES factors. In this study, we determined if a commonly used SES measurehousehold‐income quintilewas associated with the risk of early death or urgent readmission after controlling for factors known to influence this outcome.
METHODS
Study Setting and Data Sources
This population‐based study took place in Ontario, Canada, between April 1, 2003 and March 31, 2009. All hospital and physician care in Ontario is publicly funded. The study used 2 databases, the Discharge Abstract Database and the Registered Persons Database. The Discharge Abstract Database records information about all nonpsychiatric hospitalizations, including dates of hospital admission and discharge, vital status at end of hospitalization, discharge destination (ie, community, nursing home, or chronic hospital), admission urgency, primary and other diagnoses, and postal code of patient's household. The Registered Persons Database captures basic demographic data about all Ontarians, including date of birth and date of death (if applicable), postal code of residence, and average household‐income quintile of postal code, determined by linking the postal code to Statistics Canada geographical units through the Postal Code Conversion File Plus.[13] The Registered Persons Database captures all deaths regardless of the death location (ie, community vs hospital).
Study Population
This study used patients from a previous analysis that internally validated an index to predict the risk of 30‐day death or urgent readmission.[12] This analysis included a simple random sample of 250,000 adult Ontarians (age >18 years) who were discharged from the hospital to the community between April 1, 2003 and March 31, 2009. These medical and surgical hospitalizations were sampled from the Discharge Abstract Database described above. Psychiatric admissions were excluded because their hospitalizations are captured in a distinct database; obstetrical admissions were also excluded because they have a very low risk of 30‐day death or readmission. We randomly chose 1 index admission per person to ensure that the patient was the unit of analysis.
For the present study, we selected all patients from the previous analysis who were discharged from the hospital in 2006. This year was chosen because the SES indicator we used in the study (average household‐income quintile) was measured during the 2006 Canadian Census and would be most accurate for patients discharged in that year. The present study also limited patients to those with a valid postal code, because this was required to link patients to their neighborhood and their household‐income quintile.
Study Outcome
The study outcome was all‐cause death or urgent readmission within 30 days of discharge from hospital. We combined death with urgent readmission to avoid potential biases that could occur when measuring associations between risk factors and urgent readmission; in analyses having readmission as the sole outcome, the categorization of early deaths that occur prior to readmission as nonevents could minimize the importance of factors (such as severe comorbidities or patient age) that are associated with both early death and readmission.
We linked to the Registered Patients Database to determine each person's 30‐day death status. We linked to the Discharge Abstract Database to determine if patients had been urgently readmitted to any hospital within 30 days of discharge. All deaths were considered regardless of cause. All urgent (ie, nonscheduled) readmissions were included regardless of the reason for admission. Urgent status was determined by the urgency field in the Discharge Abstract Database, for which data abstractors are instructed to classify all nonscheduled admissions as urgent; these admissions frequently include those admitted after presenting to the emergency department.
Study Covariates: Readmission Risk and Neighborhood Household‐Income Quintile
In our primary analysis, we quantified the risk of 30‐day death or urgent readmission using an internally validated index, the LACE+ index: length of stay (L), acuity of the admission (A), comorbidity of the patient (measured with the Charlson Comorbidity Index score (C), and emergency‐department use (E).[12] The LACE+ index predicts the risk of 30‐day all‐cause death or urgent readmission for nonpsychiatric and nonobstetrical admissions. This index includes patient age, sex, comorbidities, and previous hospital and emergency‐department utilization; admission urgency; hospital type; total length of stay (LOS) and days in hospital awaiting placement; and hospitalization diagnostic risk.[14] The index quantified outcome risk as a score that ranged from 17 to 114. It was very discriminatory (C statistic, 77.1%) and was well calibrated (the observed and expected outcome risk was statistically distinct in only 2 of 14 risk groups that contained <2% of the population). The LACE+ quintiles were defined using score distribution from the entire 20032009 cohort.[12]
We used neighborhood income quintile as 1 measure of patient SES. Neighborhood income quintile was calculated by Statistics Canada using the Income Per Person Equivalent (IPPE) determined from the 2006 Canadian census.[13] The IPPE was calculated as total household income divided by the Single Persons Equivalent, which reflects decreased costs per person (and therefore increased available income per household occupant) in households having greater numbers of people. Within each dissemination area (each contains 400700 people), the average IPPE was calculated. Then, within each region (delineated by the Census Metropolitan Area, the Census Agglomeration, or provincial residual areas), dissemination areas were ranked by their average IPPE and then categorized into quintiles. These household‐income quintiles, therefore, are community‐specific and ensure that neighborhood household incomes are categorized based on comparisons within the same community. As such, the income thresholds for quintile categorization will vary between regions. We linked each patient's postal code to their dissemination area using the Postal Code Conversion File Plus[13] to determine their neighborhood income quintile.
Analysis
We described the patient cohort by readmission status. We categorized the expected risk of 30‐day death or urgent readmission to hospital (as determined by the LACE+ score) into quintiles. We used the 2 test and the test for trend to determine the association of these risk quintiles and SES quintiles with observed rates of 30‐day death or urgent readmission. The Cochran‐Mantel‐Haenszel test was used to determine the association of household‐income quintile and outcome risk after adjusting for LACE+ quintile.
To determine how the association between income quintile and outcome changes with increase adjustment, we constructed a series of logistic‐regression models that contained household‐income quintile and the sequential addition of components of the LACE+ score. For each model, we measured the influence of these added covariates on the association between household‐income quintile and early death or urgent readmission. We used orthogonal parameterization (which facilitates the comparison of parameter estimates in a regression model) to measure linear trends in the association of the income quintiles with outcomes.
RESULTS
The original cohort contained 250,000 people, of which 40,827 people (16.3%) were included in the present study (208,995 were excluded because patients were discharged in years other than 2006; 178 were excluded because of invalid postal codes).
Patients are described in Table 1. Patients were middle‐aged and had few documented chronic comorbidities. Of the patients, 37% had been to the emergency department and 12% had been admitted urgently. Most admissions were to large, nonteaching hospitals with a median LOS of 3 days.
Variable | Value | No Death/Readmission, n=38,189 | Death/Readmission, n=2,638 | Overall, N=40,827 | |||
---|---|---|---|---|---|---|---|
| |||||||
Mean age (SD), y | 57.39 (18.3) | 67.17 (17.2) | 58.02 (18.4) | ||||
Female sex | 20,044 | 52.5% | 1,291 | 48.9% | 21,335 | 52.3% | |
Charlson index | 0 | 28,908 | 75.7% | 1,238 | 46.9% | 30,146 | 73.8% |
1 | 450 | 11.7% | 362 | 13.7% | 4,812 | 11.8% | |
2 | 2,668 | 7.0% | 427 | 16.2% | 3,095 | 7.6% | |
3+ | 2,163 | 5.7% | 611 | 23.2% | 2,774 | 6.8% | |
ED visits in previous 6 moths | 0 | 24,599 | 64.4% | 1,210 | 45.9% | 25,809 | 63.2% |
12 | 11,262 | 29.5% | 1,008 | 38.2% | 12,270 | 30.1% | |
3+ | 2,328 | 6.1% | 420 | 15.9% | 2,748 | 6.7% | |
Urgent hospitalizations, previous year | 0 | 33,729 | 88.3% | 1,796 | 68.1% | 35,525 | 87.0% |
1 | 3,425 | 9.0% | 525 | 19.9% | 3,950 | 9.7% | |
1+ | 1,035 | 2.7% | 317 | 12.0% | 1,352 | 3.3% | |
Elective hospitalizations, previous year | 0 | 35,988 | 94.2% | 2,389 | 90.6% | 38,377 | 94.0% |
1 | 1,998 | 5.2% | 213 | 8.1% | 2,211 | 5.4% | |
2+ | 203 | 0.5% | 36 | 1.4% | 239 | 0.6% | |
Hospital type | Nonteaching, large | 20,554 | 53.8% | 1,334 | 50.6% | 21,888 | 53.6% |
Nonteaching, small | 5,239 | 13.7% | 487 | 18.5% | 5726 | 14.0% | |
Teaching | 12,396 | 32.5% | 817 | 31.0% | 13,213 | 32.4% | |
Urgent admit | 23,769 | 62.2% | 2,223 | 84.3% | 25,992 | 63.7% | |
LOS rounded to nearest day, median (IQR) | 3 (26) | 5 (311) | 3 (26) | ||||
Any hospital days on ALC | 0 | 646 | 1.7% | 127 | 4.8% | 773 | 1.9% |
CMG score of index admission | 0 | 27,257 | 71.4% | 1,594 | 60.4% | 28,851 | 70.7% |
1+ | 5,218 | 13.7% | 948 | 35.9% | 6,166 | 15.1% | |
<0 | 5,714 | 15.0% | 96 | 3.6% | 5,810 | 14.2% | |
LACE+ score of index admission, median (IQR) | 31 (1848) | 61 (4175) | 32 (1951) | ||||
Household‐income quintile | 1 (poorest) | 7,798 | 20.4% | 621 | 23.5% | 8,419 | 20.6% |
2 | 7,812 | 20.5% | 586 | 22.2% | 8,398 | 20.6% | |
3 | 7,557 | 19.8% | 484 | 18.3% | 8,041 | 19.7% | |
4 | 7,561 | 19.8% | 500 | 19.0% | 8,061 | 19.7% | |
5 (richest) | 7,461 | 19.5% | 447 | 16.9% | 7,908 | 19.4% |
Death or urgent readmission within 30 days occurred in 2638 people (6.5%) (Table 1). Outcome risk increased with age; in males; as comorbidities increased; with greater numbers of emergency‐department visits, urgent admissions, and previous elective admissions; when index admissions were emergent; with longer hospital LOS and increased number of alternate level of care days; and as the diagnostic risk (measured as the Case Mix Group [CMG] score)[14] increased. Outcome risk increased as income quintile became poorer.
Household Income and Risk of 30‐Day Death or Urgent Readmission
People were evenly divided among the income quintiles (Table 2). By itself, household‐income quintile was significantly associated with the risk of early death or urgent hospital readmission (Table 2, column C, 2=27.4, P<0.0001; Mantel‐Haenszel trend 2=24.3, P<0.0001). In the poorest quintile, 7.4% of people had an outcome, compared with 5.6% in the richest quintile (2=19.8, df=1, P<0.0001).
Risk Quintile of 30‐Day Death or Readmission (LACE+ Points Range) | ||||||
---|---|---|---|---|---|---|
1 (1416) [A] | 2 (1727) | 3 (2839) | 4 (4056) | 5 (57114) [B] | Income Quintile Overall [C] | |
| ||||||
Income quintile | ||||||
1 (poorest) | 18/1,485 (1.2%) | 42/1,667 (2.5%) | 65/1,635 (4.0%) | 117/1,722 (6.8%) | 379/1,910 (19.8%) | 621/8,419 (7.4%) |
2 | 21/1,627 (1.3%) | 39/1,665 (2.3%) | 65/1,598 (4.1%) | 130/1,808 (5.2%) | 331/1,700 (19.5%) | 586/8,398 (7.0%) |
3 | 18/1,761 (1.0%) | 33/1,665 (2.0%) | 63/1,568 (4.0%) | 96/1,499 (6.4%) | 274/1,548 (17.7%) | 484/8,041 (6.0%) |
4 | 27/1,851 (1.5%) | 42/1,698 (2.4%) | 57/1,585 (3.6%) | 110/1,548 (6.1%) | 264/1,379 (19.1%) | 500/8,061 (6.2%) |
5 (richest) | 20/1,864 (1.1%) | 32/1,736 (1.8%) | 60/1,468 (4.1%) | 107/1,525 (7.0%) | 228/1,315 (17.3%) | 447/7,908 (5.6%) |
Risk quintile overall [D] | 104/8,588 (1.2%) | 188/8,431 (2.2%) | 310/7,854 (4.0%) | 560/8,102 (6.9%) | 1476/7,852 (18.8%) | 2,638/40,827 (6.5%) |
However, household income was also strongly associated with LACE+ scores (2=240, P<0.0001; Mantel‐Haenszel trend 2=209, P<0.0001). The number of people in the lowest‐risk quintile increased with income, from 1485 in the poorest quintile to 1864 in the richest quintile (Table 2, column A). In contrast, the number of high‐risk people progressively decreased with income, from 1910 in the poorest quintile to 1315 in the richest quintile (Table 2, column B).
The LACE+ quintile was very strongly associated with outcome risk, as shown in Table 2, row D (2=2703, P<0.0001; Mantel‐Haenszel trend 2=2102, P<0.0001). Within each LACE+ stratum, the risk of death or urgent readmission did not appear to consistently change with income quintile. After adjusting for LACE+ scores, income quintile was no longer associated with 30‐day death or readmission (Cochran‐Mantel‐Haenszel 2=5.9, df=4, P=0.21).
We found no nonlinear associations between household‐income quintile and 30‐day death or readmission after adjusting for the LACE+ score. In addition, the association between LACE+ quintile and outcome did not vary significantly by household‐income quintile (P value for interaction term in logistic regression model=0.5582).
The association between income quintile and 30‐day death or urgent readmission decreased when incrementally controlling for other covariates in the LACE+ model (Figure 1). By itself, all income quintiles except 2 were significantly distinct from the poorest income quintile. The addition of patient age, sex, and hospital type had little effect on the association between income and outcomes. The addition of index admission urgency shifted all point estimates toward unity (Figure 1). Associations between income and death or readmission then remained relatively stable until the addition of number of urgent admissions in the previous year (Figure 1). The subsequent addition of number of emergency visits and comorbidities resulted in none of the income quintiles being statistically distinct from the poorest quintile, as well as a nonsignificant linear trend over the quintiles.
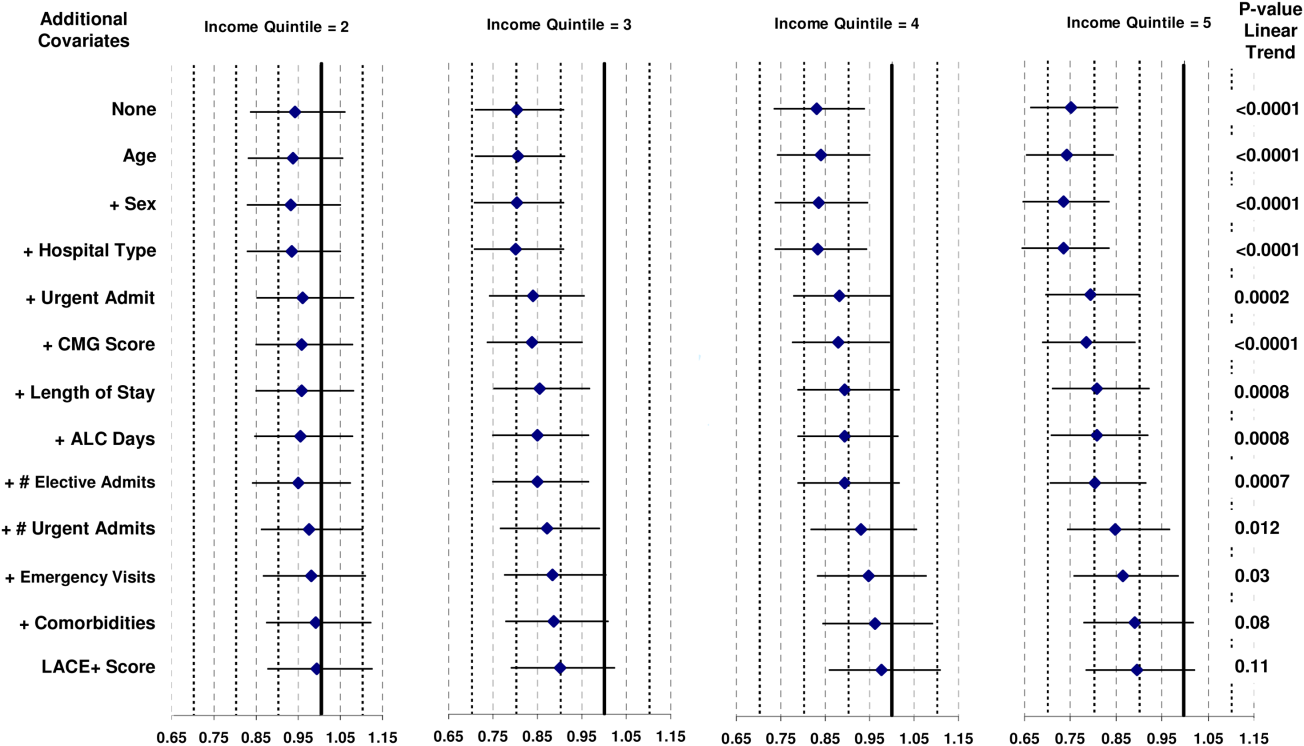
DISCUSSION
Our study shows that the risk of 30‐day death or urgent readmission was higher in people from lower‐income neighborhoods. However, this risk appears to be explained by patient‐level factors that are known to be associated with bad postdischarge outcomes. After accounting for these factors with the LACE+ index, we found no notable changes in the risk of early death or urgent readmission with SES as measured with average neighborhood household income.
Nine previous studies have measured the association between various SES measures and hospital readmission in disparate populations.[3, 4, 5, 6, 7, 8, 9, 10, 11] These studies were done in the United States,[5, 6, 8, 9, 10] the United Kingdom,[3, 7] Australia,[4] and Canada.[11] They used a range of SES indicators (from area‐level measures of household income[5] or deprivation[3] to personal education and income)[8, 9, 10] in diverse patient populations (from a random sample of all hospitalizations[3] to people with disabilities living in New York City)[15] and very different time horizons (capturing hospital readmissions that occurred from within 30 days[5] to 4 years).[10] Of these 9 studies, 5 found no independent association between their SES measure and readmission,[5, 6, 8, 9, 10] and 2 included SES in their final regression model but did not present the modelmaking it impossible to determine if SES significantly influenced outcomes.[3, 15] One study found that the risk of hospital readmission independently increased as a composite measure of area‐level social and economic indicators decreased.[4] A Canadian study[11] measured neighborhood income quintile and showed, after adjusting for patient sex, comorbidities, LOS variance, and previous admissions, that the odds of acute, nonpsychiatric readmission within 30 days of discharge were approximately 10% higher in the lowest versus the highest SES quintile. The ability of this model to adjust for important confounders when associating SES and risk of readmission is uncertain because the model fit was not reported.
Several factors could explain the difference between our study and the previous Canadian analysis showing significantly higher adjusted risk of readmission in patients from the lowest versus the highest SES quintile.[11] First, our analysis had a slightly different outcome, combining early death with urgent readmission (rather than the latter alone). We believe that this combination is important to avoid biased results when associating patient factors with readmission risk.[14] Second, our unit of analysis was the patient, whereas in the previous analysis it was the hospitalization.[11] A recent analysis by our group found that this distinction can change the results on analyses in early postdischarge outcomes.[16] In the present analysis, different results could occur if patients with multiple readmissions were disproportionately prevalent in low‐income neighborhoods. Third, our analysis was limited to Ontario rather than the entire country. Finally, and we believe most importantly, we used a validated model to control for risk of poor outcomes soon after discharge from hospital. Our analysis shows that this risk was strongly associated with neighborhood income (Table 2). This suggests that the association between SES and bad postdischarge outcomes could be explained by factors that independently increase the risk of these outcomes. Adequately controlling for these covariates would then remove variation in readmission risk by SES. We believe that these results highlight the importance of adequately controlling for potential confounders.
We believe that our results are reassuring but not definitive. We found no indication that, in Ontario, people from poorer neighborhoods are systematically more likelyafter considering factors that are known to be associated with early death or urgent readmissionto have a worse outcome early after their discharge from hospital. However, patient income and other SES measures could be associated with early death or readmission for several reasons. First, our study used average neighborhood income quintiles to quantify SES. It is possible that other SES measures (such as education or social deprivation) or patient‐level SES indicators could be significantly associated with early death or readmission.[17, 18] Second, we previously found that approximately only 25% of hospital readmissions are potentially avoidable.[19] Further study is required to determine if patient SES independently influences potentially avoidable hospital readmissions. Third, we cannot be certain how our results might generalize to health populations outside of Ontario. Specifically, SES might play a more important role in regions without universal healthcare in which community‐based healthcare resources that could decrease readmission risk, such as medications or physician follow‐up, are unavailable to those without health insurance coverage. Finally, we found notable confounding between neighborhood income quintile and factors known to be independently associated with early death or urgent readmission (Figure 1). This was especially prominent with index admission urgency, number of previous urgent admissions and emergency visits, and patient comorbidities. These factors have a much stronger association with early death or readmission than neighborhood income quintile. If low neighborhood income actually results in urgent hospital admission, emergency‐department visits, and comorbidities, then the inclusion of these covariates in the model could obscure the influence of neighborhood income on early death or readmission.
In summary, our study found that neighborhood income was not associated with early death or urgent readmission independent of known risk factors. Our analysis indicates that focusing resources on patients in lower‐income neighborhoods is unlikely to change the risk of early postdischarge adverse events. Further study is required to determine if SES is associated with adverse postdischarge outcomes in settings without publicly funded healthcare.
Acknowledgment
Disclosure: Nothing to report.
Socioeconomic status (SES) classifies people according to occupation, prior education, or income.[1] Socioeconomic status has been associated with several population‐health outcomes, albeit with geographically inconsistent results.[2] If lower SES is associated with higher readmission rates, then further studies could be done to determine which specific socioeconomic factors are potentially modifiable and whether the provision of additional resources could allay the increased risk associated with those factors.
Nine studies have examined the association between SES and readmissions.[3, 4, 5, 6, 7, 8, 9, 10, 11] These studies varied extensively in methodologies, SES measures, and results. However, results from 1 of these studies[11] were particularly notable given the study's significant association between lower household income and increased risk of acute readmission in a publicly funded, open‐access healthcare system. Given the implications of these results, an accurate and explicit assessment of the association between SES measures and the risk of adverse postdischarge outcomes is important.
We recently developed a model that accurately predicts the risk of 30‐day death or urgent readmission using administrative data.[12] This model did not directly control for any SES factors. In this study, we determined if a commonly used SES measurehousehold‐income quintilewas associated with the risk of early death or urgent readmission after controlling for factors known to influence this outcome.
METHODS
Study Setting and Data Sources
This population‐based study took place in Ontario, Canada, between April 1, 2003 and March 31, 2009. All hospital and physician care in Ontario is publicly funded. The study used 2 databases, the Discharge Abstract Database and the Registered Persons Database. The Discharge Abstract Database records information about all nonpsychiatric hospitalizations, including dates of hospital admission and discharge, vital status at end of hospitalization, discharge destination (ie, community, nursing home, or chronic hospital), admission urgency, primary and other diagnoses, and postal code of patient's household. The Registered Persons Database captures basic demographic data about all Ontarians, including date of birth and date of death (if applicable), postal code of residence, and average household‐income quintile of postal code, determined by linking the postal code to Statistics Canada geographical units through the Postal Code Conversion File Plus.[13] The Registered Persons Database captures all deaths regardless of the death location (ie, community vs hospital).
Study Population
This study used patients from a previous analysis that internally validated an index to predict the risk of 30‐day death or urgent readmission.[12] This analysis included a simple random sample of 250,000 adult Ontarians (age >18 years) who were discharged from the hospital to the community between April 1, 2003 and March 31, 2009. These medical and surgical hospitalizations were sampled from the Discharge Abstract Database described above. Psychiatric admissions were excluded because their hospitalizations are captured in a distinct database; obstetrical admissions were also excluded because they have a very low risk of 30‐day death or readmission. We randomly chose 1 index admission per person to ensure that the patient was the unit of analysis.
For the present study, we selected all patients from the previous analysis who were discharged from the hospital in 2006. This year was chosen because the SES indicator we used in the study (average household‐income quintile) was measured during the 2006 Canadian Census and would be most accurate for patients discharged in that year. The present study also limited patients to those with a valid postal code, because this was required to link patients to their neighborhood and their household‐income quintile.
Study Outcome
The study outcome was all‐cause death or urgent readmission within 30 days of discharge from hospital. We combined death with urgent readmission to avoid potential biases that could occur when measuring associations between risk factors and urgent readmission; in analyses having readmission as the sole outcome, the categorization of early deaths that occur prior to readmission as nonevents could minimize the importance of factors (such as severe comorbidities or patient age) that are associated with both early death and readmission.
We linked to the Registered Patients Database to determine each person's 30‐day death status. We linked to the Discharge Abstract Database to determine if patients had been urgently readmitted to any hospital within 30 days of discharge. All deaths were considered regardless of cause. All urgent (ie, nonscheduled) readmissions were included regardless of the reason for admission. Urgent status was determined by the urgency field in the Discharge Abstract Database, for which data abstractors are instructed to classify all nonscheduled admissions as urgent; these admissions frequently include those admitted after presenting to the emergency department.
Study Covariates: Readmission Risk and Neighborhood Household‐Income Quintile
In our primary analysis, we quantified the risk of 30‐day death or urgent readmission using an internally validated index, the LACE+ index: length of stay (L), acuity of the admission (A), comorbidity of the patient (measured with the Charlson Comorbidity Index score (C), and emergency‐department use (E).[12] The LACE+ index predicts the risk of 30‐day all‐cause death or urgent readmission for nonpsychiatric and nonobstetrical admissions. This index includes patient age, sex, comorbidities, and previous hospital and emergency‐department utilization; admission urgency; hospital type; total length of stay (LOS) and days in hospital awaiting placement; and hospitalization diagnostic risk.[14] The index quantified outcome risk as a score that ranged from 17 to 114. It was very discriminatory (C statistic, 77.1%) and was well calibrated (the observed and expected outcome risk was statistically distinct in only 2 of 14 risk groups that contained <2% of the population). The LACE+ quintiles were defined using score distribution from the entire 20032009 cohort.[12]
We used neighborhood income quintile as 1 measure of patient SES. Neighborhood income quintile was calculated by Statistics Canada using the Income Per Person Equivalent (IPPE) determined from the 2006 Canadian census.[13] The IPPE was calculated as total household income divided by the Single Persons Equivalent, which reflects decreased costs per person (and therefore increased available income per household occupant) in households having greater numbers of people. Within each dissemination area (each contains 400700 people), the average IPPE was calculated. Then, within each region (delineated by the Census Metropolitan Area, the Census Agglomeration, or provincial residual areas), dissemination areas were ranked by their average IPPE and then categorized into quintiles. These household‐income quintiles, therefore, are community‐specific and ensure that neighborhood household incomes are categorized based on comparisons within the same community. As such, the income thresholds for quintile categorization will vary between regions. We linked each patient's postal code to their dissemination area using the Postal Code Conversion File Plus[13] to determine their neighborhood income quintile.
Analysis
We described the patient cohort by readmission status. We categorized the expected risk of 30‐day death or urgent readmission to hospital (as determined by the LACE+ score) into quintiles. We used the 2 test and the test for trend to determine the association of these risk quintiles and SES quintiles with observed rates of 30‐day death or urgent readmission. The Cochran‐Mantel‐Haenszel test was used to determine the association of household‐income quintile and outcome risk after adjusting for LACE+ quintile.
To determine how the association between income quintile and outcome changes with increase adjustment, we constructed a series of logistic‐regression models that contained household‐income quintile and the sequential addition of components of the LACE+ score. For each model, we measured the influence of these added covariates on the association between household‐income quintile and early death or urgent readmission. We used orthogonal parameterization (which facilitates the comparison of parameter estimates in a regression model) to measure linear trends in the association of the income quintiles with outcomes.
RESULTS
The original cohort contained 250,000 people, of which 40,827 people (16.3%) were included in the present study (208,995 were excluded because patients were discharged in years other than 2006; 178 were excluded because of invalid postal codes).
Patients are described in Table 1. Patients were middle‐aged and had few documented chronic comorbidities. Of the patients, 37% had been to the emergency department and 12% had been admitted urgently. Most admissions were to large, nonteaching hospitals with a median LOS of 3 days.
Variable | Value | No Death/Readmission, n=38,189 | Death/Readmission, n=2,638 | Overall, N=40,827 | |||
---|---|---|---|---|---|---|---|
| |||||||
Mean age (SD), y | 57.39 (18.3) | 67.17 (17.2) | 58.02 (18.4) | ||||
Female sex | 20,044 | 52.5% | 1,291 | 48.9% | 21,335 | 52.3% | |
Charlson index | 0 | 28,908 | 75.7% | 1,238 | 46.9% | 30,146 | 73.8% |
1 | 450 | 11.7% | 362 | 13.7% | 4,812 | 11.8% | |
2 | 2,668 | 7.0% | 427 | 16.2% | 3,095 | 7.6% | |
3+ | 2,163 | 5.7% | 611 | 23.2% | 2,774 | 6.8% | |
ED visits in previous 6 moths | 0 | 24,599 | 64.4% | 1,210 | 45.9% | 25,809 | 63.2% |
12 | 11,262 | 29.5% | 1,008 | 38.2% | 12,270 | 30.1% | |
3+ | 2,328 | 6.1% | 420 | 15.9% | 2,748 | 6.7% | |
Urgent hospitalizations, previous year | 0 | 33,729 | 88.3% | 1,796 | 68.1% | 35,525 | 87.0% |
1 | 3,425 | 9.0% | 525 | 19.9% | 3,950 | 9.7% | |
1+ | 1,035 | 2.7% | 317 | 12.0% | 1,352 | 3.3% | |
Elective hospitalizations, previous year | 0 | 35,988 | 94.2% | 2,389 | 90.6% | 38,377 | 94.0% |
1 | 1,998 | 5.2% | 213 | 8.1% | 2,211 | 5.4% | |
2+ | 203 | 0.5% | 36 | 1.4% | 239 | 0.6% | |
Hospital type | Nonteaching, large | 20,554 | 53.8% | 1,334 | 50.6% | 21,888 | 53.6% |
Nonteaching, small | 5,239 | 13.7% | 487 | 18.5% | 5726 | 14.0% | |
Teaching | 12,396 | 32.5% | 817 | 31.0% | 13,213 | 32.4% | |
Urgent admit | 23,769 | 62.2% | 2,223 | 84.3% | 25,992 | 63.7% | |
LOS rounded to nearest day, median (IQR) | 3 (26) | 5 (311) | 3 (26) | ||||
Any hospital days on ALC | 0 | 646 | 1.7% | 127 | 4.8% | 773 | 1.9% |
CMG score of index admission | 0 | 27,257 | 71.4% | 1,594 | 60.4% | 28,851 | 70.7% |
1+ | 5,218 | 13.7% | 948 | 35.9% | 6,166 | 15.1% | |
<0 | 5,714 | 15.0% | 96 | 3.6% | 5,810 | 14.2% | |
LACE+ score of index admission, median (IQR) | 31 (1848) | 61 (4175) | 32 (1951) | ||||
Household‐income quintile | 1 (poorest) | 7,798 | 20.4% | 621 | 23.5% | 8,419 | 20.6% |
2 | 7,812 | 20.5% | 586 | 22.2% | 8,398 | 20.6% | |
3 | 7,557 | 19.8% | 484 | 18.3% | 8,041 | 19.7% | |
4 | 7,561 | 19.8% | 500 | 19.0% | 8,061 | 19.7% | |
5 (richest) | 7,461 | 19.5% | 447 | 16.9% | 7,908 | 19.4% |
Death or urgent readmission within 30 days occurred in 2638 people (6.5%) (Table 1). Outcome risk increased with age; in males; as comorbidities increased; with greater numbers of emergency‐department visits, urgent admissions, and previous elective admissions; when index admissions were emergent; with longer hospital LOS and increased number of alternate level of care days; and as the diagnostic risk (measured as the Case Mix Group [CMG] score)[14] increased. Outcome risk increased as income quintile became poorer.
Household Income and Risk of 30‐Day Death or Urgent Readmission
People were evenly divided among the income quintiles (Table 2). By itself, household‐income quintile was significantly associated with the risk of early death or urgent hospital readmission (Table 2, column C, 2=27.4, P<0.0001; Mantel‐Haenszel trend 2=24.3, P<0.0001). In the poorest quintile, 7.4% of people had an outcome, compared with 5.6% in the richest quintile (2=19.8, df=1, P<0.0001).
Risk Quintile of 30‐Day Death or Readmission (LACE+ Points Range) | ||||||
---|---|---|---|---|---|---|
1 (1416) [A] | 2 (1727) | 3 (2839) | 4 (4056) | 5 (57114) [B] | Income Quintile Overall [C] | |
| ||||||
Income quintile | ||||||
1 (poorest) | 18/1,485 (1.2%) | 42/1,667 (2.5%) | 65/1,635 (4.0%) | 117/1,722 (6.8%) | 379/1,910 (19.8%) | 621/8,419 (7.4%) |
2 | 21/1,627 (1.3%) | 39/1,665 (2.3%) | 65/1,598 (4.1%) | 130/1,808 (5.2%) | 331/1,700 (19.5%) | 586/8,398 (7.0%) |
3 | 18/1,761 (1.0%) | 33/1,665 (2.0%) | 63/1,568 (4.0%) | 96/1,499 (6.4%) | 274/1,548 (17.7%) | 484/8,041 (6.0%) |
4 | 27/1,851 (1.5%) | 42/1,698 (2.4%) | 57/1,585 (3.6%) | 110/1,548 (6.1%) | 264/1,379 (19.1%) | 500/8,061 (6.2%) |
5 (richest) | 20/1,864 (1.1%) | 32/1,736 (1.8%) | 60/1,468 (4.1%) | 107/1,525 (7.0%) | 228/1,315 (17.3%) | 447/7,908 (5.6%) |
Risk quintile overall [D] | 104/8,588 (1.2%) | 188/8,431 (2.2%) | 310/7,854 (4.0%) | 560/8,102 (6.9%) | 1476/7,852 (18.8%) | 2,638/40,827 (6.5%) |
However, household income was also strongly associated with LACE+ scores (2=240, P<0.0001; Mantel‐Haenszel trend 2=209, P<0.0001). The number of people in the lowest‐risk quintile increased with income, from 1485 in the poorest quintile to 1864 in the richest quintile (Table 2, column A). In contrast, the number of high‐risk people progressively decreased with income, from 1910 in the poorest quintile to 1315 in the richest quintile (Table 2, column B).
The LACE+ quintile was very strongly associated with outcome risk, as shown in Table 2, row D (2=2703, P<0.0001; Mantel‐Haenszel trend 2=2102, P<0.0001). Within each LACE+ stratum, the risk of death or urgent readmission did not appear to consistently change with income quintile. After adjusting for LACE+ scores, income quintile was no longer associated with 30‐day death or readmission (Cochran‐Mantel‐Haenszel 2=5.9, df=4, P=0.21).
We found no nonlinear associations between household‐income quintile and 30‐day death or readmission after adjusting for the LACE+ score. In addition, the association between LACE+ quintile and outcome did not vary significantly by household‐income quintile (P value for interaction term in logistic regression model=0.5582).
The association between income quintile and 30‐day death or urgent readmission decreased when incrementally controlling for other covariates in the LACE+ model (Figure 1). By itself, all income quintiles except 2 were significantly distinct from the poorest income quintile. The addition of patient age, sex, and hospital type had little effect on the association between income and outcomes. The addition of index admission urgency shifted all point estimates toward unity (Figure 1). Associations between income and death or readmission then remained relatively stable until the addition of number of urgent admissions in the previous year (Figure 1). The subsequent addition of number of emergency visits and comorbidities resulted in none of the income quintiles being statistically distinct from the poorest quintile, as well as a nonsignificant linear trend over the quintiles.
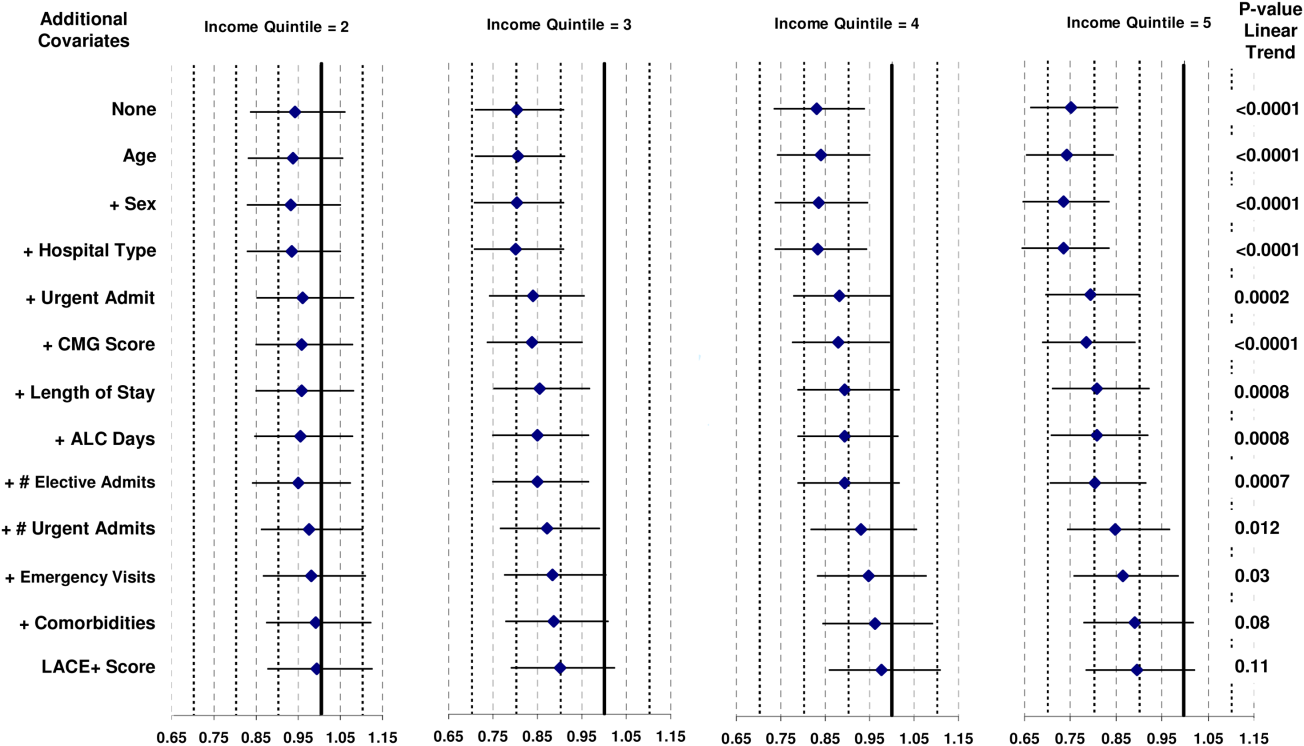
DISCUSSION
Our study shows that the risk of 30‐day death or urgent readmission was higher in people from lower‐income neighborhoods. However, this risk appears to be explained by patient‐level factors that are known to be associated with bad postdischarge outcomes. After accounting for these factors with the LACE+ index, we found no notable changes in the risk of early death or urgent readmission with SES as measured with average neighborhood household income.
Nine previous studies have measured the association between various SES measures and hospital readmission in disparate populations.[3, 4, 5, 6, 7, 8, 9, 10, 11] These studies were done in the United States,[5, 6, 8, 9, 10] the United Kingdom,[3, 7] Australia,[4] and Canada.[11] They used a range of SES indicators (from area‐level measures of household income[5] or deprivation[3] to personal education and income)[8, 9, 10] in diverse patient populations (from a random sample of all hospitalizations[3] to people with disabilities living in New York City)[15] and very different time horizons (capturing hospital readmissions that occurred from within 30 days[5] to 4 years).[10] Of these 9 studies, 5 found no independent association between their SES measure and readmission,[5, 6, 8, 9, 10] and 2 included SES in their final regression model but did not present the modelmaking it impossible to determine if SES significantly influenced outcomes.[3, 15] One study found that the risk of hospital readmission independently increased as a composite measure of area‐level social and economic indicators decreased.[4] A Canadian study[11] measured neighborhood income quintile and showed, after adjusting for patient sex, comorbidities, LOS variance, and previous admissions, that the odds of acute, nonpsychiatric readmission within 30 days of discharge were approximately 10% higher in the lowest versus the highest SES quintile. The ability of this model to adjust for important confounders when associating SES and risk of readmission is uncertain because the model fit was not reported.
Several factors could explain the difference between our study and the previous Canadian analysis showing significantly higher adjusted risk of readmission in patients from the lowest versus the highest SES quintile.[11] First, our analysis had a slightly different outcome, combining early death with urgent readmission (rather than the latter alone). We believe that this combination is important to avoid biased results when associating patient factors with readmission risk.[14] Second, our unit of analysis was the patient, whereas in the previous analysis it was the hospitalization.[11] A recent analysis by our group found that this distinction can change the results on analyses in early postdischarge outcomes.[16] In the present analysis, different results could occur if patients with multiple readmissions were disproportionately prevalent in low‐income neighborhoods. Third, our analysis was limited to Ontario rather than the entire country. Finally, and we believe most importantly, we used a validated model to control for risk of poor outcomes soon after discharge from hospital. Our analysis shows that this risk was strongly associated with neighborhood income (Table 2). This suggests that the association between SES and bad postdischarge outcomes could be explained by factors that independently increase the risk of these outcomes. Adequately controlling for these covariates would then remove variation in readmission risk by SES. We believe that these results highlight the importance of adequately controlling for potential confounders.
We believe that our results are reassuring but not definitive. We found no indication that, in Ontario, people from poorer neighborhoods are systematically more likelyafter considering factors that are known to be associated with early death or urgent readmissionto have a worse outcome early after their discharge from hospital. However, patient income and other SES measures could be associated with early death or readmission for several reasons. First, our study used average neighborhood income quintiles to quantify SES. It is possible that other SES measures (such as education or social deprivation) or patient‐level SES indicators could be significantly associated with early death or readmission.[17, 18] Second, we previously found that approximately only 25% of hospital readmissions are potentially avoidable.[19] Further study is required to determine if patient SES independently influences potentially avoidable hospital readmissions. Third, we cannot be certain how our results might generalize to health populations outside of Ontario. Specifically, SES might play a more important role in regions without universal healthcare in which community‐based healthcare resources that could decrease readmission risk, such as medications or physician follow‐up, are unavailable to those without health insurance coverage. Finally, we found notable confounding between neighborhood income quintile and factors known to be independently associated with early death or urgent readmission (Figure 1). This was especially prominent with index admission urgency, number of previous urgent admissions and emergency visits, and patient comorbidities. These factors have a much stronger association with early death or readmission than neighborhood income quintile. If low neighborhood income actually results in urgent hospital admission, emergency‐department visits, and comorbidities, then the inclusion of these covariates in the model could obscure the influence of neighborhood income on early death or readmission.
In summary, our study found that neighborhood income was not associated with early death or urgent readmission independent of known risk factors. Our analysis indicates that focusing resources on patients in lower‐income neighborhoods is unlikely to change the risk of early postdischarge adverse events. Further study is required to determine if SES is associated with adverse postdischarge outcomes in settings without publicly funded healthcare.
Acknowledgment
Disclosure: Nothing to report.
- Last JM, ed. A Dictionary of Epidemiology. 3rd ed. New York, NY: Oxford University Press; 1995.
- Is income inequality a determinant of population health? Part 1: A systematic review. Milbank Q. 2004;82(1):5–99. , , , et al.
- Identifying patients at high risk of emergency hospital admissions: a logistic regression analysis. J R Soc Med. 2006;99(8):406–414. , , .
- Using routine inpatient data to identify patients at risk of hospital readmission. BMC Health Serv Res. 2009;9:96. , , , .
- Risk factors for 30‐day hospital readmission in patients ≥65 years of age. Proc (Bayl Univ Med Cent). 2008;21(4):363–372. , , , , .
- An automated model to identify heart failure patients at risk for 30‐day readmission or death using electronic medical record data. Med Care. 2010;48(11):981–988. , , , et al.
- Improving the management of care for high‐cost Medicaid patients. Health Aff (Millwood). 2007;26(6):1643–1654. , .
- Factors predicting readmission of older general medicine patients. J Gen Intern Med. 1991;6(5):389–393. , .
- Hospital readmission in general medicine patients: a prediction model. J Gen Intern Med. 2010;25(3):211–219. , , , et al.
- Screening elders for risk of hospital admission. J Am Geriatr Soc. 1993;41(8):811–817. , , , , , .
- Canadian Institute for Health Information. All‐Cause Readmission to Acute Care and Return to the Emergency Department. Ottawa, ON: Canadian Institute for Health Information; 2012:1–64.
- LACE+ index: extension of a validated index to predict early death or unplanned readmission following hospital discharge using administrative data. Open Medicine. 2012;6(2):80–89. , , .
- PCCF Plus version 5E user's guide. Ottawa ON: Statistics Canada; 2009;82F0086‐XDB. .
- Derivation and validation of diagnostic score based on case‐mix groups to predict 30‐day death or urgent readmission. Open Medicine. 2012;6(3):e80–e89. , , .
- Executing high‐quality care transitions: a call to do it right. J Hosp Med. 2007;2(5):287–290. , .
- Predicting post‐discharge death or readmission: deterioration of model performance in a population having multiple admissions per patient [published online ahead of print November 19, 2012]. J Eval Clin Pract. doi: 10.1111/jep.12012. , , , .
- Patient self‐management of chronic disease in primary care. JAMA. 2002;288(19): 2469–2475. , , , .
- Multilevel analyses of neighbourhood socioeconomic context and health outcomes: a critical review. J Epidemiol Community Health. 2001;55(2):111–122. , .
- Incidence of potentially avoidable hospital readmissions and its relationship to all‐cause urgent readmissions. CMAJ. 2011;183(14):E1067–E1072. , , , et al.
- Validation of a combined comorbidity index. J Clin Epidemiol. 1994;47(11):1245–1251. , , , .
- Last JM, ed. A Dictionary of Epidemiology. 3rd ed. New York, NY: Oxford University Press; 1995.
- Is income inequality a determinant of population health? Part 1: A systematic review. Milbank Q. 2004;82(1):5–99. , , , et al.
- Identifying patients at high risk of emergency hospital admissions: a logistic regression analysis. J R Soc Med. 2006;99(8):406–414. , , .
- Using routine inpatient data to identify patients at risk of hospital readmission. BMC Health Serv Res. 2009;9:96. , , , .
- Risk factors for 30‐day hospital readmission in patients ≥65 years of age. Proc (Bayl Univ Med Cent). 2008;21(4):363–372. , , , , .
- An automated model to identify heart failure patients at risk for 30‐day readmission or death using electronic medical record data. Med Care. 2010;48(11):981–988. , , , et al.
- Improving the management of care for high‐cost Medicaid patients. Health Aff (Millwood). 2007;26(6):1643–1654. , .
- Factors predicting readmission of older general medicine patients. J Gen Intern Med. 1991;6(5):389–393. , .
- Hospital readmission in general medicine patients: a prediction model. J Gen Intern Med. 2010;25(3):211–219. , , , et al.
- Screening elders for risk of hospital admission. J Am Geriatr Soc. 1993;41(8):811–817. , , , , , .
- Canadian Institute for Health Information. All‐Cause Readmission to Acute Care and Return to the Emergency Department. Ottawa, ON: Canadian Institute for Health Information; 2012:1–64.
- LACE+ index: extension of a validated index to predict early death or unplanned readmission following hospital discharge using administrative data. Open Medicine. 2012;6(2):80–89. , , .
- PCCF Plus version 5E user's guide. Ottawa ON: Statistics Canada; 2009;82F0086‐XDB. .
- Derivation and validation of diagnostic score based on case‐mix groups to predict 30‐day death or urgent readmission. Open Medicine. 2012;6(3):e80–e89. , , .
- Executing high‐quality care transitions: a call to do it right. J Hosp Med. 2007;2(5):287–290. , .
- Predicting post‐discharge death or readmission: deterioration of model performance in a population having multiple admissions per patient [published online ahead of print November 19, 2012]. J Eval Clin Pract. doi: 10.1111/jep.12012. , , , .
- Patient self‐management of chronic disease in primary care. JAMA. 2002;288(19): 2469–2475. , , , .
- Multilevel analyses of neighbourhood socioeconomic context and health outcomes: a critical review. J Epidemiol Community Health. 2001;55(2):111–122. , .
- Incidence of potentially avoidable hospital readmissions and its relationship to all‐cause urgent readmissions. CMAJ. 2011;183(14):E1067–E1072. , , , et al.
- Validation of a combined comorbidity index. J Clin Epidemiol. 1994;47(11):1245–1251. , , , .
Copyright © 2013 Society of Hospital Medicine